Analyzing Representations inside Convolutional Neural Networks
arxiv(2020)
摘要
How can we discover and succinctly summarize the concepts that a neural network has learned? Such a task is of great importance in applications of networks in areas of inference that involve classification, like medical diagnosis based on fMRI/x-ray etc. In this work, we propose a framework to categorize the concepts a network learns based on the way it clusters a set of input examples, clusters neurons based on the examples they activate for, and input features all in the same latent space. This framework is unsupervised and can work without any labels for input features, it only needs access to internal activations of the network for each input example, thereby making it widely applicable. We extensively evaluate the proposed method and demonstrate that it produces human-understandable and coherent concepts that a ResNet-18 has learned on the CIFAR-100 dataset.
更多查看译文
关键词
convolutional neural networks,representations,neural networks
AI 理解论文
溯源树
样例
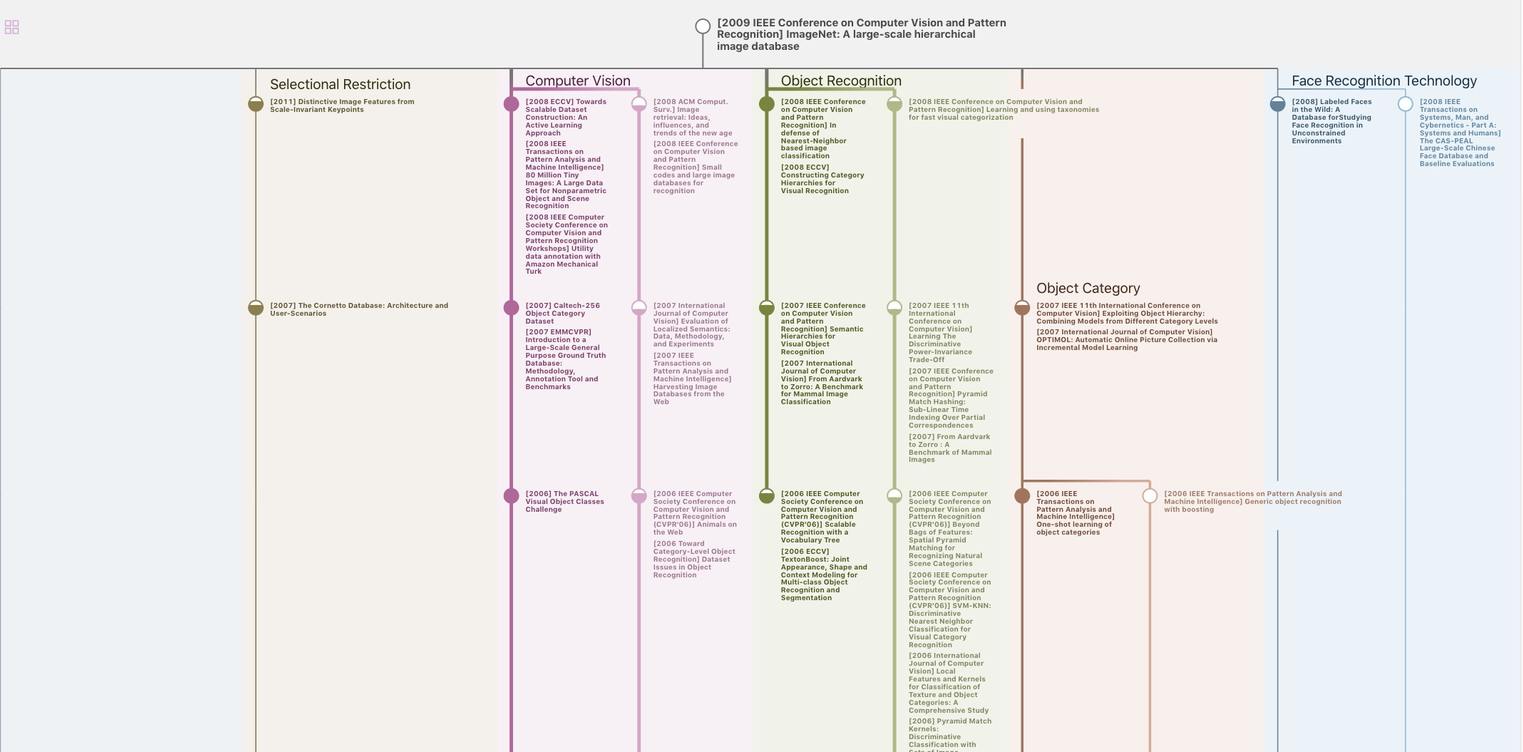
生成溯源树,研究论文发展脉络
Chat Paper
正在生成论文摘要