RAP-NET: COARSE-TO-FINE MULTI-ORGAN SEGMENTATION WITH SINGLE RANDOM ANATOMICAL PRIOR
2021 IEEE 18TH INTERNATIONAL SYMPOSIUM ON BIOMEDICAL IMAGING (ISBI)(2021)
摘要
Performing coarse-to-fine abdominal multi-organ segmentation facilitates extraction of high-resolution segmentation minimizing the loss of spatial contextual information. However, current coarse-to-refine approaches require a significant number of models to perform single organ segmentation. We propose a coarse-to-fine pipeline RAP-Net, which starts from the extraction of the global prior context of multiple organs from 3D volumes using a low-resolution coarse network, followed by a fine phase that uses a single refined model to segment all abdominal organs instead of multiple organ corresponding models. We combine the anatomical prior with corresponding extracted patches to preserve the anatomical locations and boundary information for performing high-resolution segmentation across all organs in a single model. To train and evaluate our method, a clinical research cohort consisting of 100 patient volumes with 13 organs well-annotated is used. We tested our algorithms with 4-fold cross-validation and computed the Dice score for evaluating the segmentation performance of the 13 organs. Our proposed method using single auto-context outperforms the state-of-the-art on 13 models with an average Dice score 84.58% versus 81.69% (p<0.0001).
更多查看译文
关键词
Computed Tomography, Abdominal Multi-Organ Segmentation, Random Anatomical Prior, Single Multi-Organ Patch Model
AI 理解论文
溯源树
样例
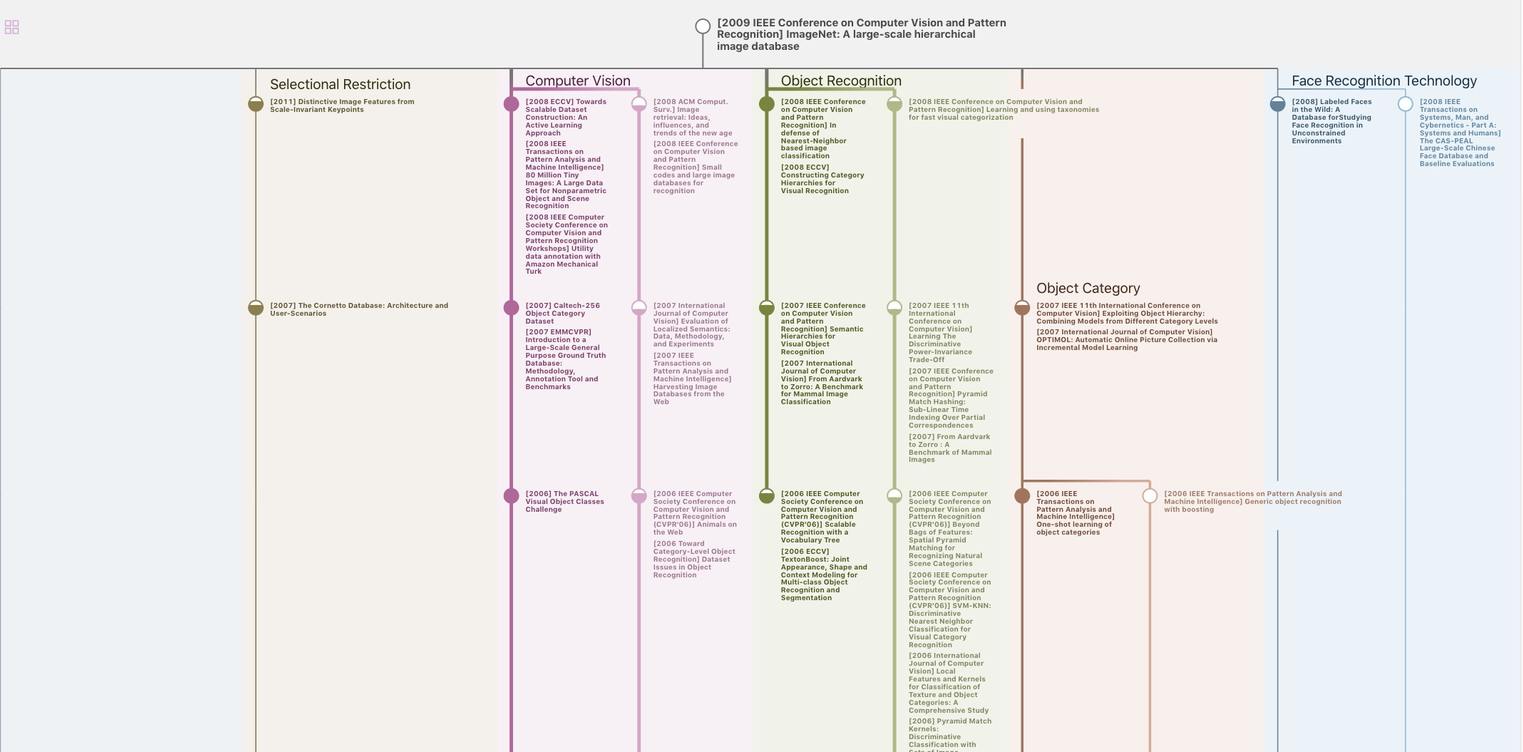
生成溯源树,研究论文发展脉络
Chat Paper
正在生成论文摘要