Combining cox regressions across a heterogeneous distributed research network facing small and zero counts
STATISTICAL METHODS IN MEDICAL RESEARCH(2022)
摘要
Studies of the effects of medical interventions increasingly take place in distributed research settings using data from multiple clinical data sources including electronic health records and administrative claims. In such settings, privacy concerns typically prohibit sharing of individual patient data, and instead, cross-network analyses can only utilize summary statistics from the individual databases such as hazard ratios and standard errors. In the specific but very common context of the Cox proportional hazards model, we show that combining such per site summary statistics into a single network-wide estimate using standard meta-analysis methods leads to substantial bias when outcome counts are small. This bias derives primarily from the normal approximations of the per site likelihood that the methods utilized. Here we propose and evaluate methods that eschew normal approximations in favor of three more flexible approximations: a skew-normal, a one-dimensional grid, and a custom parametric function that mimics the behavior of the Cox likelihood function. In extensive simulation studies, we demonstrate how these approximations impact bias in the context of both fixed-effects and (Bayesian) random-effects models. We then apply these approaches to three real-world studies of the comparative safety of antidepressants, each using data from four observational health care databases.
更多查看译文
关键词
proportional hazards, meta-analysis, privacy preservation, Bayesian, distributed research networks
AI 理解论文
溯源树
样例
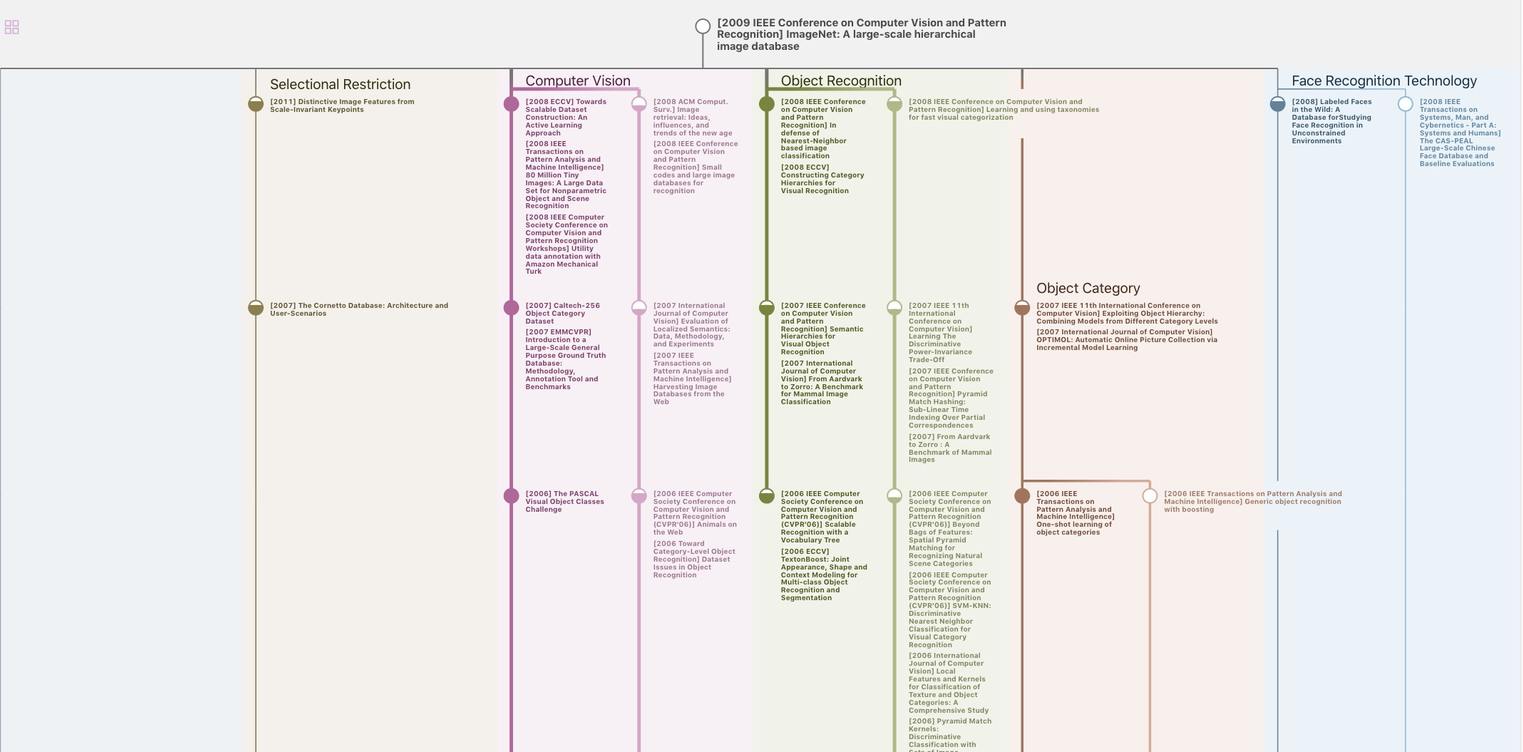
生成溯源树,研究论文发展脉络
Chat Paper
正在生成论文摘要