Exploiting Differential Flatness for Robust Learning-Based Tracking Control Using Gaussian Processes
IEEE Control Systems Letters(2021)
摘要
Learning-based control has shown to outperform conventional model-based techniques in the presence of model uncertainties and systematic disturbances. However, most state-of-the-art learning-based nonlinear trajectory tracking controllers still lack any formal guarantees. In this letter, we exploit the property of differential flatness to design an online, robust learning-based controller to achieve both high tracking performance and probabilistically guarantee a uniform ultimate bound on the tracking error. A common control approach for differentially flat systems is to try to linearize the system by using a feedback (FB) linearization controller designed based on a nominal system model. Performance and safety are limited by the mismatch between the nominal model and the actual system. Our proposed approach uses a nonparametric Gaussian Process (GP) to both improve FB linearization and quantify, probabilistically, the uncertainty in our FB linearization. We use this probabilistic bound in a robust linear quadratic regulator (LQR) framework. Through simulation, we highlight that our proposed approach significantly outperforms alternative learning-based strategies that use differential flatness.
更多查看译文
关键词
Feedback linearization,machine learning,robust control
AI 理解论文
溯源树
样例
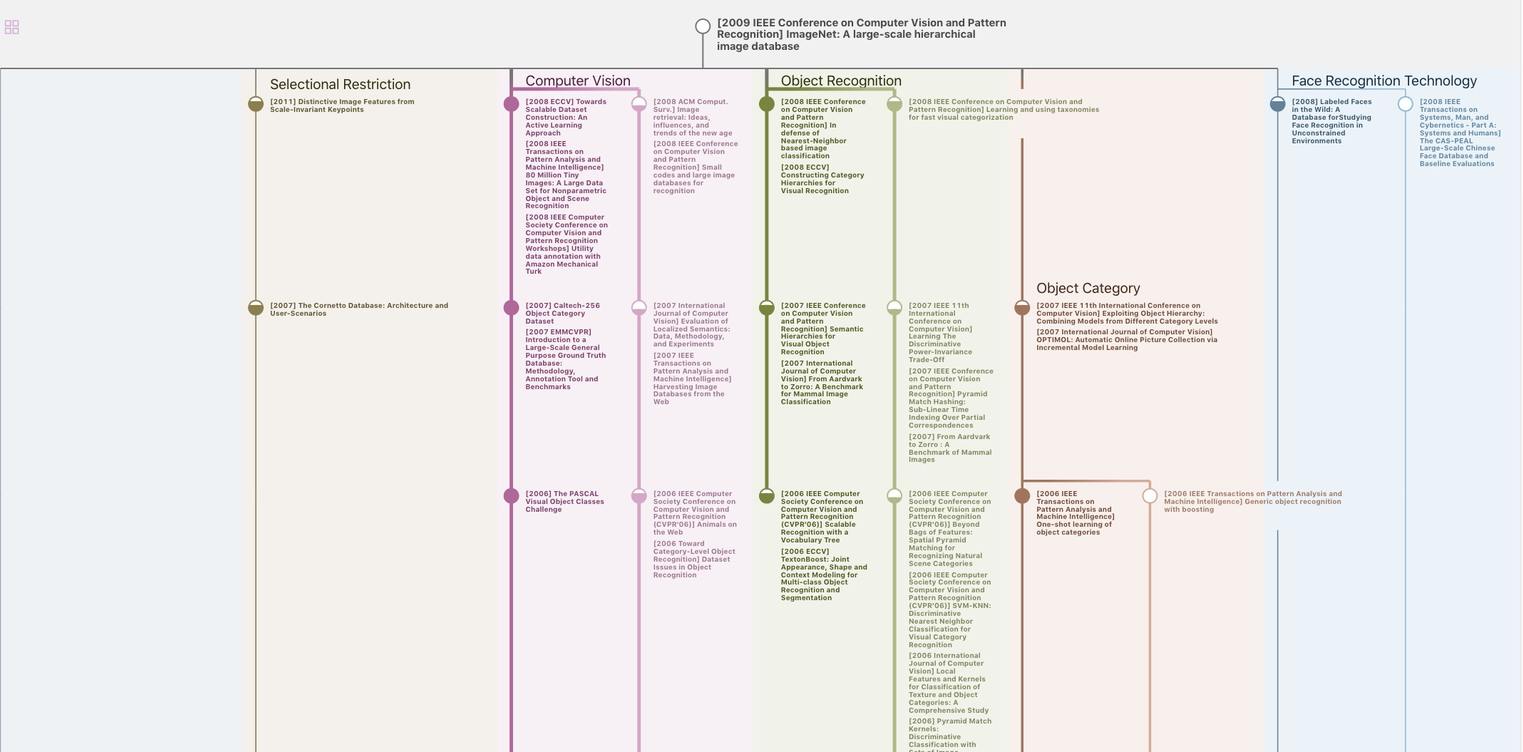
生成溯源树,研究论文发展脉络
Chat Paper
正在生成论文摘要