High-Speed Robot Navigation using Predicted Occupancy Maps
2021 IEEE INTERNATIONAL CONFERENCE ON ROBOTICS AND AUTOMATION (ICRA 2021)(2021)
摘要
Safe and high-speed navigation is a key enabling capability for real world deployment of robotic systems. A significant limitation of existing approaches is the computational bottleneck associated with explicit mapping and the limited field of view (FOV) of existing sensor technologies. In this paper, we study algorithmic approaches that allow the robot to predict spaces extending beyond the sensor horizon for robust planning at high speeds. We accomplish this using a generative neural network trained from real-world data without requiring human annotated labels. Further, we extend our existing control algorithms to support leveraging the predicted spaces to improve collision-free planning and navigation at high speeds. Our experiments are conducted on a physical robot based on the MIT race car using an RGBD sensor where were able to demonstrate improved performance at 4 m/s compared to a controller not operating on predicted regions of the map.
更多查看译文
关键词
robust planning,generative neural network,human annotated labels,predicted spaces,collision-free planning,physical robot,RGBD sensor,predicted regions,high-speed robot navigation,robotic systems,computational bottleneck,explicit mapping,algorithmic approaches,sensor horizon,predicted occupancy maps,field of view,FOV,MIT race car,velocity 4.0 m/s
AI 理解论文
溯源树
样例
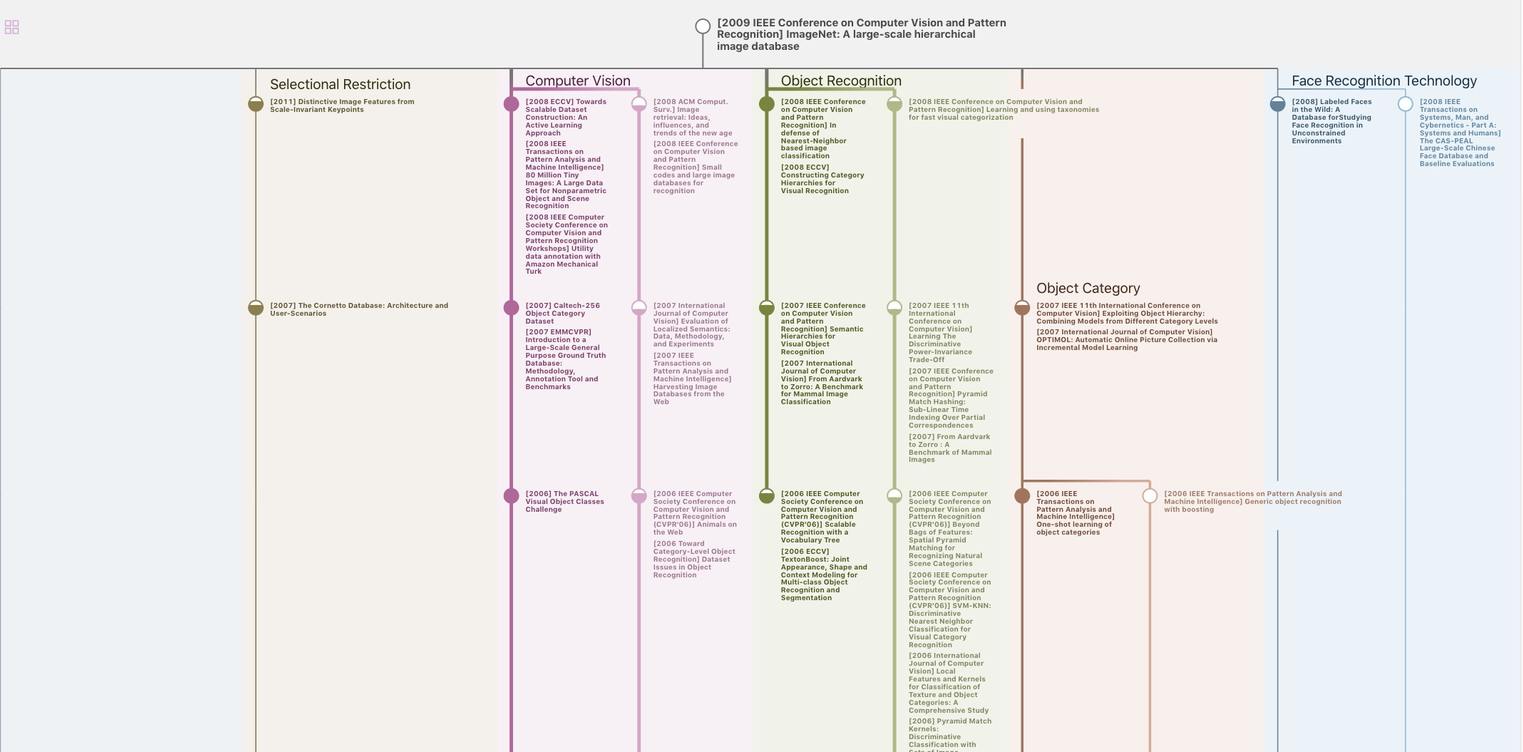
生成溯源树,研究论文发展脉络
Chat Paper
正在生成论文摘要