Predicting Protracted Concussion Recovery To Inform Proactive Care: A Genetic Fuzzy Machine Learning Approach
Medicine and Science in Sports and Exercise(2020)
Abstract
Poor prognostic accuracy of sport-related concussion (SRC) recovery times has limited proactive clinical care. Currently, clinicians consolidate a battery of assessments and combine their own practical knowledge to develop prognosis and treatment plans. Machine learning may provide a useful method to augment clinicians’ prognostic decision making: a critical first step to enhance proactive care. PURPOSE: Determine utility of a novel genetic fuzzy system (GFS) machine learning approach, FuzzyBolt, to predict protracted recovery after SRC. METHOD: Data from 76 pediatric patients (age 14.44 ± 2.54 years; 28 F) were obtained from 186 combined clinic visits following initial SRC. Recovery time was indexed from the physician-recorded full clearance to return to play date in the medical record and then classified as less than or equal to 28 days (N=88) vs. greater than 28 days (N=98)—the consensus pediatric threshold for persistent symptoms. A GFS model classified protracted recovery on patients that were less than 28 days in recovery. GFS uses fuzzification, rule-inference and defuzzification to make decisions, and FuzzyBolt provides an efficient method of optimizing model parameters via genetic algorithms. The model used 36 inputs, including ordinal and binary variables related to patient demographics, standardized Post-Concussion Symptom Inventory responses, and self-reported responses from a clinic-based Head Injury Questionnaire. RESULTS: Data were split, via stratified sampling, into a training set (80%; 61 athletes with 151 visits) and a hold-out validation set (20%; 15 athletes with 35 visits). Each patient visit was considered a unique case, with visits from the same patient never part of both the training and validation sets to reduce the risk of over-fitting and inflation of non-generalizable prediction accuracy. The FuzzyBolt model correctly predicted 12 of 16 protracted (and 16 of 19 typical) recovery cases, for an overall classification accuracy of 80%. CONCLUSION: This is the highest prediction accuracy, to date, for any published prognostic model of concussion recovery. It is a first step toward promoting early allocation of resources for patients at high-risk for protracted recovery, and demonstrates a novel technique to empower a data-driven solution to improve outcomes in these athletes.
MoreTranslated text
Key words
concussion recovery,proactive care,machine learning,machine learning approach
AI Read Science
Must-Reading Tree
Example
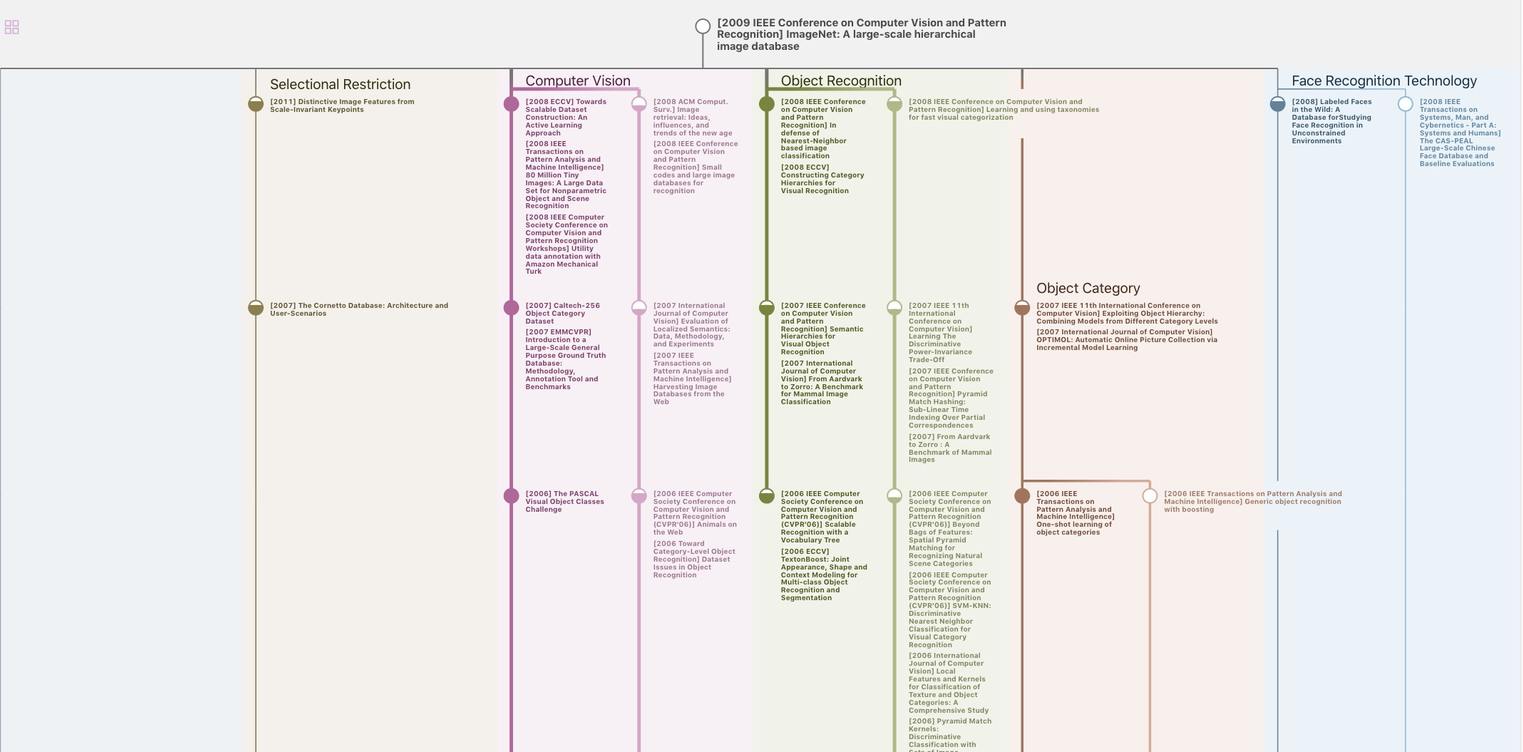
Generate MRT to find the research sequence of this paper
Chat Paper
Summary is being generated by the instructions you defined