MultiDefectNet: Multi-Class Defect Detection of Building Facade Based on Deep Convolutional Neural Network
SUSTAINABILITY(2020)
摘要
Defects in residential building facades affect the structural integrity of buildings and degrade external appearances. Defects in a building facade are typically managed using manpower during maintenance. This approach is time-consuming, yields subjective results, and can lead to accidents or casualties. To address this, we propose a building facade monitoring system that utilizes an object detection method based on deep learning to efficiently manage defects by minimizing the involvement of manpower. The dataset used for training a deep-learning-based network contains actual residential building facade images. Various building designs in these raw images make it difficult to detect defects because of their various types and complex backgrounds. We employed the faster regions with convolutional neural network (Faster R-CNN) structure for more accurate defect detection in such environments, achieving an average precision (intersection over union (IoU) = 0.5) of 62.7% for all types of trained defects. As it is difficult to detect defects in a training environment, it is necessary to improve the performance of the network. However, the object detection network employed in this study yields an excellent performance in complex real-world images, indicating the possibility of developing a system that would detect defects in more types of building facades.
更多查看译文
关键词
multi-class defect detection,building faç,ade defect,deep learning,Faster R-CNN
AI 理解论文
溯源树
样例
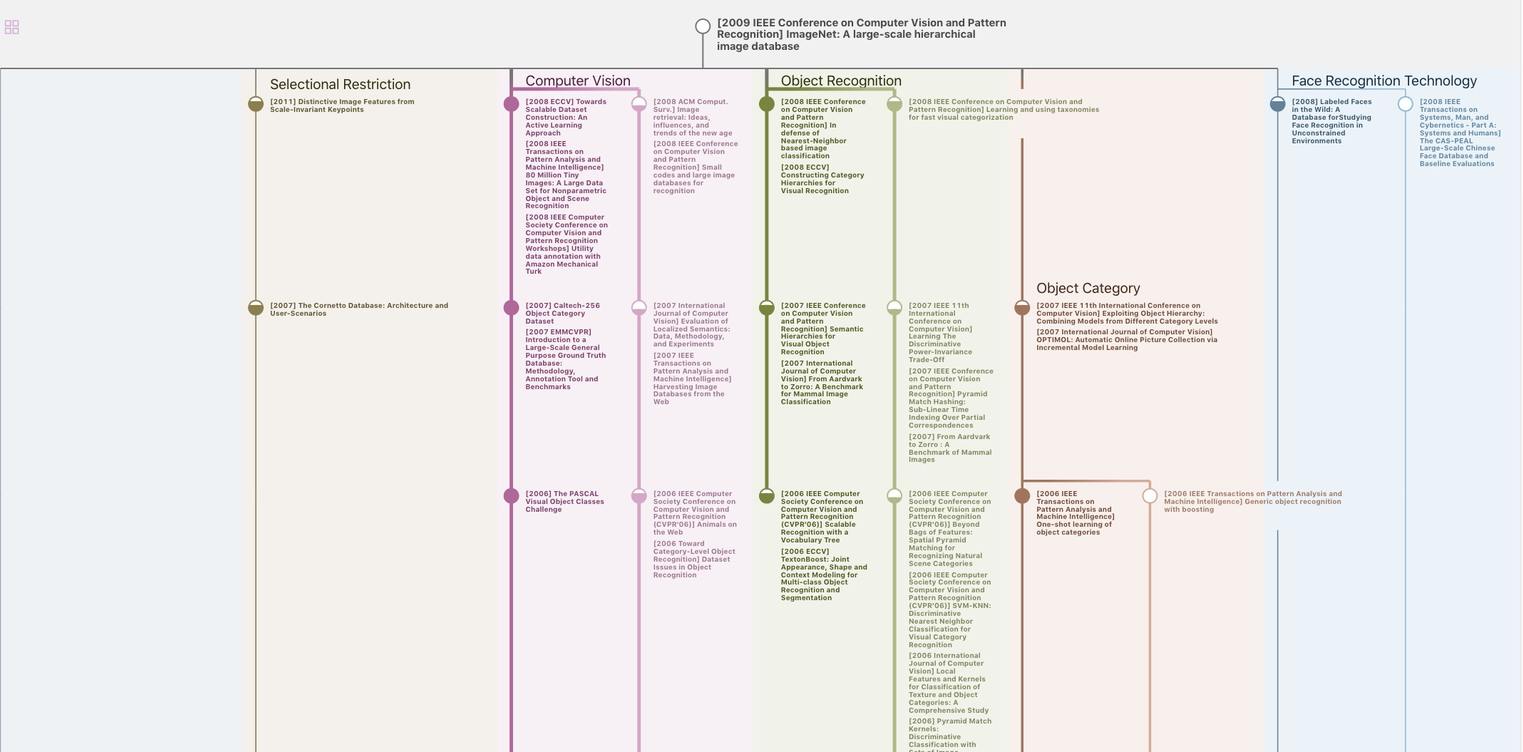
生成溯源树,研究论文发展脉络
Chat Paper
正在生成论文摘要