To Talk or to Work: Flexible Communication Compression for Energy Efficient Federated Learning over Heterogeneous Mobile Edge Devices
IEEE CONFERENCE ON COMPUTER COMMUNICATIONS (IEEE INFOCOM 2021)(2021)
摘要
Recent advances in machine learning, wireless communication, and mobile hardware technologies promisingly enable federated learning (FL) over massive mobile edge devices, which opens new horizons for numerous intelligent mobile applications. Despite the potential benefits, FL imposes huge communication and computation burdens on participating devices due to periodical global synchronization and continuous local training, raising great challenges to battery constrained mobile devices. In this work, we target at improving the energy efficiency of FL over mobile edge networks to accommodate heterogeneous participating devices without sacrificing the learning performance. To this end, we develop a convergence-guaranteed FL algorithm enabling flexible communication compression. Guided by the derived convergence bound, we design a compression control scheme to balance the energy consumption of local computing (i.e., "working") and wireless communication (i.e., "talking") from the long-term learning perspective. In particular, the compression parameters are elaborately chosen fir FL participants adapting to their computing and communication environments. Extensive simulations are conducted using various datasets to validate our theoretical analysis, and the results also demonstrate the efficacy of the proposed scheme in energy saving.
更多查看译文
关键词
Federated Learning over Wireless Networks, Gradient Compression, Local SGD, Edge Computing on GPUs
AI 理解论文
溯源树
样例
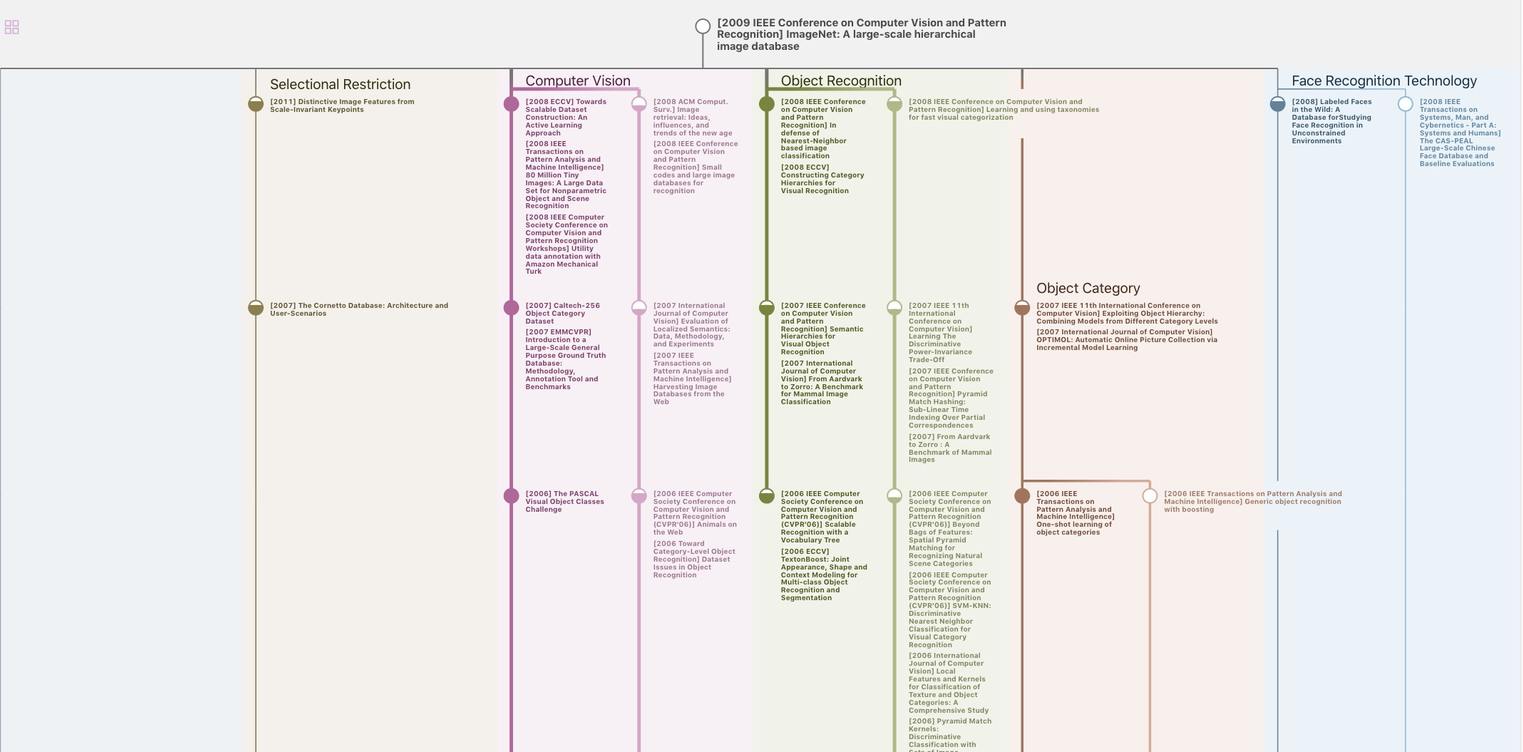
生成溯源树,研究论文发展脉络
Chat Paper
正在生成论文摘要