CLEAR: Contrastive Learning for Sentence Representation
arxiv(2020)
摘要
Pre-trained language models have proven their unique powers in capturing implicit language features. However, most pre-training approaches focus on the word-level training objective, while sentence-level objectives are rarely studied. In this paper, we propose Contrastive LEArning for sentence Representation (CLEAR), which employs multiple sentence-level augmentation strategies in order to learn a noise-invariant sentence representation. These augmentations include word and span deletion, reordering, and substitution. Furthermore, we investigate the key reasons that make contrastive learning effective through numerous experiments. We observe that different sentence augmentations during pre-training lead to different performance improvements on various downstream tasks. Our approach is shown to outperform multiple existing methods on both SentEval and GLUE benchmarks.
更多查看译文
关键词
contrastive learning,sentence representation
AI 理解论文
溯源树
样例
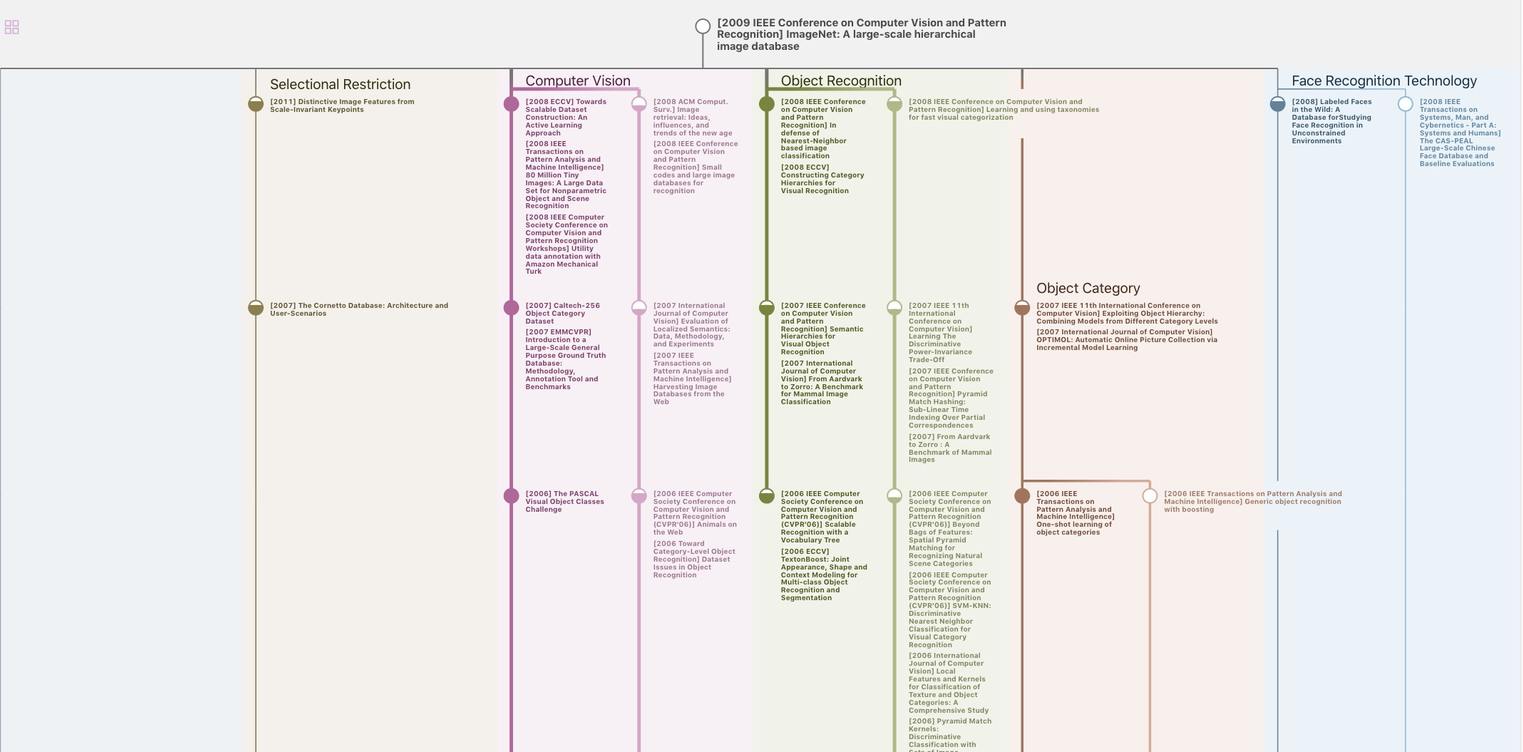
生成溯源树,研究论文发展脉络
Chat Paper
正在生成论文摘要