DenseHMM: Learning Hidden Markov Models by Learning Dense Representations.
International Conference on Pattern Recognition Applications and Methods (ICPRAM)(2022)
摘要
We propose DenseHMM - a modification of Hidden Markov Models (HMMs) that allows to learn dense representations of both the hidden states and the observables. Compared to the standard HMM, transition probabilities are not atomic but composed of these representations via kernelization. Our approach enables constraint-free and gradient-based optimization. We propose two optimization schemes that make use of this: a modification of the Baum-Welch algorithm and a direct co-occurrence optimization. The latter one is highly scalable and comes empirically without loss of performance compared to standard HMMs. We show that the non-linearity of the kernelization is crucial for the expressiveness of the representations. The properties of the DenseHMM like learned co-occurrences and log-likelihoods are studied empirically on synthetic and biomedical datasets.
更多查看译文
关键词
Hidden Markov Model,Representation Learning,Gradient Descent Optimization
AI 理解论文
溯源树
样例
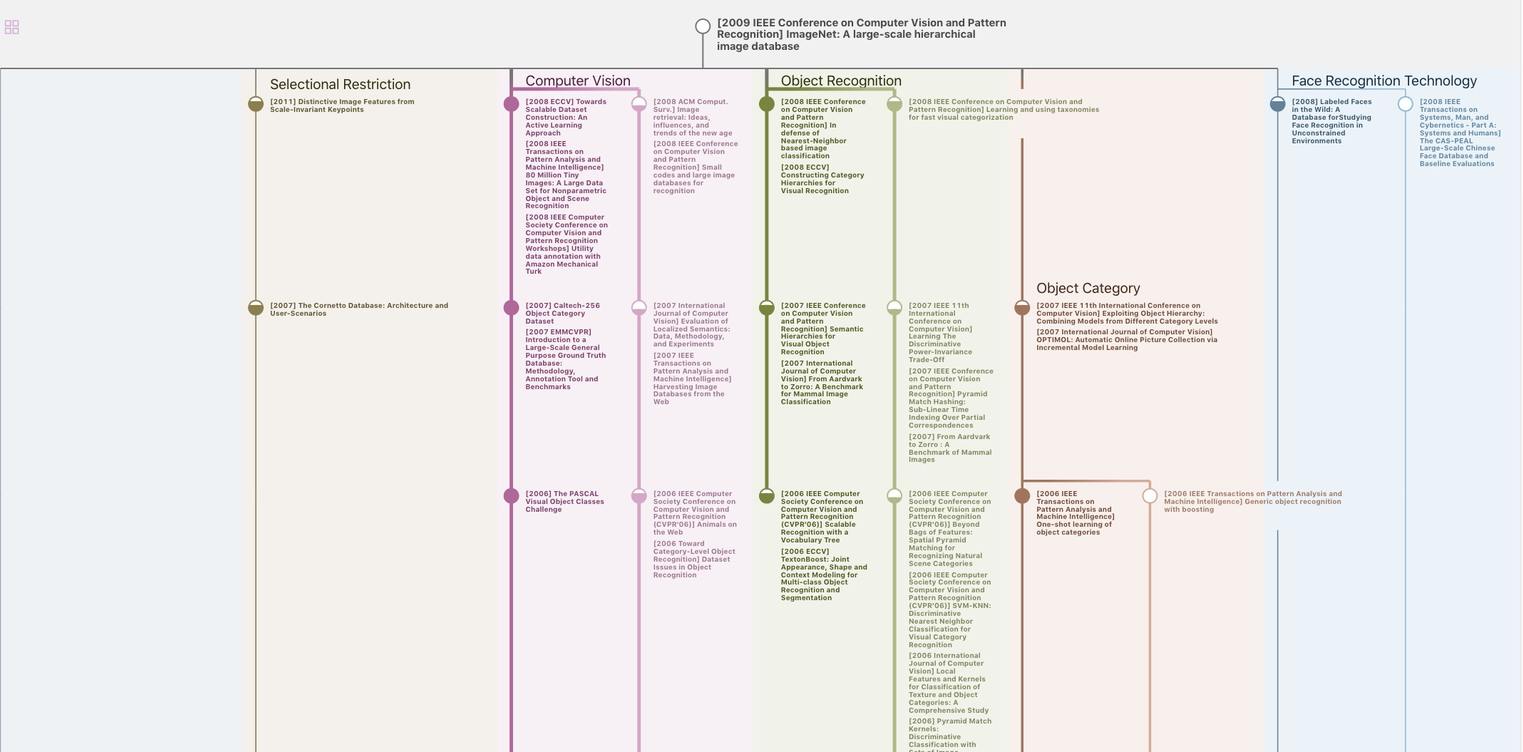
生成溯源树,研究论文发展脉络
Chat Paper
正在生成论文摘要