Handling non-linearity between classes using spectral and spatial information with kernel based modified possibilisticc-means classifier
GEOCARTO INTERNATIONAL(2022)
摘要
In this research work, non-linearity in data has been handled by incorporating kernel with the Modified Possibilistic c-Means (MPCM) algorithm. Nine different types of kernel function have been proposed to classify nine different classes and have non-linearity among them. Gaussian has been identified as the best performing kernel at an optimized fuzzified value m = 1.5 with an overall accuracy 92.45%. The composite kernels have been generated with an aim of improvement of accuracy in comparison to single kernel. Further, role of spatial constraints has been analyzed by adding neighboring pixel information to handle the noise. It was observed that overall accuracy depends on the spatial parameter that has been included. Thus, local information having local similarity measure parameters (S-ir)in the image produces highest accuracy compared to others. Moreover, the identified best kernel (Gaussian Kernel) has been then used for extraction of the burnt paddy fields in a different test site.
更多查看译文
关键词
Modified possibilistic c-mean (MPCM), kernels, composite kernel, spatial information
AI 理解论文
溯源树
样例
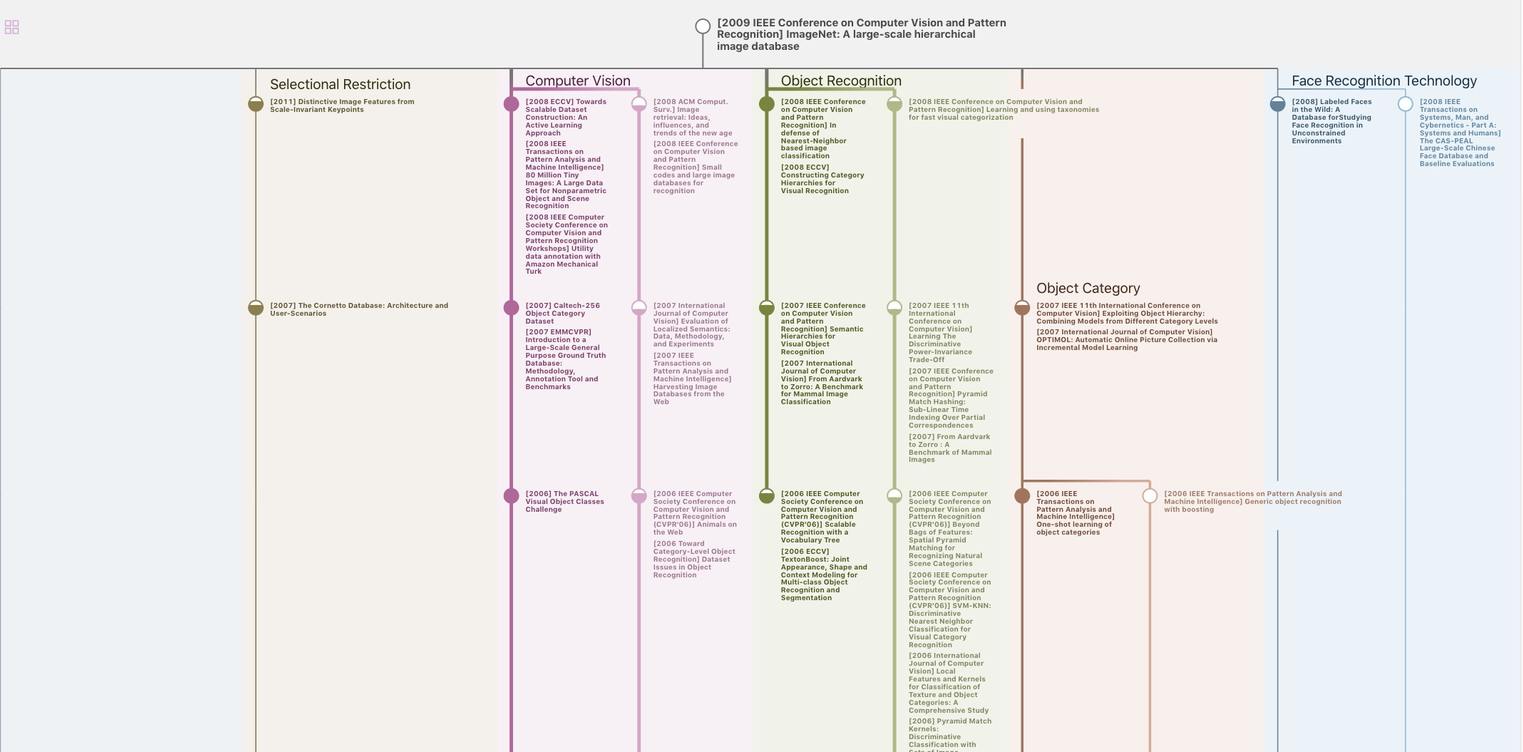
生成溯源树,研究论文发展脉络
Chat Paper
正在生成论文摘要