Efficient nearest neighbors methods for support vector machines in high dimensional feature spaces
OPTIMIZATION LETTERS(2020)
摘要
In the context of support vector machines, identifying the support vectors is a key issue when dealing with large data sets. In Camelo et al. (Ann Oper Res 235:85–101, 2015), the authors present a promising approach to finding or approximating most of the support vectors through a procedure based on sub-sampling and enriching the support vector sets by nearest neighbors. This method has been shown to improve the computational efficiency of support vector machines on large data sets with low or intermediate feature space dimension. In the present article we discuss ways of adapting the nearest neighbor enriching methodology to the context of very high dimensional data, such as text data or other high dimensional data types, for which nearest neighbor queries involve, in principle, a high computational cost. Our approach incorporates the proximity preserving order search algorithm of Chavez et al. (MICAI 2005: advances in artificial intelligence, Springer, Berlin, pp 405–414, 2005), into the nearest neighbor enriching method of Camelo et al. (2015), in order to adapt this procedure to the high dimension setting. For the required set of pivots, both random pivots and the base prototype pivot set of Micó et al. (Pattern Recogn Lett 15:9–17, 2015), are considered. The methodology proposed is evaluated on real data sets.
更多查看译文
关键词
Nearest neighbors methods, Support vector machines, High dimensional features, Pivots
AI 理解论文
溯源树
样例
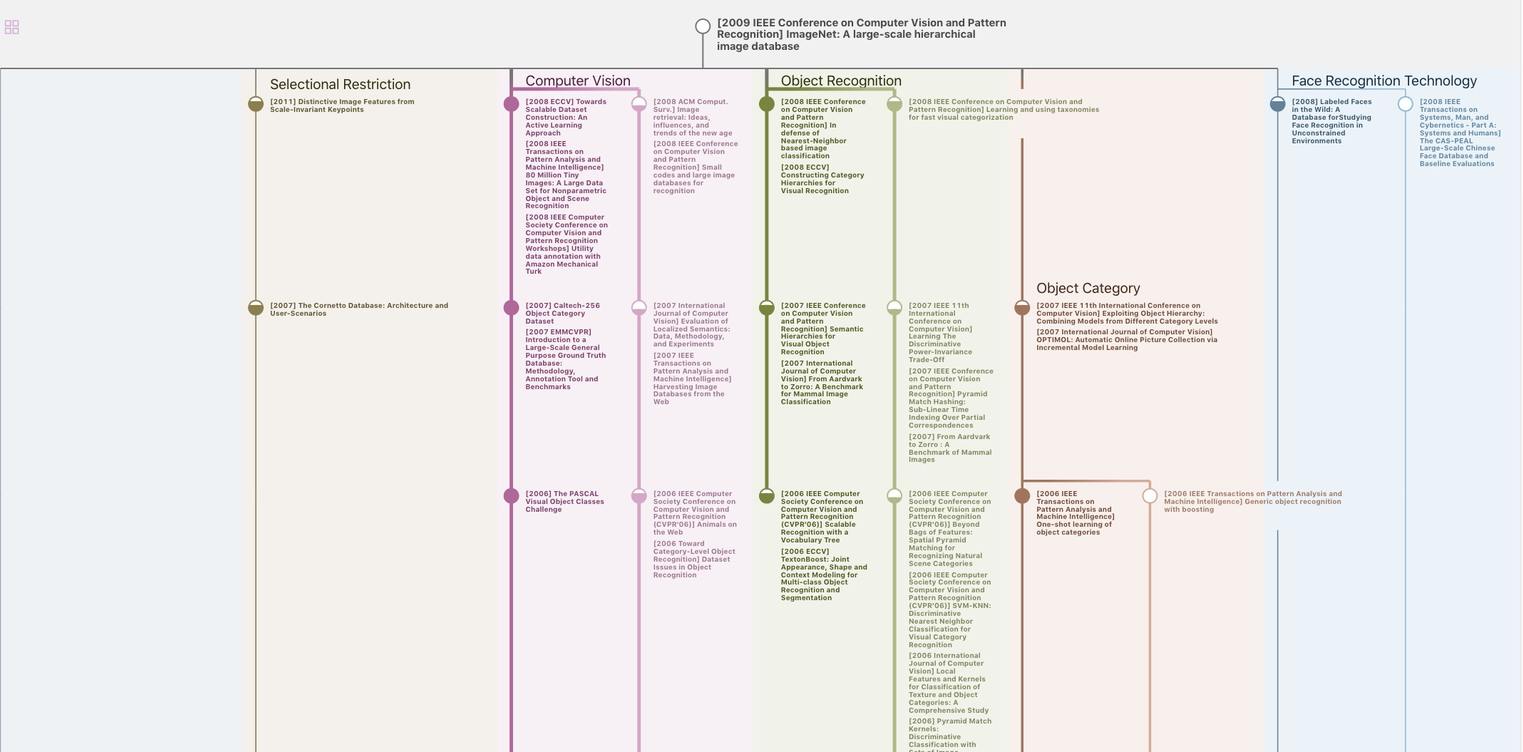
生成溯源树,研究论文发展脉络
Chat Paper
正在生成论文摘要