Joint Torque Estimation For The Human Arm From Semg Using Backpropagation Neural Networks And Autoencoders
BIOMEDICAL SIGNAL PROCESSING AND CONTROL(2020)
摘要
Estimation of joint torque for human arm is important to help a robot adapt to a human partner in human-robot collaboration. In this study, we proposed an estimating model combined the back propagation neural networks (BPNN) and autoencoders to estimate the joint torque of human arm from surface electromyography (sEMG). Autoencoders were used to select the appropriate features of sEMG signals. The joint angles obtained from a vision-system were combined with the sEMG features, which were set as the input data for the estimating model. A 4-layer BPNN was used to map the sEMG features to the joint torque. The proposed estimating model was compared to other five models through experiments by estimating shoulder joint torque and elbow joint torque. The root mean square error and correlation coefficient between the estimated joint torque value and a theoretic value calculated by inverse dynamics were used to evaluate the proposed model. The root mean square error and correlation coefficient for the proposed model is 1.3842 and 0.9439, respectively. Experimental results showed that the proposed model can derive lower root mean square error and higher correlation coefficient by comparing to other models, which indicates the proposed model can estimate more accurate joint torque from sEMG than other models. (C) 2020 Elsevier Ltd. All rights reserved.
更多查看译文
关键词
Joint torque estimation, Surface electromyography, Backpropagation neural networks, Autoencoder
AI 理解论文
溯源树
样例
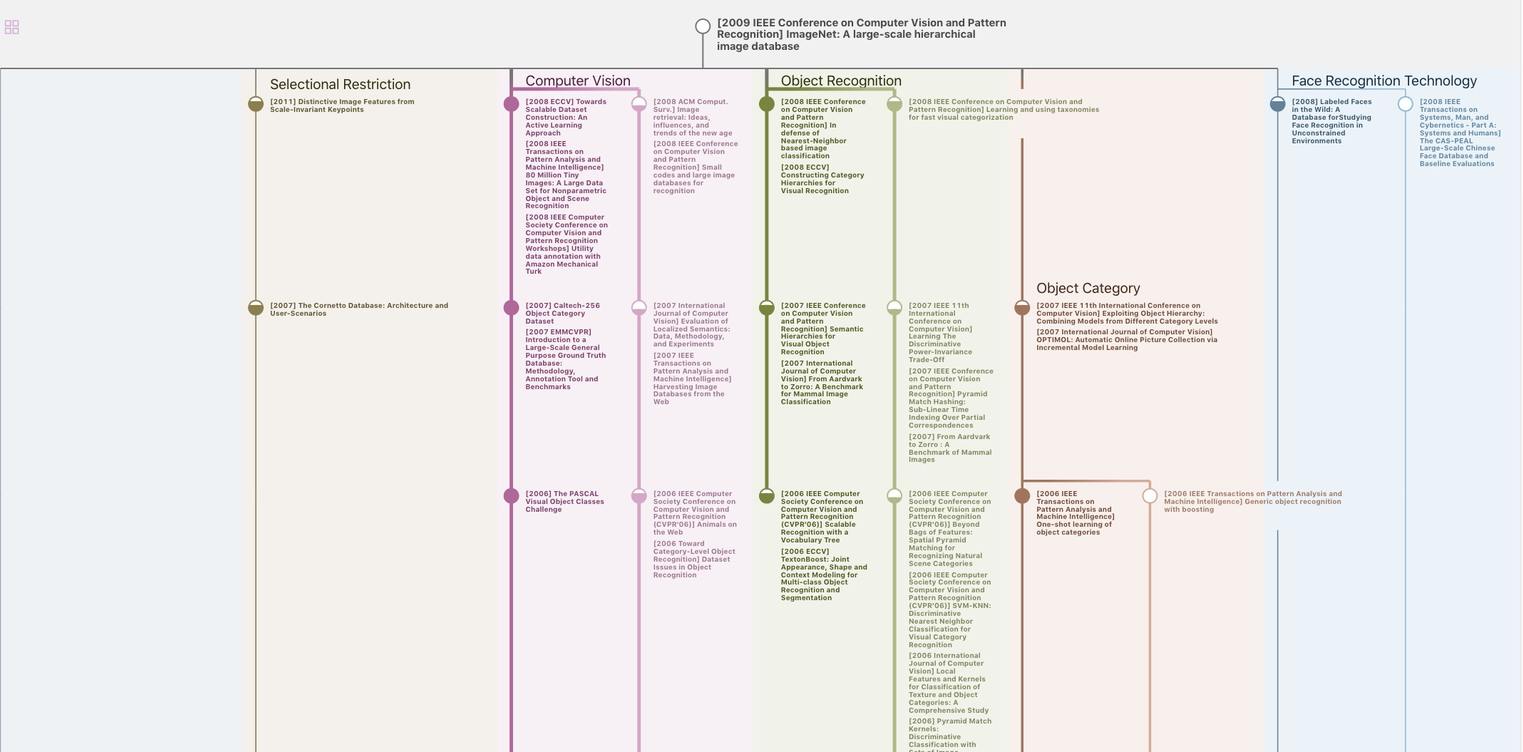
生成溯源树,研究论文发展脉络
Chat Paper
正在生成论文摘要