A Hyperspectral Image Classification Approach Based on Feature Fusion and Multi-Layered Gradient Boosting Decision Trees.
Entropy (Basel, Switzerland)(2020)
Abstract
At present, many Deep Neural Network (DNN) methods have been widely used for hyperspectral image classification. Promising classification results have been obtained by utilizing such models. However, due to the complexity and depth of the model, increasing the number of model parameters may lead to an overfitting of the model, especially when training data are insufficient. As the performance of the model mainly depends on sufficient data and a large network with reasonably optimized hyperparameters, using DNNs for classification requires better hardware conditions and sufficient training time. This paper proposes a feature fusion and multi-layered gradient boosting decision tree model (FF-DT) for hyperspectral image classification. First, we fuse extended morphology profiles (EMPs), linear multi-scale spatial characteristics, and nonlinear multi-scale spatial characteristics as final features to extract both special and spectral features. Furthermore, a multi-layered gradient boosting decision tree model is constructed for classification. We conduct experiments based on three datasets, which in this paper are referred to as the Pavia University, Indiana Pines, and Salinas datasets. It is shown that the proposed FF-DT achieves better performance in classification accuracy, training conditions, and time consumption than other current classical hyperspectral image classification methods.
MoreTranslated text
Key words
hyperspectral image,multi-layered gradient boosting decision trees (mGBDTs),feature fusion,image classification
AI Read Science
Must-Reading Tree
Example
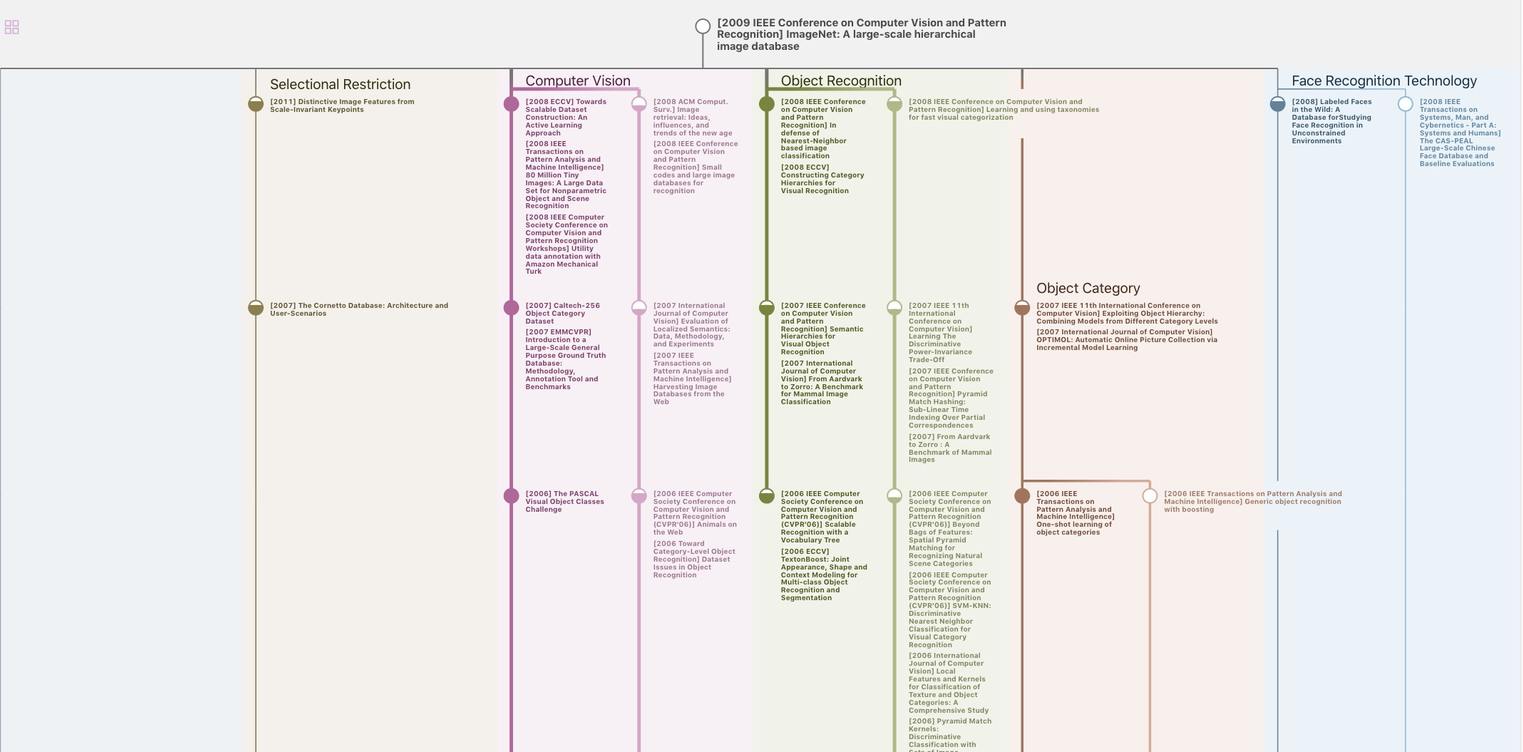
Generate MRT to find the research sequence of this paper
Chat Paper
Summary is being generated by the instructions you defined