A Distributional Approach to Controlled Text Generation
ICLR(2021)
摘要
We propose a Distributional Approach to address Controlled Text Generation from pre-trained Language Models (LMs). This view permits to define, in a single formal framework, "pointwise" and "distributional" constraints over the target LM -- to our knowledge, this is the first approach with such generality -- while minimizing KL divergence with the initial LM distribution. The optimal target distribution is then uniquely determined as an explicit EBM (Energy-Based Model) representation. From that optimal representation we then train the target controlled autoregressive LM through an adaptive distributional variant of Policy Gradient. We conduct a first set of experiments over pointwise constraints showing the advantages of our approach over a set of baselines, in terms of obtaining a controlled LM balancing constraint satisfaction with divergence from the initial LM (GPT-2). We then perform experiments over distributional constraints, a unique feature of our approach, demonstrating its potential as a remedy to the problem of Bias in Language Models. Through an ablation study we show the effectiveness of our adaptive technique for obtaining faster convergence.
更多查看译文
关键词
controlled text generation,distributional approach
AI 理解论文
溯源树
样例
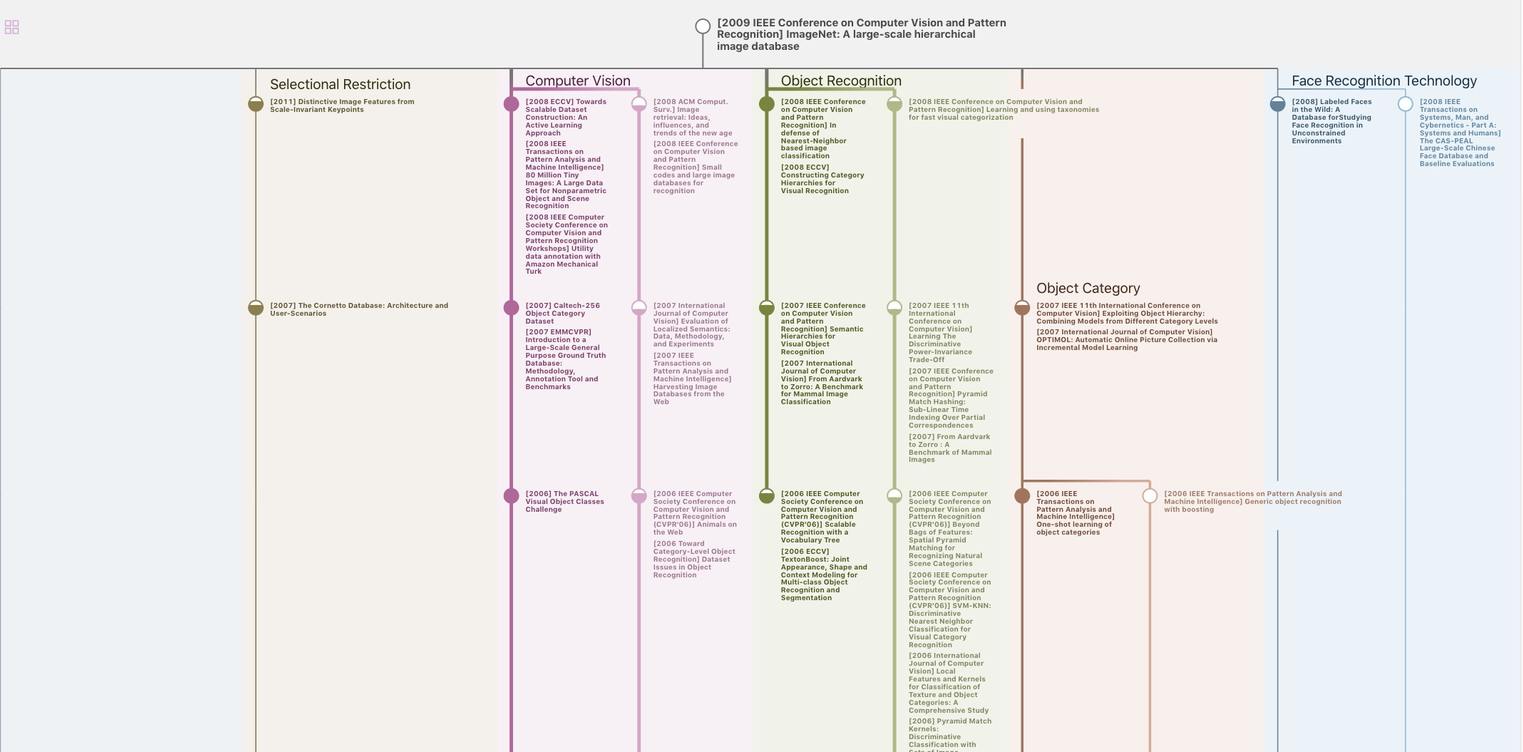
生成溯源树,研究论文发展脉络
Chat Paper
正在生成论文摘要