Incremental Clustering With Hard Centers
IEEE MultiMedia(2020)
摘要
FU-PCM is an effective clustering mode by inducing the regularization constraint in C-means to avoid the interference of noises and outliers. However, it is difficult to obtain the satisfactory performance in the context of imbalance category distribution. To address above problem, first, we propose a novel clustering model, which exploits Pearson Correlation Coefficient to auto-balance the optimal equation according to the category distribution. Then, we extend it to the incremental model, which only learns samples at the current frame to update the original model by mapping centers of two adjacent frames to the distinguishable space, and mining hard centers to recognize new categories in online datasets. In this article, offline and online methods are verified in popular datasets, and experimental results demonstrate the effectiveness and efficiency of the proposed models.
更多查看译文
关键词
mining hard centers,online datasets,incremental clustering,FU-PCM,regularization constraint,imbalance category distribution,clustering model,Pearson correlation coefficient,optimal equation,incremental model,mapping centers
AI 理解论文
溯源树
样例
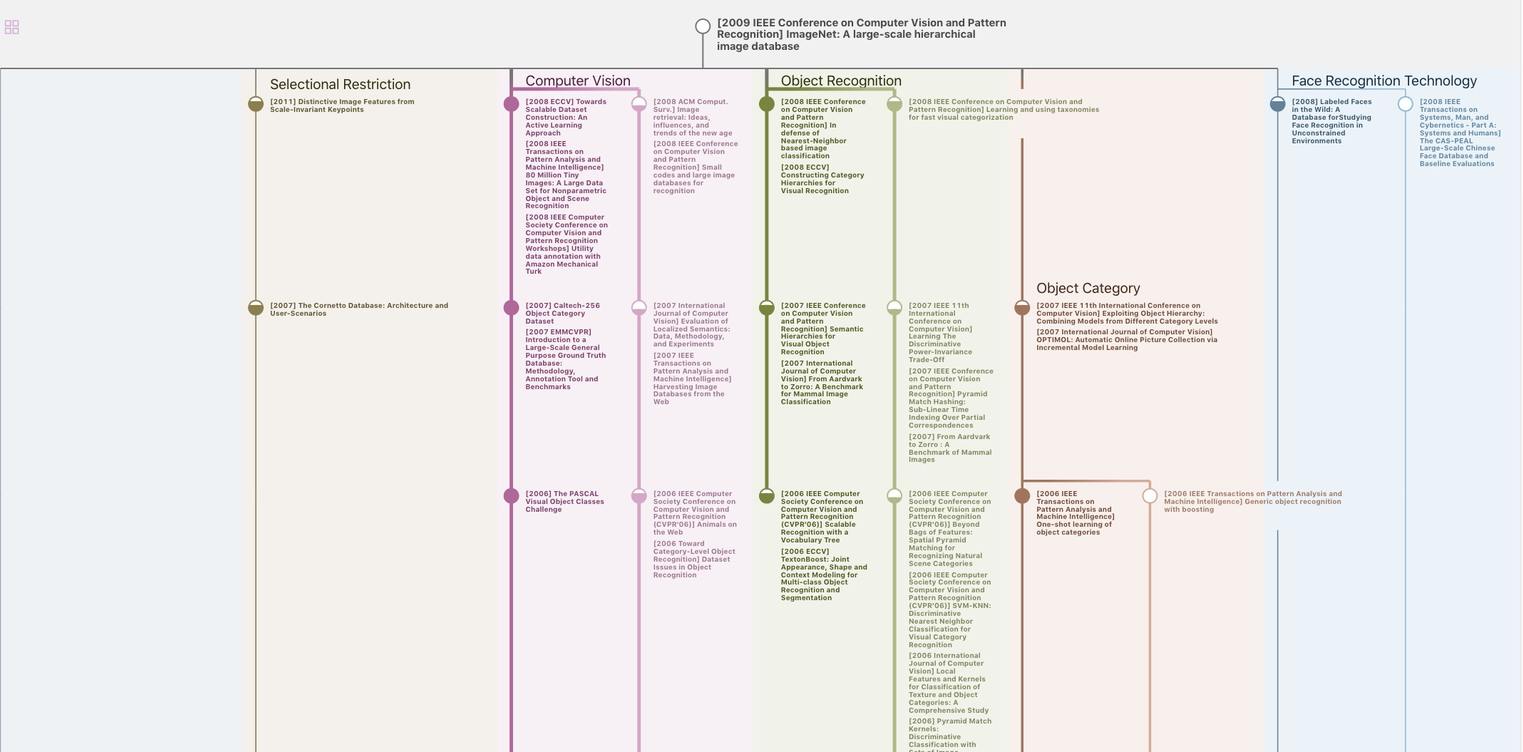
生成溯源树,研究论文发展脉络
Chat Paper
正在生成论文摘要