Self-Supervised Visual-Lidar Odometry With Flip Consistency
2021 IEEE WINTER CONFERENCE ON APPLICATIONS OF COMPUTER VISION WACV 2021(2021)
摘要
Most learning-based methods estimate ego-motion by utilizing visual sensors, which suffer from dramatic lighting variations and textureless scenarios. In this paper, we incorporate sparse but accurate depth measurements obtained from lidars to overcome the limitation of visual methods. To this end, we design a self-supervised visual-lidar odometry (Self-VLO) framework. It takes both monocular images and sparse depth maps projected from 3D lidar points as input, and produces pose and depth estimations in an end-to-end learning manner, without using any ground truth labels. To effectively fuse two modalities, we design a two-pathway encoder to extract features from visual and depth images and fuse the encoded features with those in decoders at multiple scales by our fusion module. We also adopt a siamese architecture and design an adaptively weighted flip consistency loss to facilitate the self-supervised learning of our VLO. Experiments on the KITTI odometry benchmark show that the proposed approach outperforms all self-supervised visual or lidar odometries. It also performs better than fully supervised VOs, demonstrating the power of fusion.
更多查看译文
关键词
flip consistency,self-supervised,visual-lidar
AI 理解论文
溯源树
样例
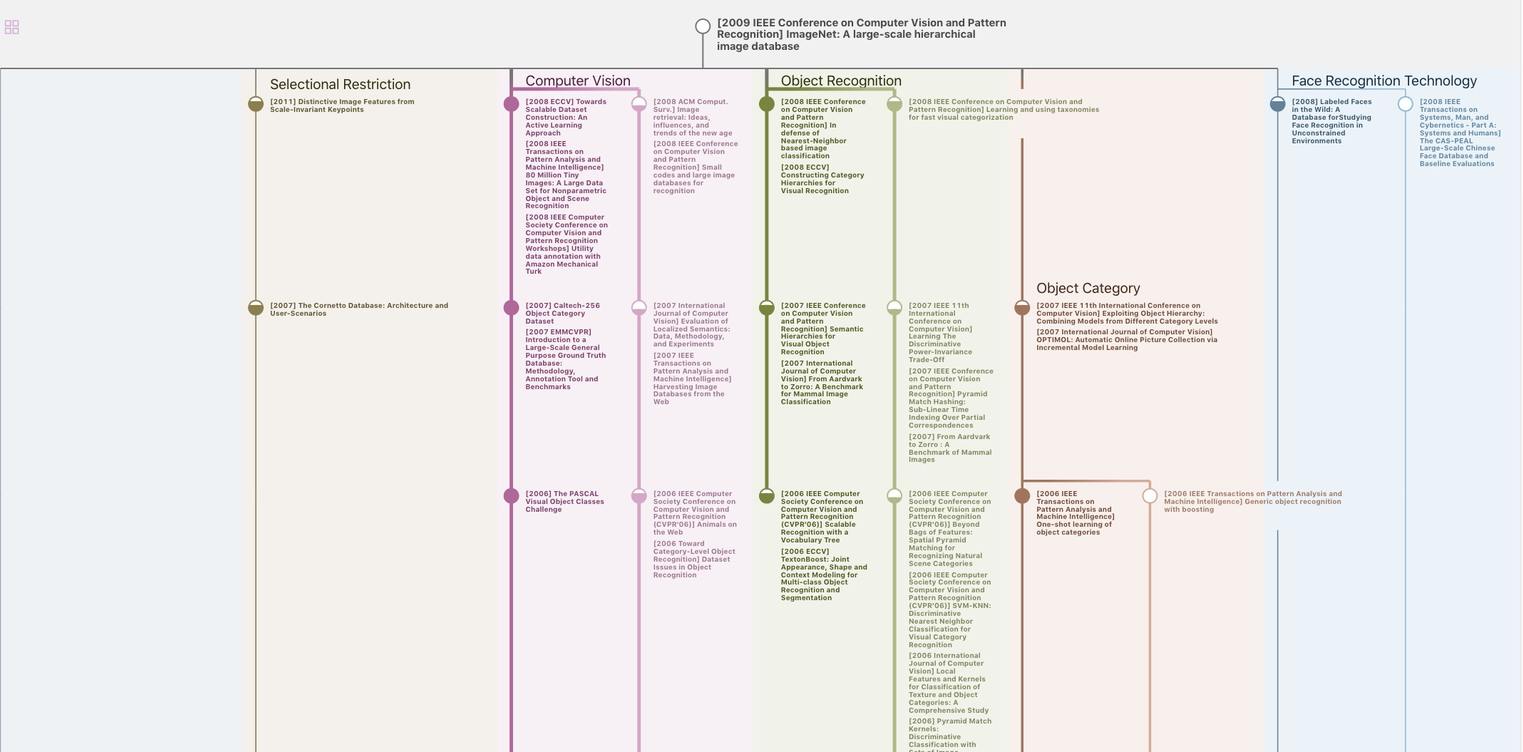
生成溯源树,研究论文发展脉络
Chat Paper
正在生成论文摘要