Assessing the validity domains of graphical Gaussian models in order
STATISTICAL APPLICATIONS IN GENETICS AND MOLECULAR BIOLOGY(2008)
摘要
The study of the interactions of cellular components is an essential base step to understand the structure and dynamics of biological networks. Various methods were recently developed for this purpose. While most of them combine different types of data and a priori knowledge, methods based on graphical Gaussian models are capable of learning the network directly from raw data. They consider the full-order partial correlations which are partial correlations between two variables given the remaining ones, for modeling direct links between variables. Statistical methods were developed for estimating these links when the number of observations is larger than the number of variables. However, the rapid advance of new technologies that allow the simultaneous measure of genome expression, led to large-scale datasets where the number of variables is far larger than the number of observations. To get around this dimensionality problem, different strategies and new statistical methods were proposed. In this study we focused on statistical methods recently published. All are based on the fact that the number of direct relationships between two variables is very small in regards to the number of possible relationships, p(p-1)/2. In the biological context, this assumption is not always satisfied over the whole graph. It is essential to precisely know the behavior of the methods in regards to the characteristics of the studied object before applying them. For this purpose, we evaluated the validity domain of each method from wide-ranging simulated datasets. We then illustrated our results using recently published biological data.
更多查看译文
关键词
graphical Gaussian models,biological networks
AI 理解论文
溯源树
样例
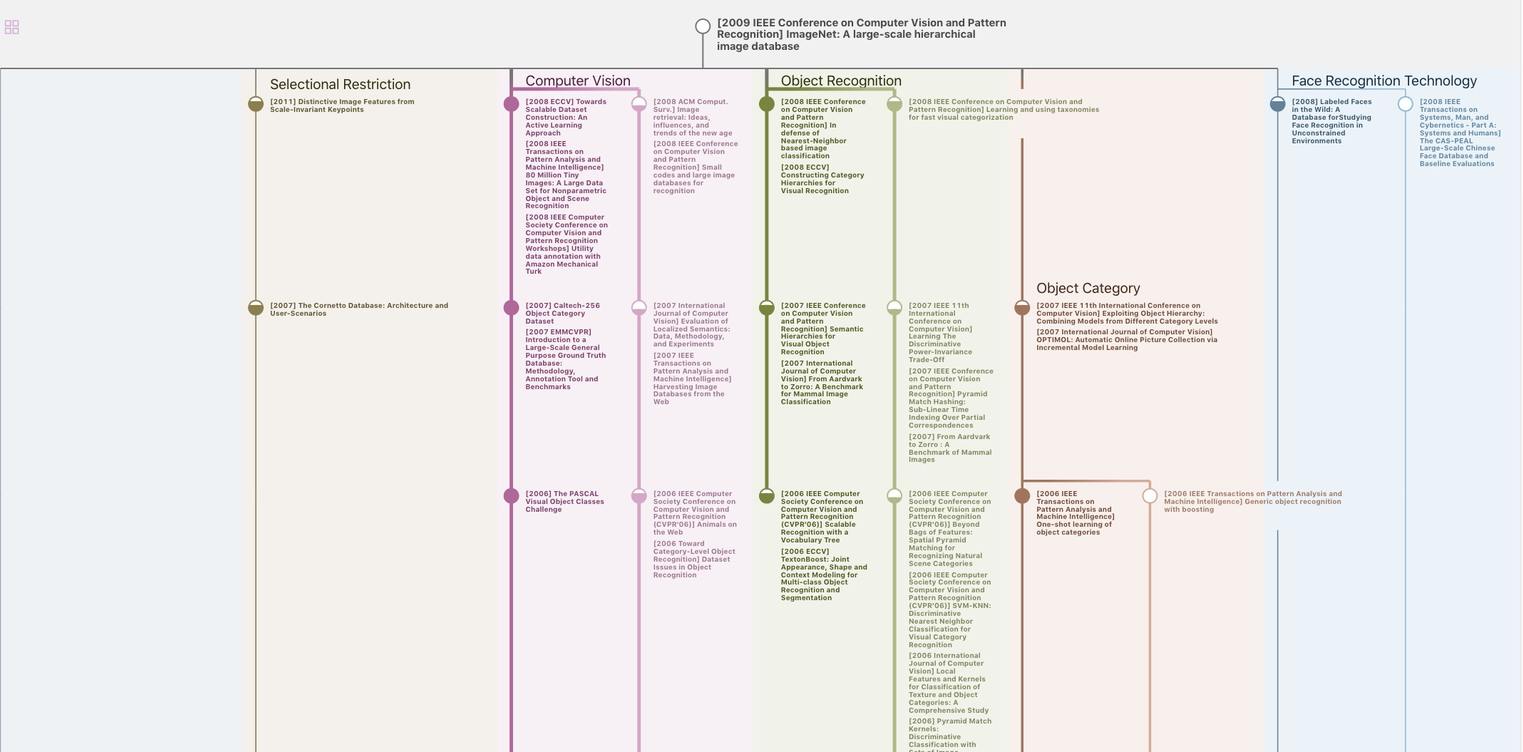
生成溯源树,研究论文发展脉络
Chat Paper
正在生成论文摘要