Adaptive density estimation on bounded domains under mixing conditions
ELECTRONIC JOURNAL OF STATISTICS(2020)
摘要
In this article, we propose a new adaptive estimator for multivariate density functions defined on a bounded domain in the framework of multivariate mixing processes. Several procedures have been proposed in the literature to tackle the boundary bias issue encountered using classical kernel estimators. Most of them are designed to work in dimension d = 1 or on the unit d-dimensional hypercube. We extend such results to more general bounded domains such as simple polygons or regular domains that satisfy a rolling condition. We introduce a specific family of kernel-type estimators devoid of boundary bias. We then propose a data-driven Goldenshluger and Lepski type procedure to jointly select a kernel and a bandwidth. We prove the optimality of our procedure in the adaptive framework, stating an oracle-type inequality. We illustrate the good behavior of our new class of estimators on simulated data. Finally, we apply our procedure to a real dataset.
更多查看译文
关键词
Compact supported density estimation,multivariate analysis,mixing stochastic processes
AI 理解论文
溯源树
样例
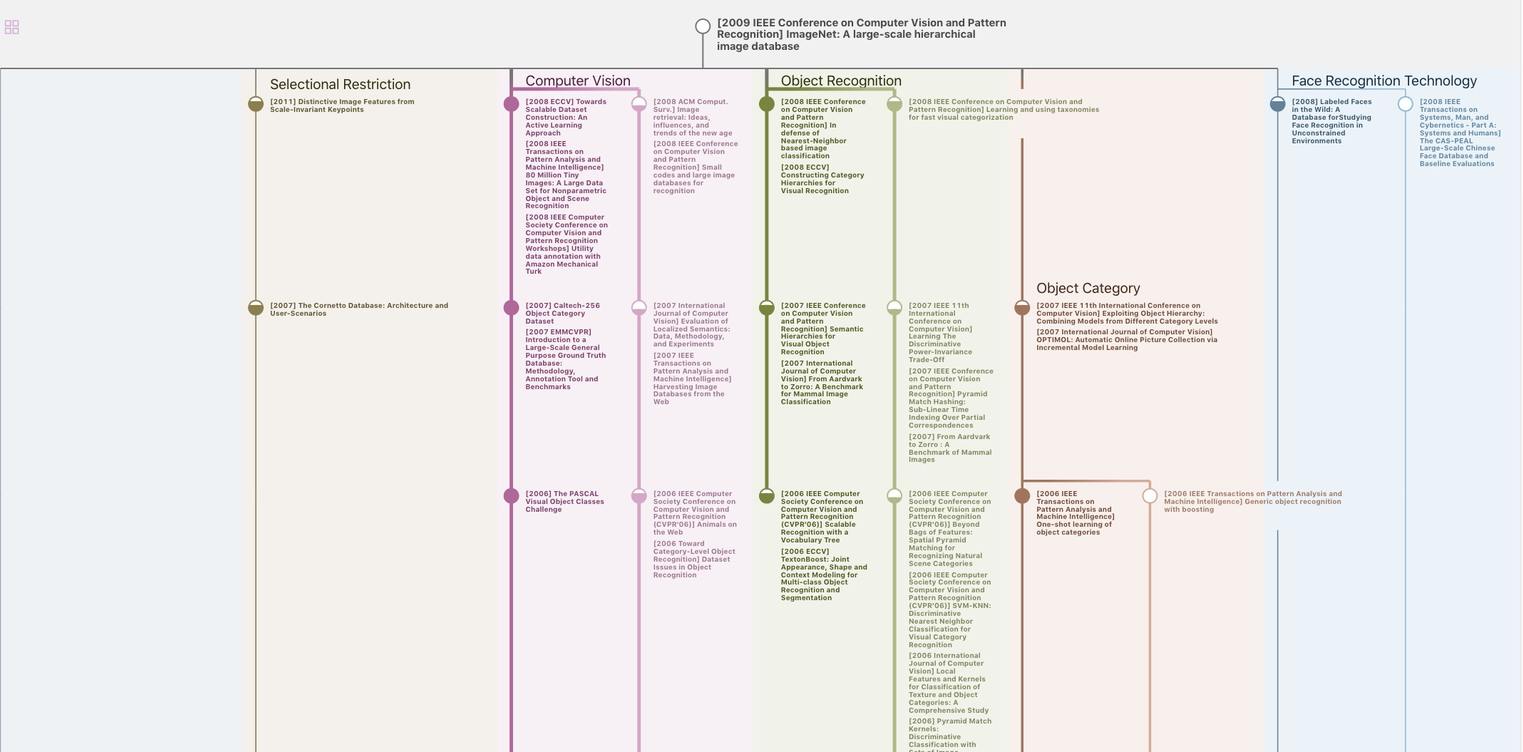
生成溯源树,研究论文发展脉络
Chat Paper
正在生成论文摘要