Predictive Digital Twins: Where Dynamic Data-Driven Learning Meets Physics-Based Modeling
DDDAS(2020)
摘要
A digital twin is an evolving virtual model that mirrors an individual physical asset throughout its lifecycle. An asset-specific model is a powerful tool to underpin intelligent automation and drive key decisions. The formulations and methods of dynamic data-driven application systems (DDDAS) have a key role to play in the tasks of inference, assimilation, prediction, control, and planning that enable the digital twin paradigm. Of particular importance is a tight feedback loop between models and data, which has long been a central concept in DDDAS. This keynote talk presents an approach to create, update, and deploy data-driven physics-based digital twins. We demonstrate the approach through the development of a structural digital twin for a custom-built unmanned aerial vehicle.
更多查看译文
关键词
Dynamic data,Machine learning,Computer science,Artificial intelligence,Physics based
AI 理解论文
溯源树
样例
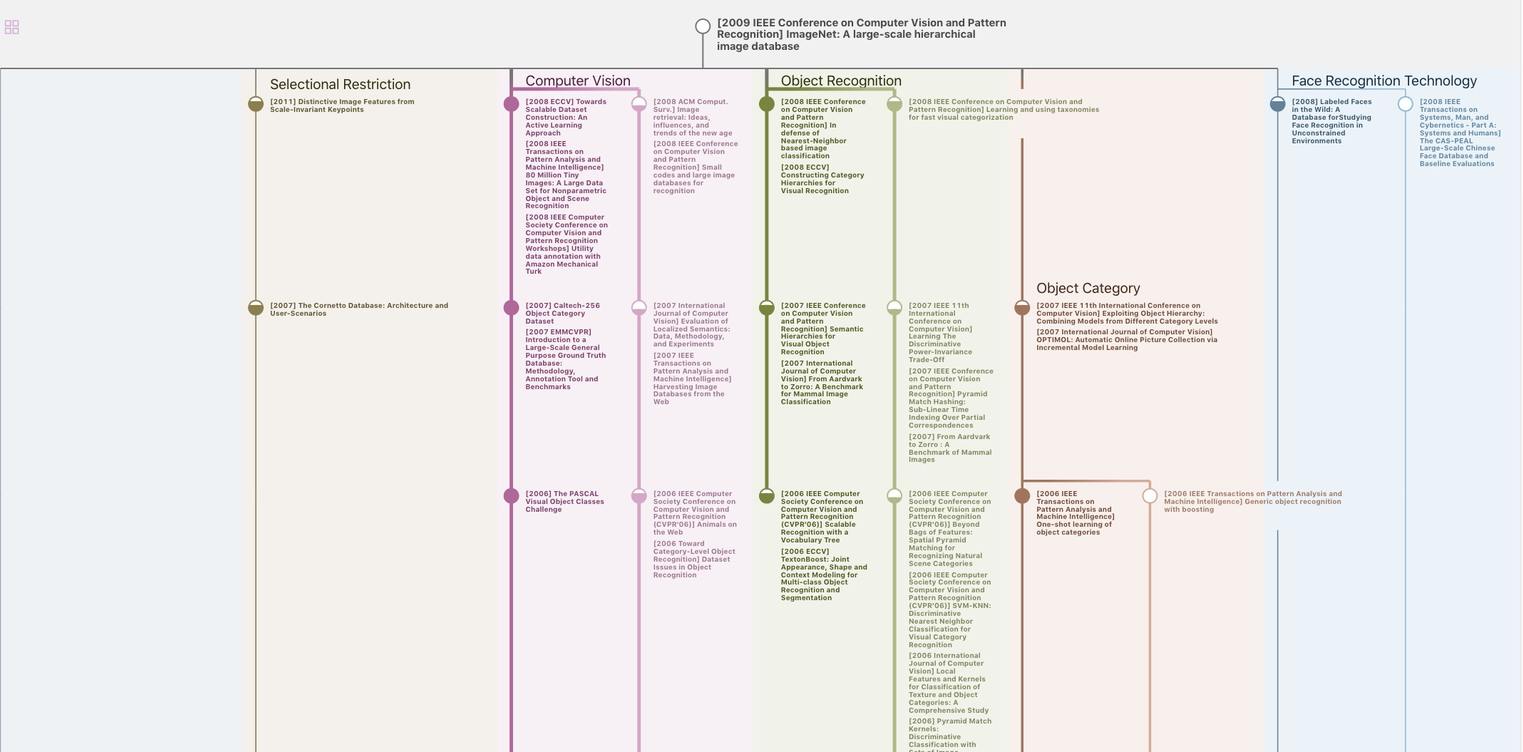
生成溯源树,研究论文发展脉络
Chat Paper
正在生成论文摘要