Image enhancement using deep-learning fully connected neural network mean filter
The Journal of Supercomputing(2020)
Abstract
Improving the quality of a noisy image is important for image applications. Many novel schemes pay great efforts in the removal of impulse noise. Most of them restore noisy pixels only by using the neighboring noise-free pixels, but the relationship between a noisy image and its noise-free one, which denotes the clean image not corrupted by noise, is ignored. So the reconstruction quality cannot be further improved. In this study, we employ a deep-learning fully connected neural network (FCNN) to select top N candidates of neighboring un-corrupted pixels for the restoration of a center noisy pixel in an analysis window. Hence, the mean value of the gray levels of these top N pixels is computed and employed to replace the noisy pixel, yielding the noisy pixel being restored. The experimental results reveal that the proposed deep-learning FCNN mean filter can remove impulse noise effectively in corrupted images with different noise densities.
MoreTranslated text
Key words
Image enhancement, Impulse noise, Mean filter, Deep learning, Neural networks
AI Read Science
Must-Reading Tree
Example
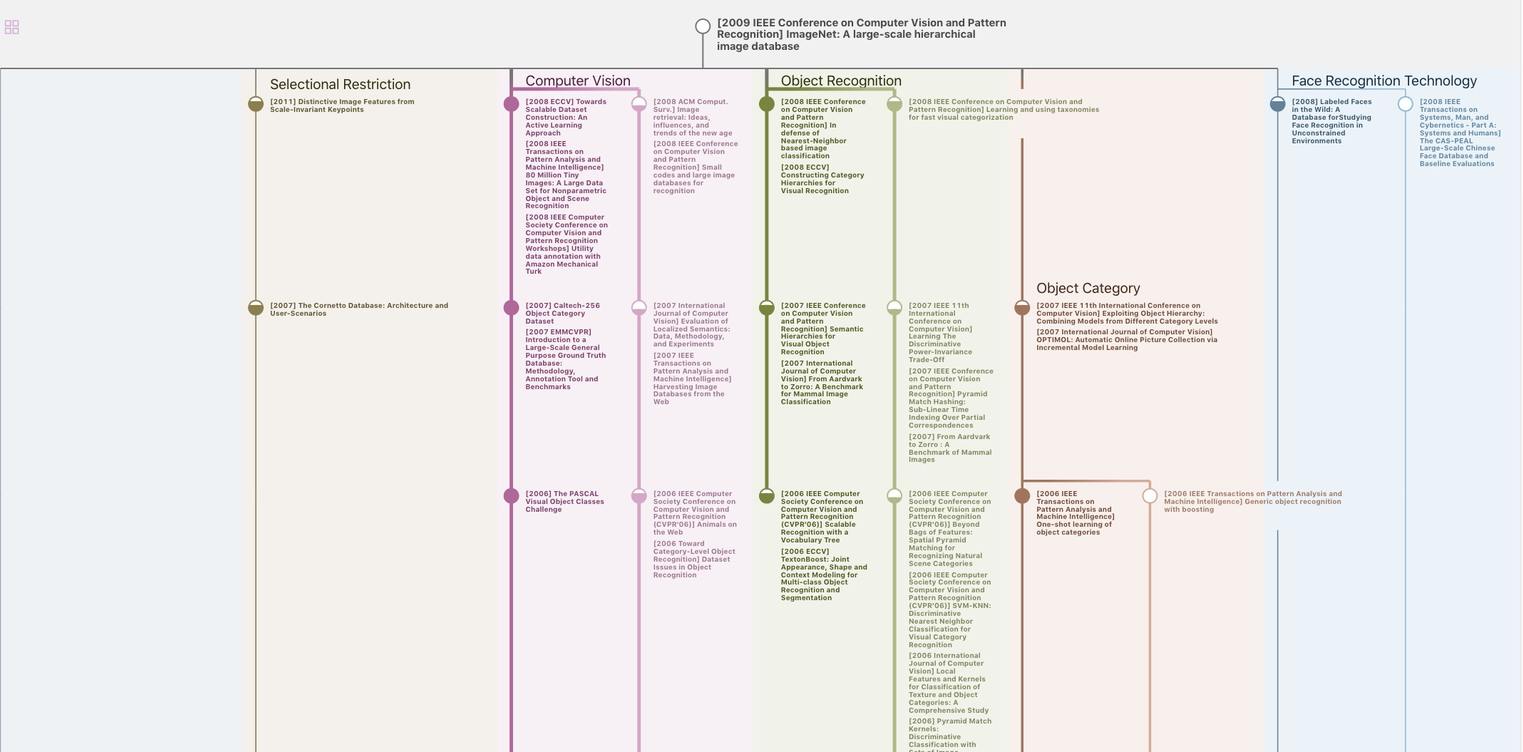
Generate MRT to find the research sequence of this paper
Chat Paper
Summary is being generated by the instructions you defined