Interpreting the Eigenbasis of Principal Component Analysis to Identify Design Space Regions of Interest
Conference Proceedings of the Society for Experimental Mechanics Series(2019)
摘要
Computational models are a valuable tool for predicting the behavior of complex systems in regimes that are difficult, or infeasible to test. Uncertainties in a model resulting from lack of knowledge regarding parameters needed to define system behavior raise concern in simulations predictions, particularly those in extreme operating regimes. In such cases, calibration and validation of numerical models across a large operational domain is beneficial, but creates a high dimensional domain in which the model must be tested. Statistical decomposition methods present an ability to reduce the dimensionality of a measured or simulated quantity while maintaining relevant correlations across the domain, therefore providing insight to important relationships between input parameters and system response throughout many loading scenarios. Principal Component Analysis (PCA) is a commonly used statistical decomposition method used to produce a low order projection from a higher dimensional space. Herein, we evaluate a specific component of PCA, the eigenvectors providing statistical weighting to explanatory variables, to identify variable model sensitivity across high dimensional operational domains. The concept is demonstrated by simulation of an elasto-plastic material undergoing tensile testing at variable loading conditions. Results indicate that the eigenbasis reveals distinct relationships between a model's behavior and uncertain parameters, even when the relationship is not readily apparent and without relying on any a priori knowledge of system or material behavior.
更多查看译文
关键词
Principal Component Analysis,Sensitivity Analysis,Statistical Decomposition,Quantifications of Margins and Uncertainties,Quantities of Interest
AI 理解论文
溯源树
样例
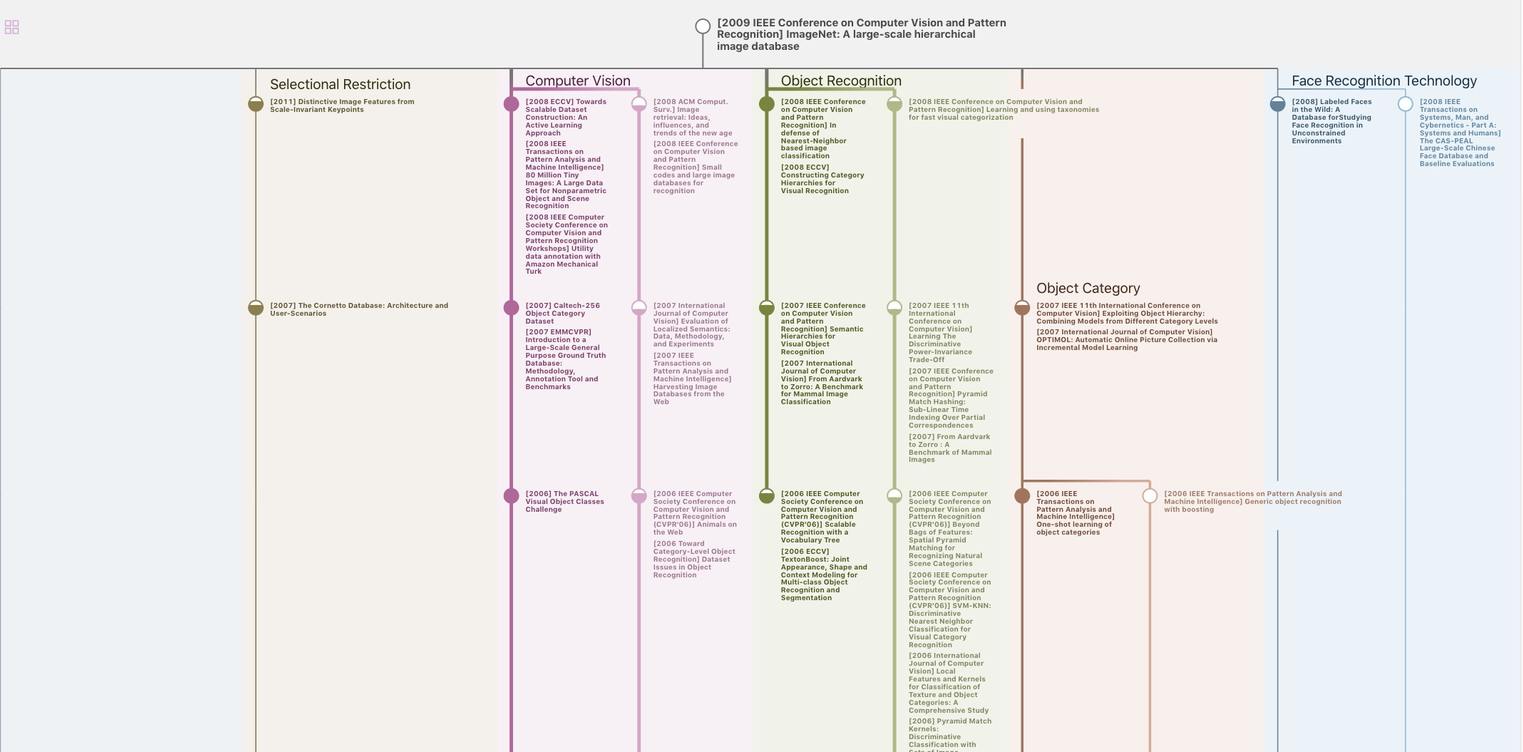
生成溯源树,研究论文发展脉络
Chat Paper
正在生成论文摘要