A Comparison of random forests and cluster analysis to identify ore deposits type using LA-ICPMS analysis of pyrite
LIFE WITH ORE DEPOSITS ON EARTH, PROCEEDINGS OF THE 15TH SGA BIENNIAL MEETING, 2019, VOLS 1-4(2019)
摘要
As exploration for new resources increasingly relies upon deeper and deeper drilling to investigate through overburden, exploration projects will encounter significantly higher drilling costs to sample target areas and to open new areas to exploration. Therefore, as much information as possible must be extracted from every drill hole. One tool that can be used is the in situ trace element analysis of individual mineral phases using LA-ICPMS. In this study, we investigate the use of pyrite trace element chemistry to fingerprint different ore deposit types so that appropriate geologic models can be employed at an early stage of exploration in new greenfields areas. While this data is effective at identifying ore deposit type, variability within the raw data leads to inherent complications for manual analysis. One way to deconvolute this data is to employ machine learning algorithms to aid in the classification. Here we develop a classifier using supervised classification (Random Forests) and further test non-supervised classification (cluster analysis) algorithms. The results of using Random Forests and cluster analysis to identify ore deposit type are then compared.
更多查看译文
AI 理解论文
溯源树
样例
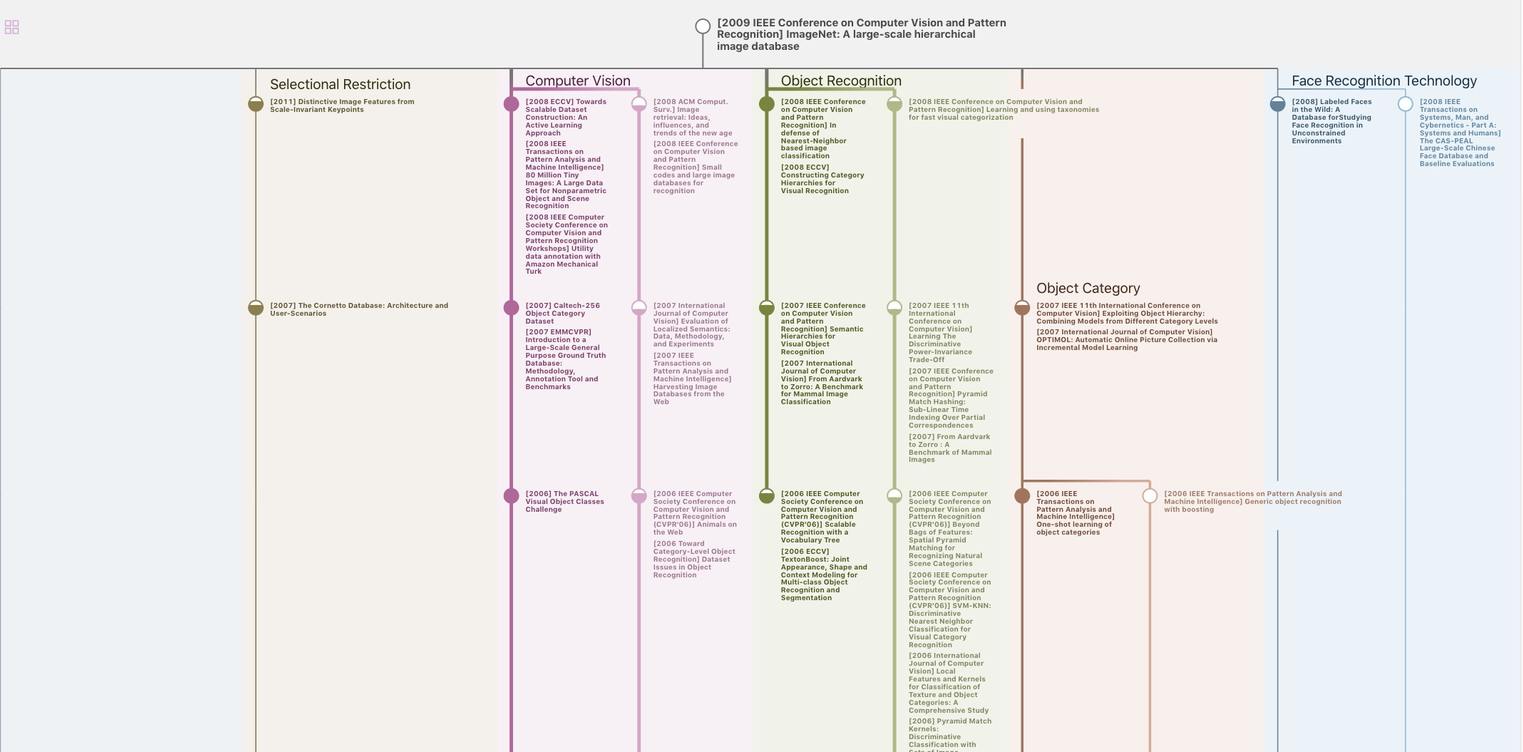
生成溯源树,研究论文发展脉络
Chat Paper
正在生成论文摘要