A Multi-Class Objects Detection Coprocessor With Dual Feature Space and Weighted Softmax
IEEE Transactions on Circuits and Systems II: Express Briefs(2020)
摘要
A critical mission for mobile robot vision is to detect and classify different objects with low power consumption. In this brief, a multi-class object detection coprocessor is proposed by using the Histogram of Oriented Gradient (HOG) and Local Binary Pattern (LBP) together with a weighted Softmax classifier. Its architecture is compact and hardware-friendly suitable for energy-constrained applications. Firstly, the cell-based feature extraction unit and block-level normalization reuse the SRAMs for storing one-row cell and one-row block. Meanwhile, the working frequency of the feature extraction and block-normalization unit is synchronized to the image sensor for low dynamic power. Then, a block-level one-time sliding-detection-window (OTSDW) mechanism is developed for partial classification with scalable object size. Finally, the Softmax classifier, which is implemented by the look-up table, linear fitting methods, and the fixed-point number, is tested in the Fashion MNIST dataset to evaluate its performance in multi-class classification problems and it reached an accuracy of over 86.2% with 10,180 parameters. The experimental result shows that the hardware-resource usage of the FPGA implementation is capable of 60 fps VGA video with 80.98 mW power consumption. This method uses similar or even fewer hardware resources than that of previous work using only the HOG feature and single-class classifier.
更多查看译文
关键词
HOG,LBP,dual feature,softmax,hardware implementation
AI 理解论文
溯源树
样例
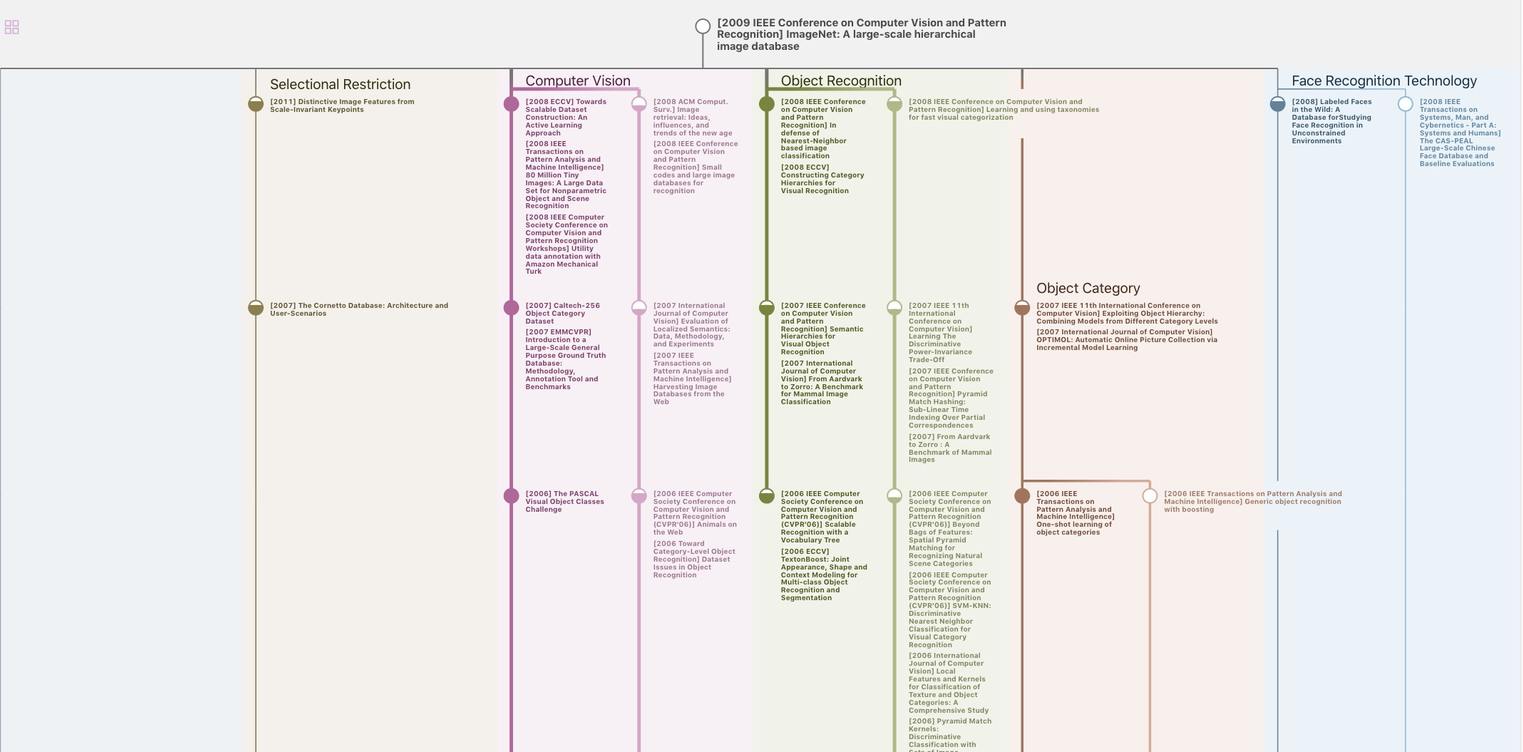
生成溯源树,研究论文发展脉络
Chat Paper
正在生成论文摘要