mDixon-Based Synthetic CT Generation via Patch Learning.
ICMTEL (2)(2020)
摘要
We proposed a new method for generating synthetic CT on abdomen from modified Dixon (mDixon) MR data of abdomens to address the challenges of PET/MR attenuation correction (AC). AC is necessary in process of PET/MR but MR data lack photon attenuation, thus multiple methods are proposed to generate synthetic CT. However, these existing methods requires advantaged MR sequences which needs fine acquisition and huge cost consumption. To address this problem, we proposed a new method for generating synthetic CT using Patch Learning (SCG-PL). Global model of SCG-PL is transfer learning and patch model is semi-supervised classification. The advantages of our method can be summarized into two points. (1) Patch learning is a gradual learning process with gradually updating global model on remodeling patch model, so our SCG-PL method is gradually capable of generating synthetic CT. (2) Semi-supervised classification adopted in the process of patch learning, only small amount of labeled data is needed in SCG-PL, which greatly reduced the workload of radiologists. The experimental results indicate that proposed SCG-PL method can effectively generate synthetic CT image from challenging abdomen images using mDixon MR sequence data only.
更多查看译文
关键词
synthetic ct generation,patch,mdixon-based
AI 理解论文
溯源树
样例
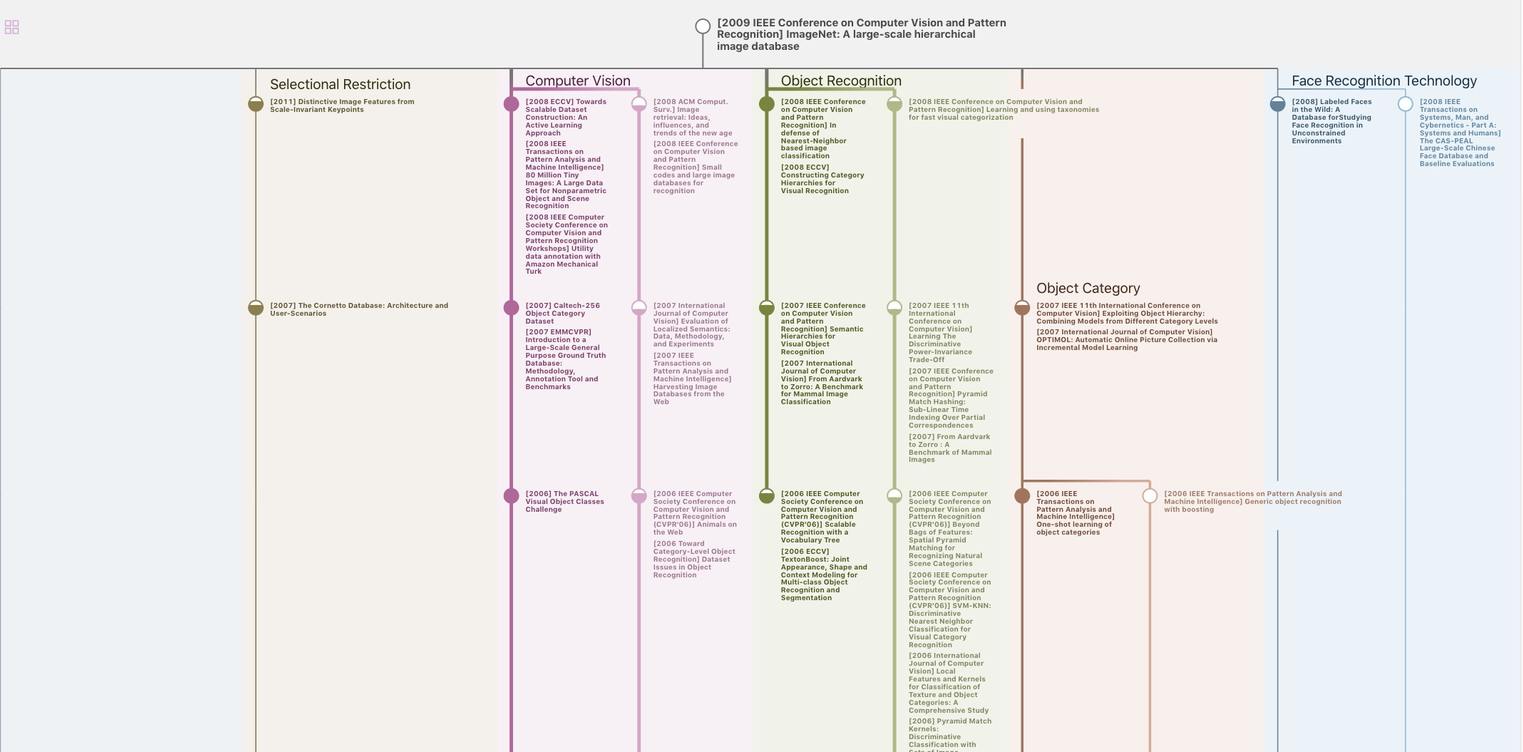
生成溯源树,研究论文发展脉络
Chat Paper
正在生成论文摘要