Self-Supervised Representation Learning For Astronomical Images
ASTROPHYSICAL JOURNAL LETTERS(2021)
摘要
Sky surveys are the largest data generators in astronomy, making automated tools for extracting meaningful scientific information an absolute necessity. We show that, without the need for labels, self-supervised learning recovers representations of sky survey images that are semantically useful for a variety of scientific tasks. These representations can be directly used as features, or fine-tuned, to outperform supervised methods trained only on labeled data. We apply a contrastive learning framework on multiband galaxy photometry from the Sloan Digital Sky Survey (SDSS), to learn image representations. We then use them for galaxy morphology classification and fine-tune them for photometric redshift estimation, using labels from the Galaxy Zoo 2 data set and SDSS spectroscopy. In both downstream tasks, using the same learned representations, we outperform the supervised state-of-the-art results, and we show that our approach can achieve the accuracy of supervised models while using 2-4 times fewer labels for training. The codes, trained models, and data can be found at https://portal.nersc.gov/ project/dasrepo/self-supervised-learning-sdss.
更多查看译文
关键词
astronomical images,learning,representation,self-supervised
AI 理解论文
溯源树
样例
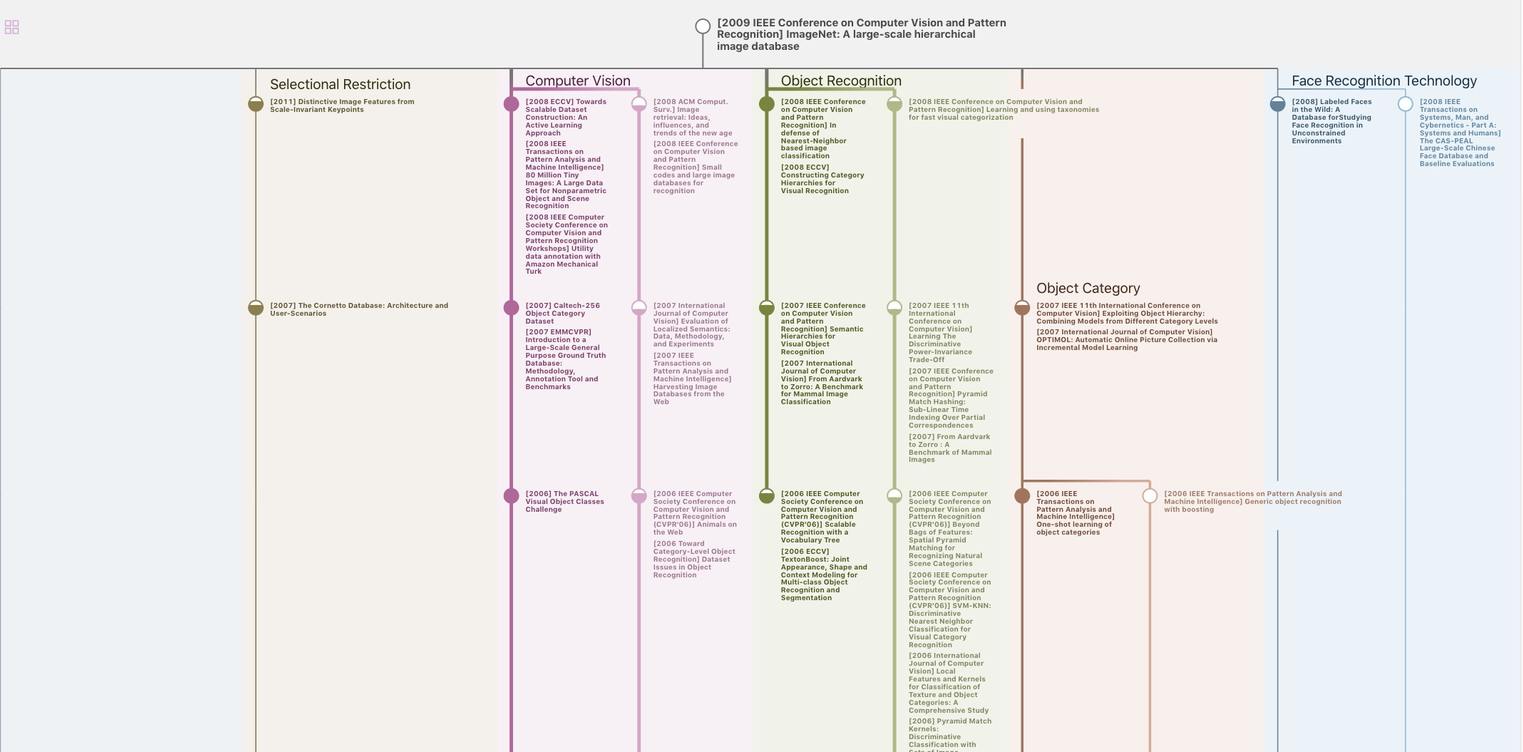
生成溯源树,研究论文发展脉络
Chat Paper
正在生成论文摘要