A Nonlinear Distortion Removal Based on Deep Neural Network for Underwater Acoustic OFDM Communication with the Mitigation of Peak to Average Power Ratio
APPLIED SCIENCES-BASEL(2020)
Abstract
Machine learning and deep learning algorithms have proved to be a powerful tool for developing data-driven signal processing algorithms for challenging engineering problems. This paper studies the modern machine learning algorithm for modeling nonlinear devices like power amplifiers (PAs) for underwater acoustic (UWA) orthogonal frequency divisional multiplexing (OFDM) communication. The OFDM system has a high peak to average power ratio (PAPR) in the time domain because the subcarriers are added coherently via inverse fast Fourier transform (IFFT). This causes a higher bit error rate (BER) and degrades the performance of the PAs; hence, it reduces the power efficiency. For long-range underwater acoustic applications such as the long-term monitoring of the sea, the PA works in full consumption mode. Thus, it becomes a challenging task to minimize power consumption and unnecessary distortion. To mitigate this problem, a receiver-based nonlinearity distortion mitigation method is proposed, assuming that the transmitting side has enough computation power. We propose a novel approach to identify the nonlinear power model using a modern deep learning algorithm named frequentative decision feedback (FFB); PAPR performance is verified by the clipping method. The simulation results prove the better performance of the PA model with a BER with the shortest learning time.
MoreTranslated text
Key words
underwater acoustic OFDM communication,machine learning,neural networks,peak to average power ratio,power amplifier,clipping
AI Read Science
Must-Reading Tree
Example
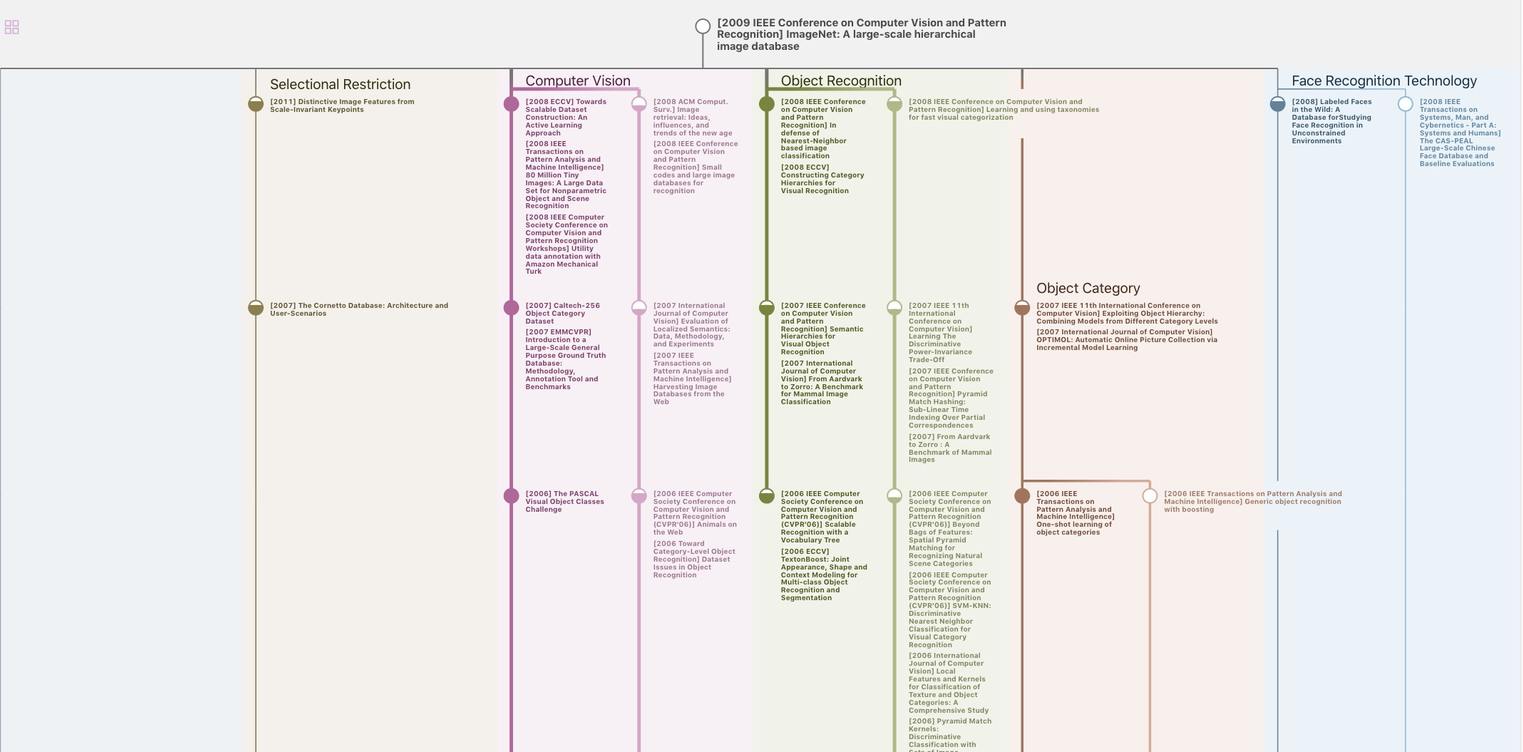
Generate MRT to find the research sequence of this paper
Chat Paper
Summary is being generated by the instructions you defined