Improvement of the cross-entropy method in high dimension through a one-dimensional projection without gradient estimation
arxiv(2021)
摘要
Rare event probability estimation is an important topic in reliability analysis. Stochastic methods, such as importance sampling, have been developed to estimate such probabilities but they often fail in high dimension. In this paper, we propose a simple cross-entropy-based importance sampling algorithm to improve rare event estimation in high dimension. We consider the cross-entropy method with Gaussian auxiliary distributions and we suggest to update the Gaussian covariance matrix only in a one-dimensional subspace. The idea is to project in the one dimensional subspace spanned by the Gaussian mean, which gives an influential direction for the variance estimation. This approach does not require any additional simulation budget compared to the basic cross-entropy algorithm and greatly improves its performance in high dimension, as we show on different numerical test cases.
更多查看译文
关键词
Rare event simulation, Failure probability, Importance sampling, Cross-entropy, High dimension, Projection
AI 理解论文
溯源树
样例
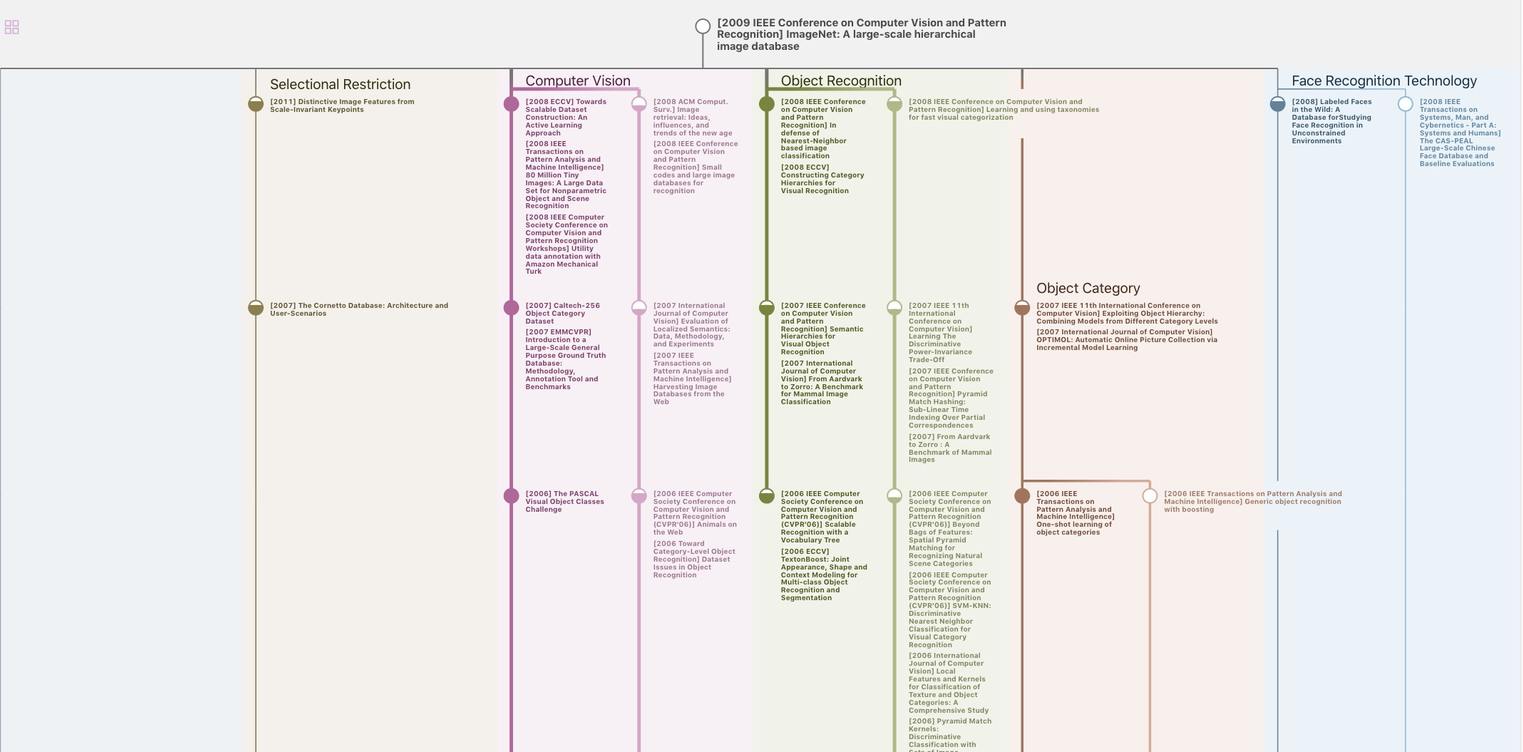
生成溯源树,研究论文发展脉络
Chat Paper
正在生成论文摘要