The Bayesian Inference Of Pareto Models Based On Information Geometry
ENTROPY(2021)
摘要
Bayesian methods have been rapidly developed due to the important role of explicable causality in practical problems. We develope geometric approaches to Bayesian inference of Pareto models, and give an application to the analysis of sea clutter. For Pareto two-parameter model, we show the non-existence of alpha-parallel prior in general, hence we adopt Jeffreys prior to deal with the Bayesian inference. Considering geodesic distance as the loss function, an estimation in the sense of minimal mean geodesic distance is obtained. Meanwhile, by involving Al-Bayyati's loss function we gain a new class of Bayesian estimations. In the simulation, for sea clutter, we adopt Pareto model to acquire various types of parameter estimations and the posterior prediction results. Simulation results show the advantages of the Bayesian estimations proposed and the posterior prediction.
更多查看译文
关键词
Bayesian inference, Pareto two-parameter model, Jeffreys prior, mean geodesic estimation, Al-Bayyati’, s loss function
AI 理解论文
溯源树
样例
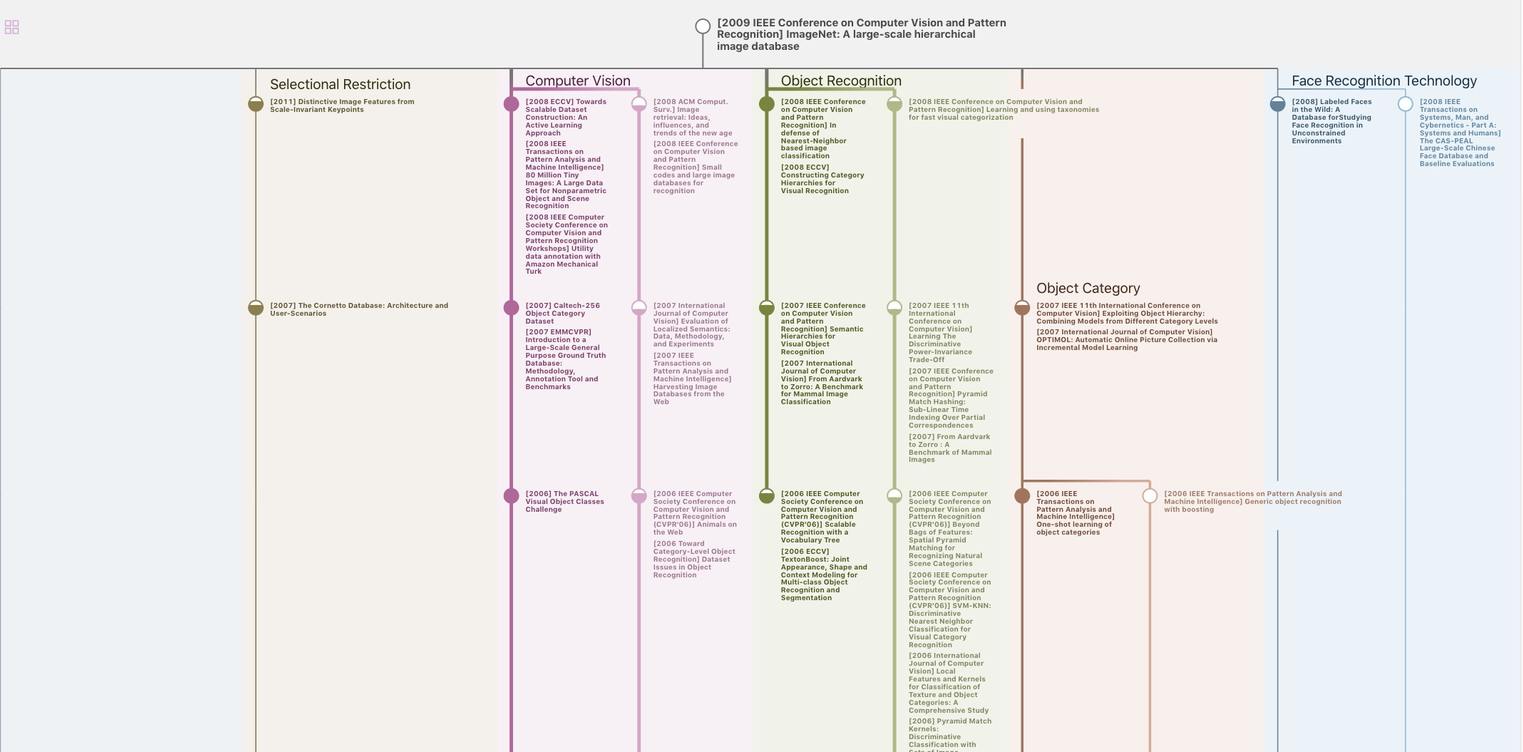
生成溯源树,研究论文发展脉络
Chat Paper
正在生成论文摘要