Towards Reducing Severe Defocus Spread Effects For Multi-Focus Image Fusion Via An Optimization Based Strategy
IEEE TRANSACTIONS ON COMPUTATIONAL IMAGING(2020)
摘要
Multi-focus image fusion (MFF) is a popular technique to generate an all-in-focus image, where all objects in the scene are sharp. However, existing methods pay little attention to defocus spread effects of the real-world multi-focus images. Consequently, most of the methods perform badly in the areas near focus map boundaries. According to the idea that each local region in the fused image should be similar to the sharpest one among source images, this paper presents an optimization-based approach to reduce defocus spread effects. Firstly, a new MFF assessment metric is presented by combining the principle of structure similarity and detected focus maps. Then, MFF problem is cast into maximizing this metric. The optimization is solved by gradient ascent. Experiments conducted on the real-world dataset verify superiority of the proposed model. The codes are available at https://github.com/xsxjtu/MFF-SSIM.
更多查看译文
关键词
Multi-focus image fusion, defocus spread effect, structure similarity
AI 理解论文
溯源树
样例
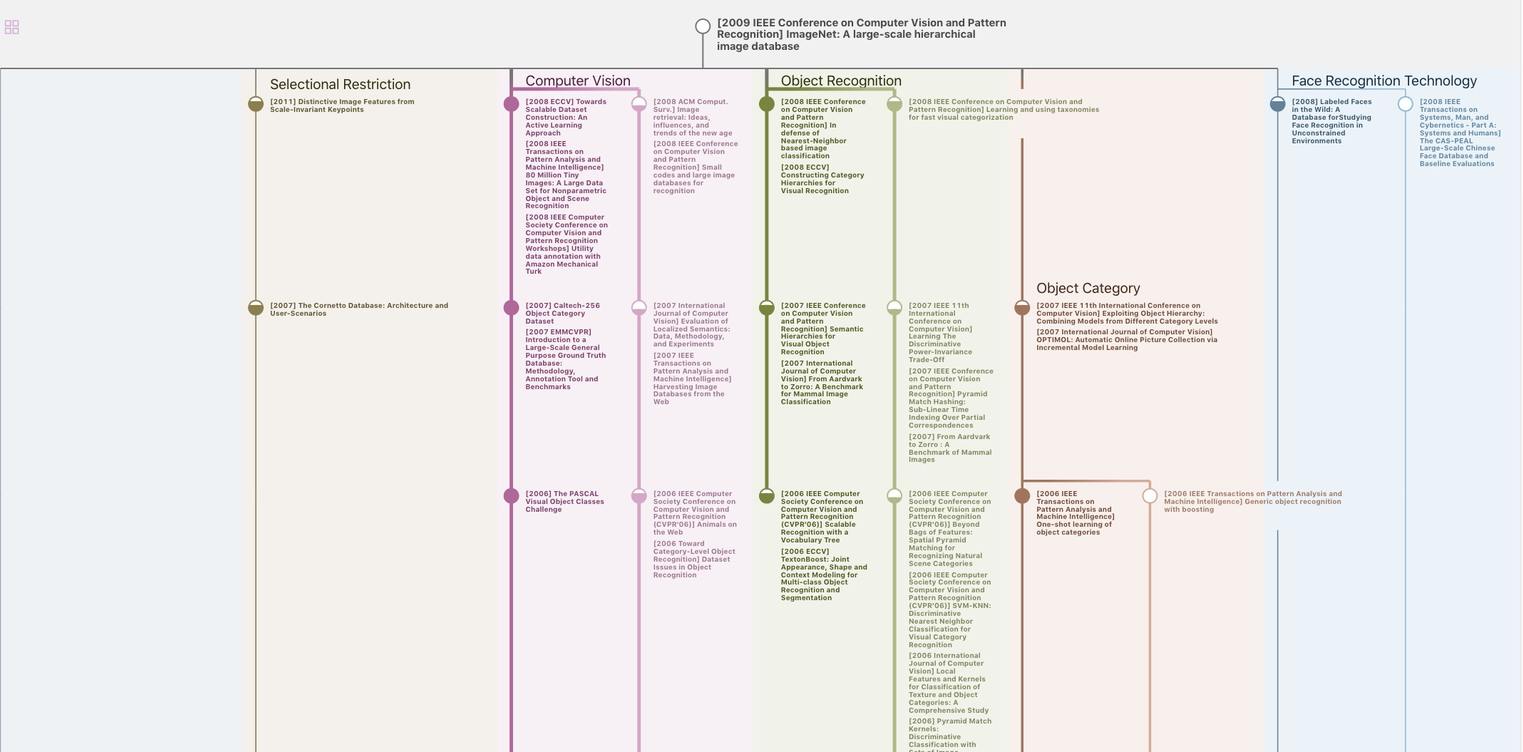
生成溯源树,研究论文发展脉络
Chat Paper
正在生成论文摘要