Data Assimilation in the Latent Space of a Neural Network
arxiv(2020)
摘要
There is an urgent need to build models to tackle Indoor Air Quality issue. Since the model should be accurate and fast, Reduced Order Modelling technique is used to reduce the dimensionality of the problem. The accuracy of the model, that represent a dynamic system, is improved integrating real data coming from sensors using Data Assimilation techniques. In this paper, we formulate a new methodology called Latent Assimilation that combines Data Assimilation and Machine Learning. We use a Convolutional neural network to reduce the dimensionality of the problem, a Long-Short-Term-Memory to build a surrogate model of the dynamic system and an Optimal Interpolated Kalman Filter to incorporate real data. Experimental results are provided for CO2 concentration within an indoor space. This methodology can be used for example to predict in real-time the load of virus, such as the SARS-COV-2, in the air by linking it to the concentration of CO2.
更多查看译文
关键词
data assimilation,latent space,neural network
AI 理解论文
溯源树
样例
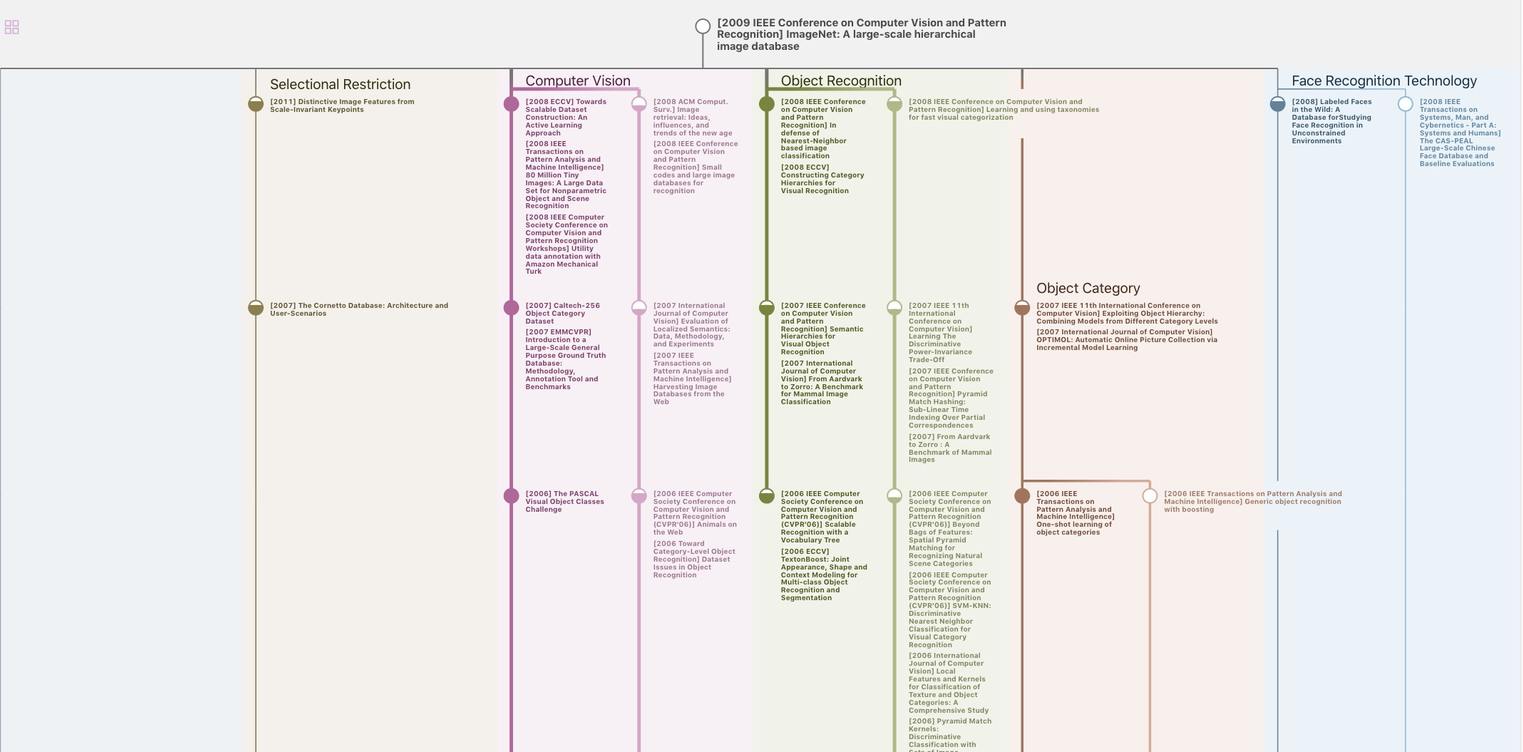
生成溯源树,研究论文发展脉络
Chat Paper
正在生成论文摘要