Distance transform data augmentation and stochastic patch-wise image prediction methodology for small dataset learning
Neurocomputing(2020)
AI Read Science
Must-Reading Tree
Example
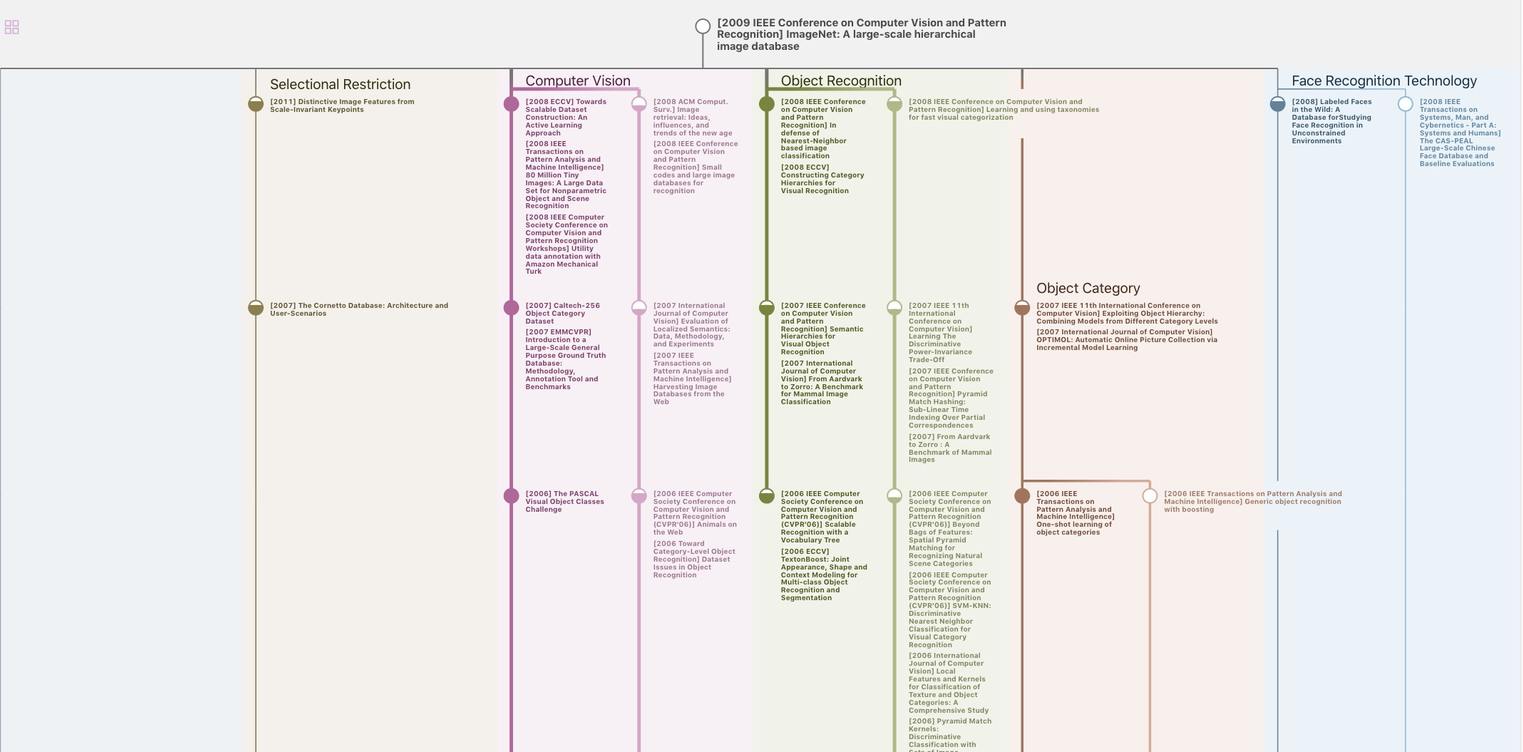
Generate MRT to find the research sequence of this paper
Chat Paper
Summary is being generated by the instructions you defined