A comparison of particle swarm optimization and genetic algorithm for daily rainfall-runoff modelling: a case study for Southeast Queensland, Australia
OPTIMIZATION AND ENGINEERING(2020)
Abstract
Real-time and short-term prediction of river flow is essential for efficient flood management. To obtain accurate flow predictions, a reliable rainfall-runoff model must be used. This study proposes the application of two evolutionary algorithms, particle swarm optimization (PSO) and genetic algorithm (GA), to train the artificial neural network (ANN) parameters in order to overcome the ANN drawbacks, such as slow learning speed and frequent trapping at local optimum. These hybrid ANN-PSO and ANN-GA approaches were validated to equip natural hazard decision makers with a robust tool for forecasting real-time streamflow as a function of combinations of different lagged rainfall and streamflow in a small catchment in Southeast Queensland, Australia. Different input combinations of lagged rainfall and streamflow (delays of one, two and three days) were tested to investigate the sensitivity of the model to the number of delayed days, and to identify the effective model input combinations for the accurate prediction of real-time streamflow, which has not yet been recognized in other studies. The results indicated that the ANN-PSO model significantly outperformed the ANN-GA model in terms of convergence speed, accuracy, and fitness function evaluation. Additionally, it was found that the rainfall and streamflow with 3-day lag time had less impact on the predicted streamflow of the studied basin, confirming that the flow of the studied river is significantly correlated with only 2-day lagged rainfall and streamflow.
MoreTranslated text
Key words
Real-time streamflow,Evolutionary computation,ANN,PSO,GA,Rainfall-runoff modelling
AI Read Science
Must-Reading Tree
Example
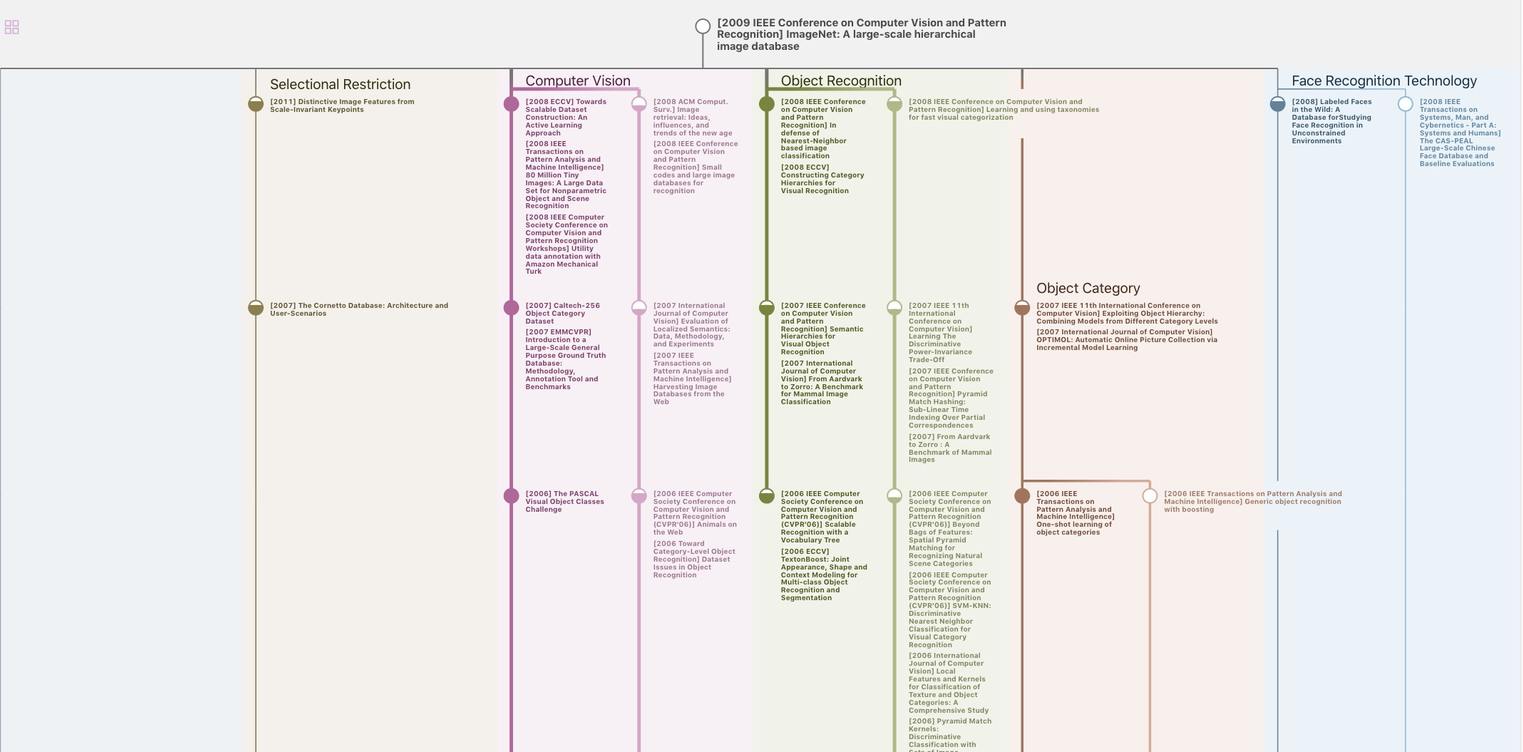
Generate MRT to find the research sequence of this paper
Chat Paper
Summary is being generated by the instructions you defined