Multi-Channel Sequential Behavior Networks for User Modeling in Online Advertising
arxiv(2020)
摘要
Multiple content providers rely on native advertisement for revenue by placing ads within the organic content of their pages. We refer to this setting as ``queryless'' to differentiate from search advertisement where a user submits a search query and gets back related ads. Understanding user intent is critical because relevant ads improve user experience and increase the likelihood of delivering clicks that have value to our advertisers. This paper presents Multi-Channel Sequential Behavior Network (MC-SBN), a deep learning approach for embedding users and ads in a semantic space in which relevance can be evaluated. Our proposed user encoder architecture summarizes user activities from multiple input channels--such as previous search queries, visited pages, or clicked ads--into a user vector. It uses multiple RNNs to encode sequences of event sessions from the different channels and then applies an attention mechanism to create the user representation. A key property of our approach is that user vectors can be maintained and updated incrementally, which makes it feasible to be deployed for large-scale serving. We conduct extensive experiments on real-world datasets. The results demonstrate that MC-SBN can improve the ranking of relevant ads and boost the performance of both click prediction and conversion prediction in the queryless native advertising setting.
更多查看译文
关键词
online advertising,user modeling,networks,multi-channel
AI 理解论文
溯源树
样例
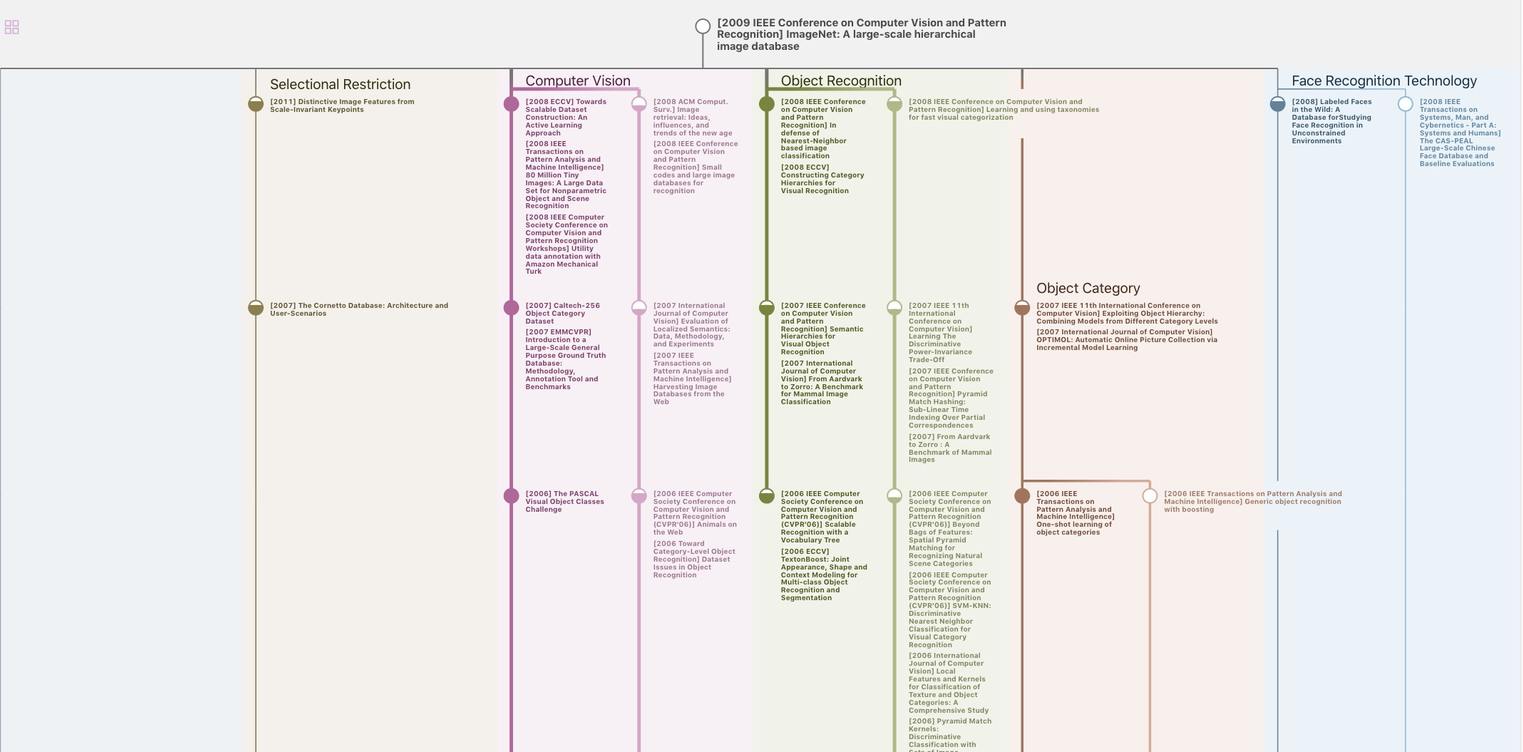
生成溯源树,研究论文发展脉络
Chat Paper
正在生成论文摘要