Clustering with Semidefinite Programming and Fixed Point Iteration
arxiv(2022)
摘要
We introduce a novel method for clustering using a semidefinite programming (SDP) relaxation of the Max k-Cut problem. The approach is based on a new methodology for rounding the solution of an SDP relaxation using iterated linear optimization. We show the vertices of the Max k-Cut relaxation correspond to partitions of the data into at most k sets. We also show the vertices are attractive fixed points of iterated linear optimization. Each step of this iterative process solves a relaxation of the closest vertex problem and leads to a new clustering problem where the underlying clusters are more clearly defined. Our experiments show that using fixed point iteration for rounding the Max k-Cut SDP relaxation leads to significantly better results when compared to randomized rounding.
更多查看译文
关键词
semidefinite programming,clustering,fixed point iteration
AI 理解论文
溯源树
样例
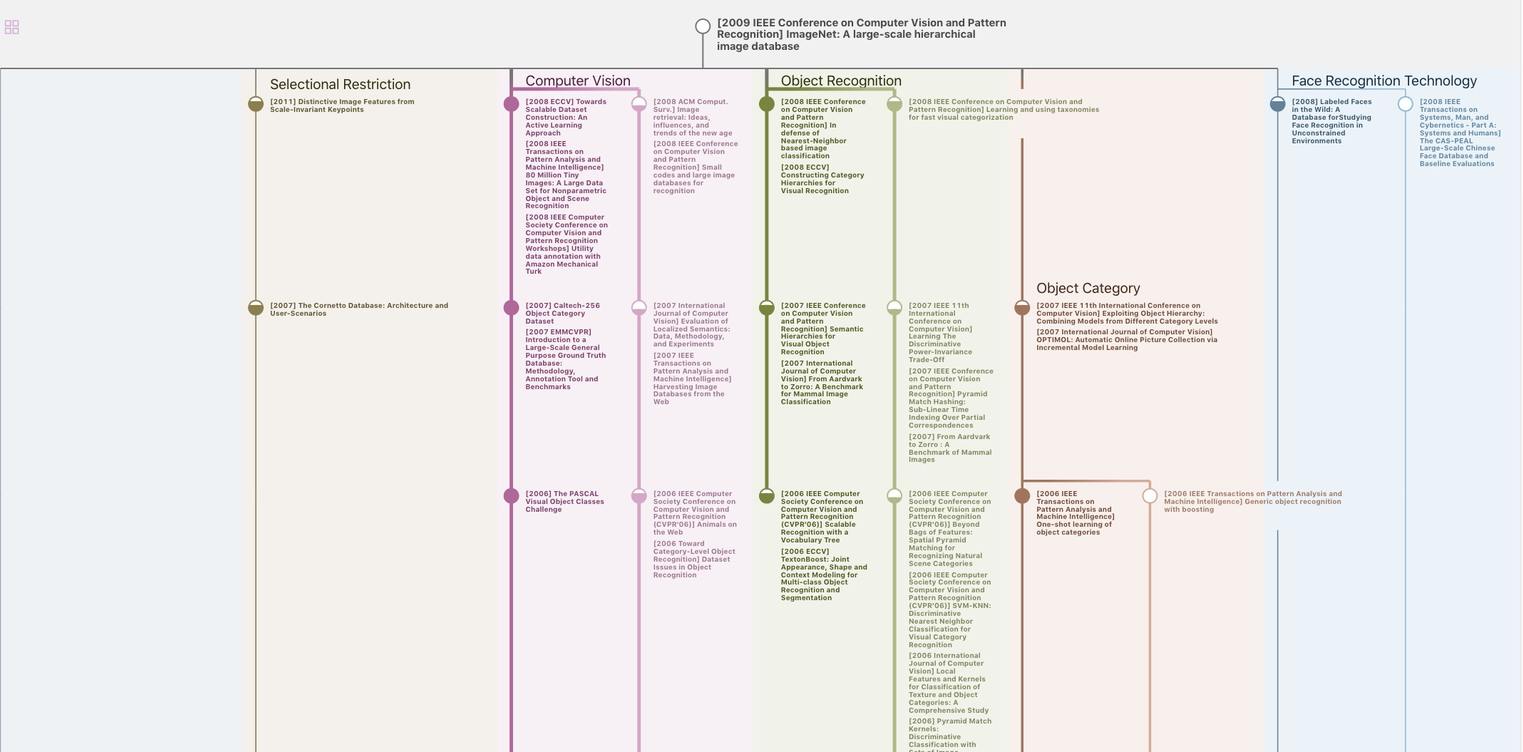
生成溯源树,研究论文发展脉络
Chat Paper
正在生成论文摘要