Reconstruction of Tree Network via Evolutionary Game Data Analysis
IEEE Transactions on Cybernetics(2022)
摘要
As one of the most effective technologies for network reconstruction, compressive sensing can recover signals from a small amount of observed data through sparse search or greedy algorithms in the assumption that the unknown signal is sufficiently sparse on a specific basis. However, there often occurs loss of precision even failure in the process of reconstruction without enough prior information. Therefore, the purpose of this article is to solve the problem of low reconstruction accuracy by mining implicit structural information in the network. Specifically, we propose a novel and efficient algorithm (MCM_TRA) for reconstructing the structure of the
$K$
-forked tree network. Based on evolutionary game dynamics, the modified clustering method (MCM) classifies all nodes into two sets, then a two-stage reconstruction algorithm (TRA) is illustrated to recover the node signals in different sets. The experimental results demonstrate that the MCM_TRA enhances the reconstruction accuracy prominently than previous algorithms. Moreover, extensive sensitivity analysis shows that the reconstruction effect can be promoted for a broad range of parameters, which further indicates the superiority of the proposed method.
更多查看译文
关键词
Algorithms,Cluster Analysis,Data Analysis,Trees
AI 理解论文
溯源树
样例
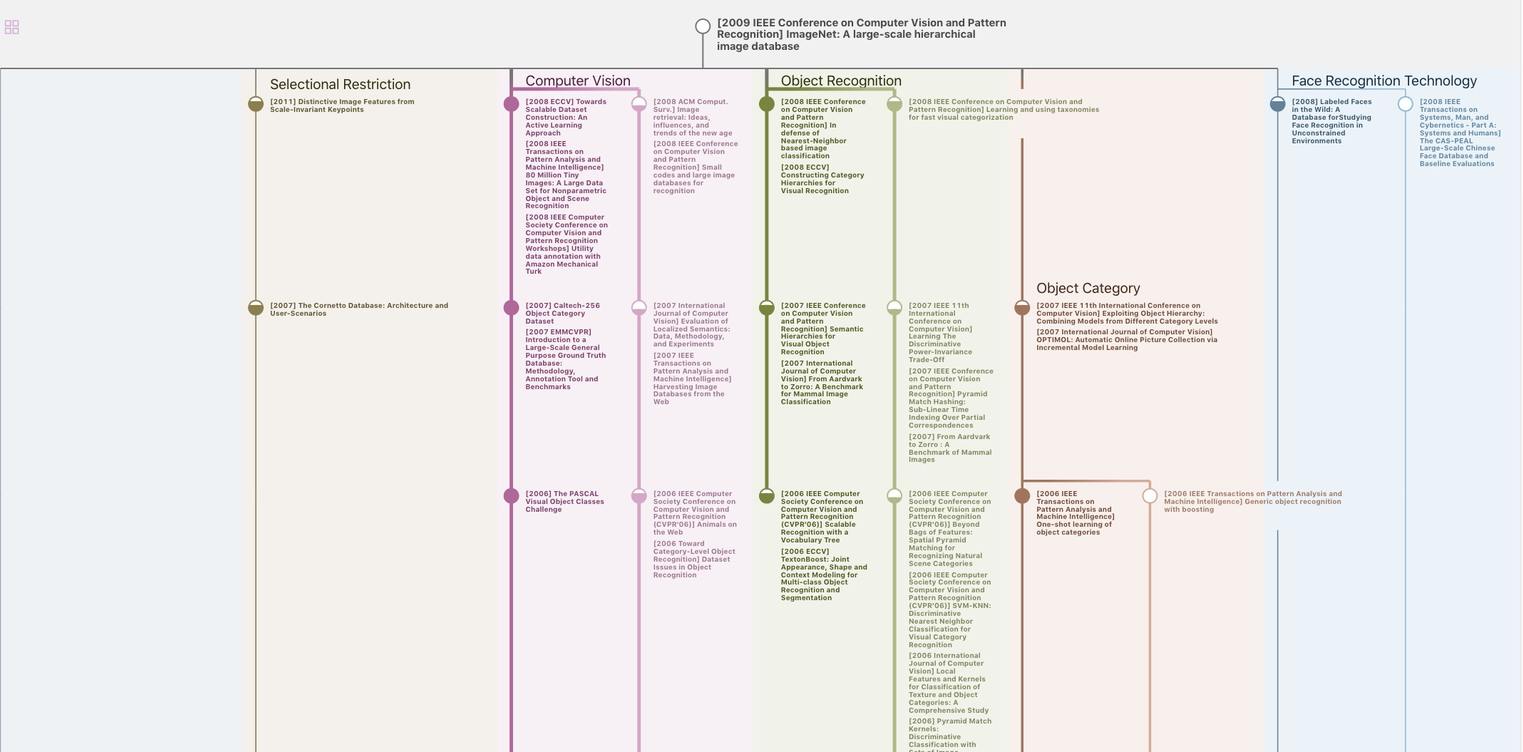
生成溯源树,研究论文发展脉络
Chat Paper
正在生成论文摘要