Emergent Hand Morphology and Control from Optimizing Robust Grasps of Diverse Objects
2021 IEEE INTERNATIONAL CONFERENCE ON ROBOTICS AND AUTOMATION (ICRA 2021)(2021)
Abstract
Evolution in nature illustrates that the creatures’ biological structure and their sensorimotor skills adapt to the environmental changes for survival. Likewise, the ability to morph and acquire new skills can facilitate an embodied agent to solve tasks of varying complexities. In this work, we introduce a data-driven approach where effective hand designs naturally emerge for the purpose of grasping diverse objects. Jointly optimizing morphology and control imposes computational challenges since it requires constant evaluation of a black-box function that measures the performance of a combination of embodiment and behavior. We develop a novel Bayesian Optimization algorithm that efficiently co-designs the morphology and grasping skills through learned latent-space representations. We design the grasping tasks based on a taxonomy of human grasp types: power grasp, pinch grasp, and lateral grasp. Through experimentation and comparative study, we demonstrate that our approach discovers robust and cost-efficient hand morphologies for grasping novel objects. Additional videos and results at https://xinleipan.github.io/emergent_morphology
MoreTranslated text
Key words
data-driven approach,constant evaluation,black-box function,grasping skills,Bayesian optimization algorithm,latent-space representations,grasping tasks,human grasp types,cost-efficient hand morphologies,hand morphology,optimizing robust grasps,sensorimotor skills,environmental changes,embodied agent
AI Read Science
Must-Reading Tree
Example
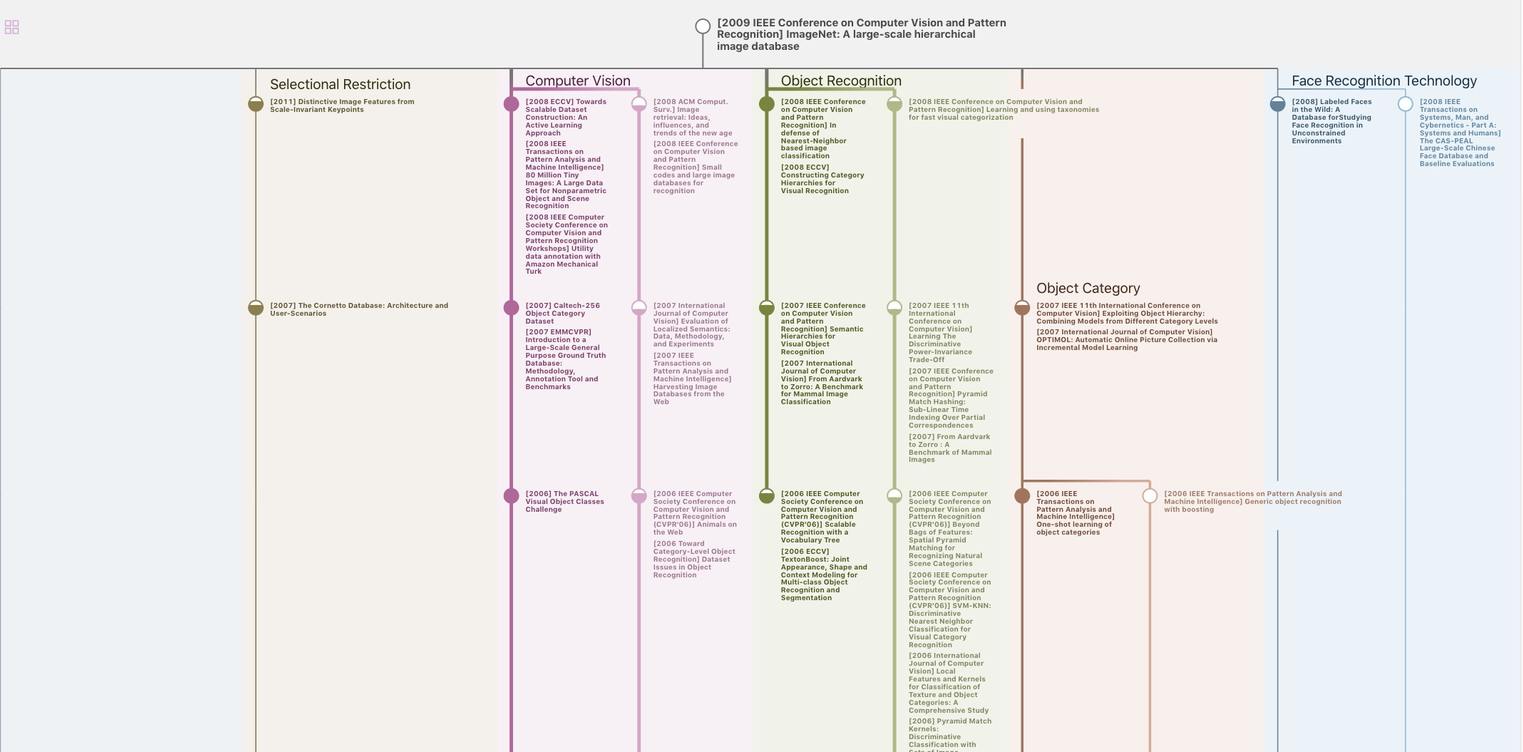
Generate MRT to find the research sequence of this paper
Chat Paper
Summary is being generated by the instructions you defined