Improving Object Detection Performance Using Scene Contextual Constraints
IEEE Transactions on Cognitive and Developmental Systems(2022)
Abstract
Contextual information, such as the co-occurrence of objects and the spatial and relative size among objects, provides rich and complex information about digital scenes. It also plays an important role in improving object detection and determining out-of-context objects. In this work, we present contextual models that leverage contextual information (16 contextual relationships are applied in this article) to enhance the performance of two of the state-of-the-art object detectors (i.e., faster RCNN and you look only once), which are applied as a postprocessing process for most of the existing detectors, especially for refining the confidences and associated categorical labels, without refining bounding boxes. We experimentally demonstrate that our models lead to enhancement in detection performance using the most common data set used in this field (MSCOCO), where in some experiments, PASCAL2012 is also used. We also show that iterating the process of applying our contextual models also enhances the detection performance further.
MoreTranslated text
Key words
Contextual information,neural network,object detection,out-of-context,relabeling,rescoring,scale,semantic,spatial
AI Read Science
Must-Reading Tree
Example
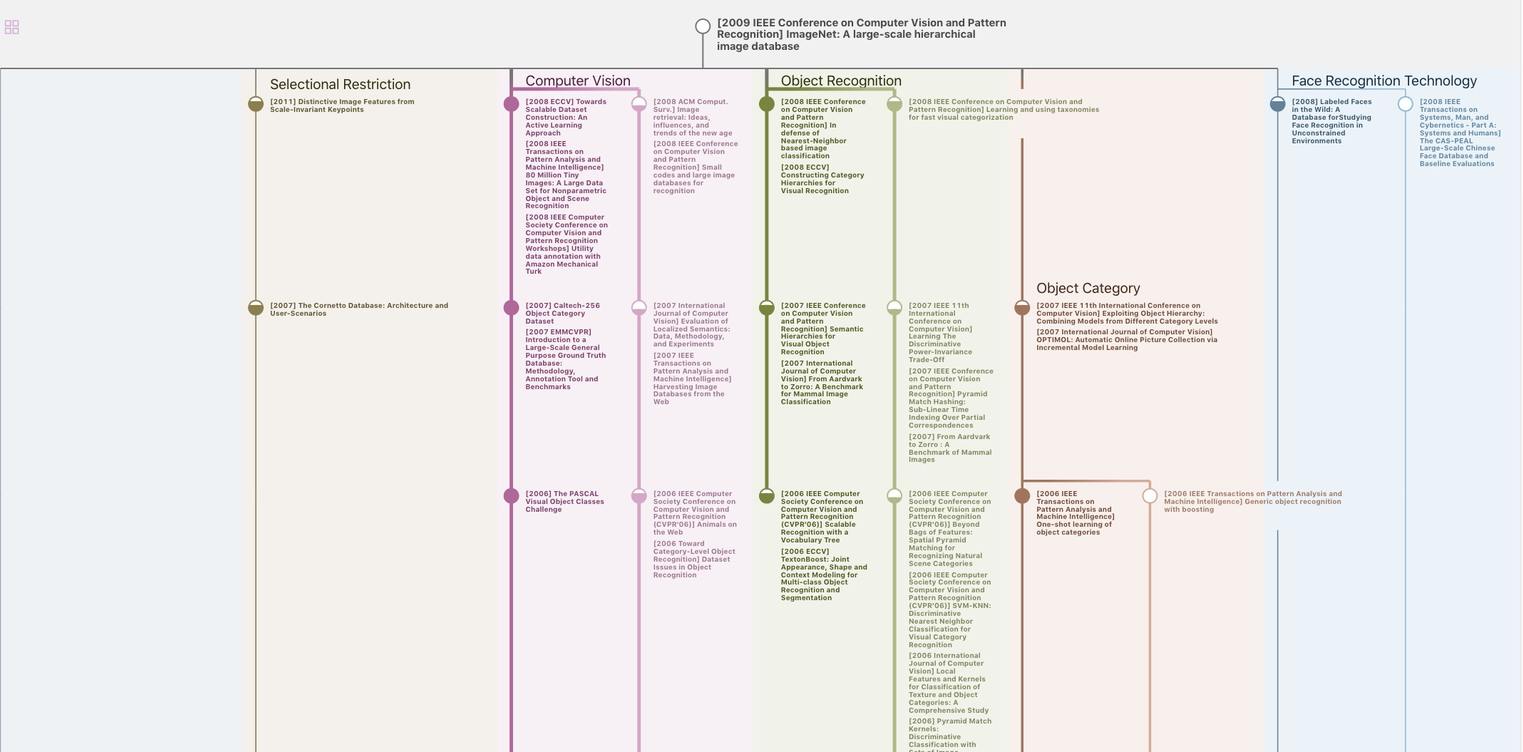
Generate MRT to find the research sequence of this paper
Chat Paper
Summary is being generated by the instructions you defined