A convolutional autoencoder-based approach with batch normalization for energy disaggregation
The Journal of Supercomputing(2020)
摘要
Non-intrusive loading monitoring (NILM) is a load analyzing algorithm that performs the energy dis-aggregation of power load for the smart meter technology. NILM is a highly valuable application due to its cost effectiveness, but it is a very challenging research because the noisy low-level features are not easily distinguishable when multiple appliances are used together. This paper proposes a deep learning-based scheme, named the CAEBN-HC, to address this issue. The proposed CAEBN-HC is designed based on the one-dimensional convolutional neural networks (1D-CNN) autoencoder and uses advanced training techniques, particularly the batch normalization (BN) and hill climbing (HC) algorithm to solve the NILM problem. The 1D-CNN autoencoder is used to extract the temporal features, and the BN is used to re-adjust the output distribution of each layer to prevent the gradient vanishing or explosion problem in the training process. In addition, the HC is used to perform the hyperparameter tuning. The NILM problem is first modeled as a regression problem, and the proposed method can predict the target signal correctly. To validate the effectiveness of the proposed scheme, the REDD appliance and power usage dataset is applied as a benchmark for performance comparison. Results showed that the proposed CAEBN-HC performed the best when compared with the LSTM and the conventional convolutional autoencoder (CAE) scheme without batch normalization and hyperparameter optimization.
更多查看译文
关键词
NILM, Autoencoder, Deep learning, CNN, Energy disaggregation, Batch normalization, Hill climbing algorithm
AI 理解论文
溯源树
样例
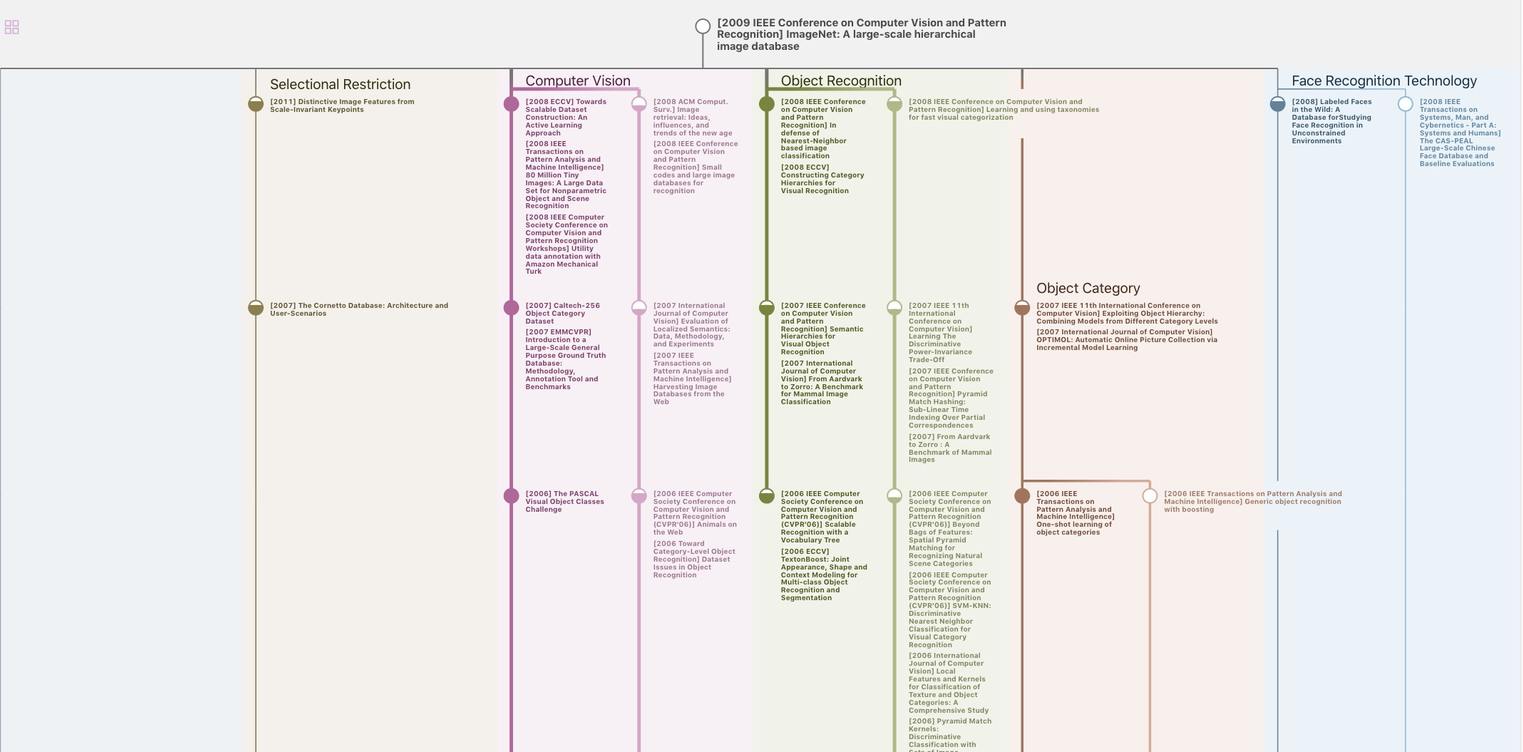
生成溯源树,研究论文发展脉络
Chat Paper
正在生成论文摘要