Jet tagging in the Lund plane with graph networks
JOURNAL OF HIGH ENERGY PHYSICS(2021)
摘要
bstract The identification of boosted heavy particles such as top quarks or vector bosons is one of the key problems arising in experimental studies at the Large Hadron Collider. In this article, we introduce LundNet, a novel jet tagging method which relies on graph neural networks and an efficient description of the radiation patterns within a jet to optimally disentangle signatures of boosted objects from background events. We apply this framework to a number of different benchmarks, showing significantly improved performance for top tagging compared to existing state-of-the-art algorithms. We study the robustness of the LundNet taggers to non-perturbative and detector effects, and show how kinematic cuts in the Lund plane can mitigate overfitting of the neural network to model-dependent contributions. Finally, we consider the computational complexity of this method and its scaling as a function of kinematic Lund plane cuts, showing an order of magnitude improvement in speed over previous graph-based taggers.
更多查看译文
关键词
Jets, Phenomenological Models
AI 理解论文
溯源树
样例
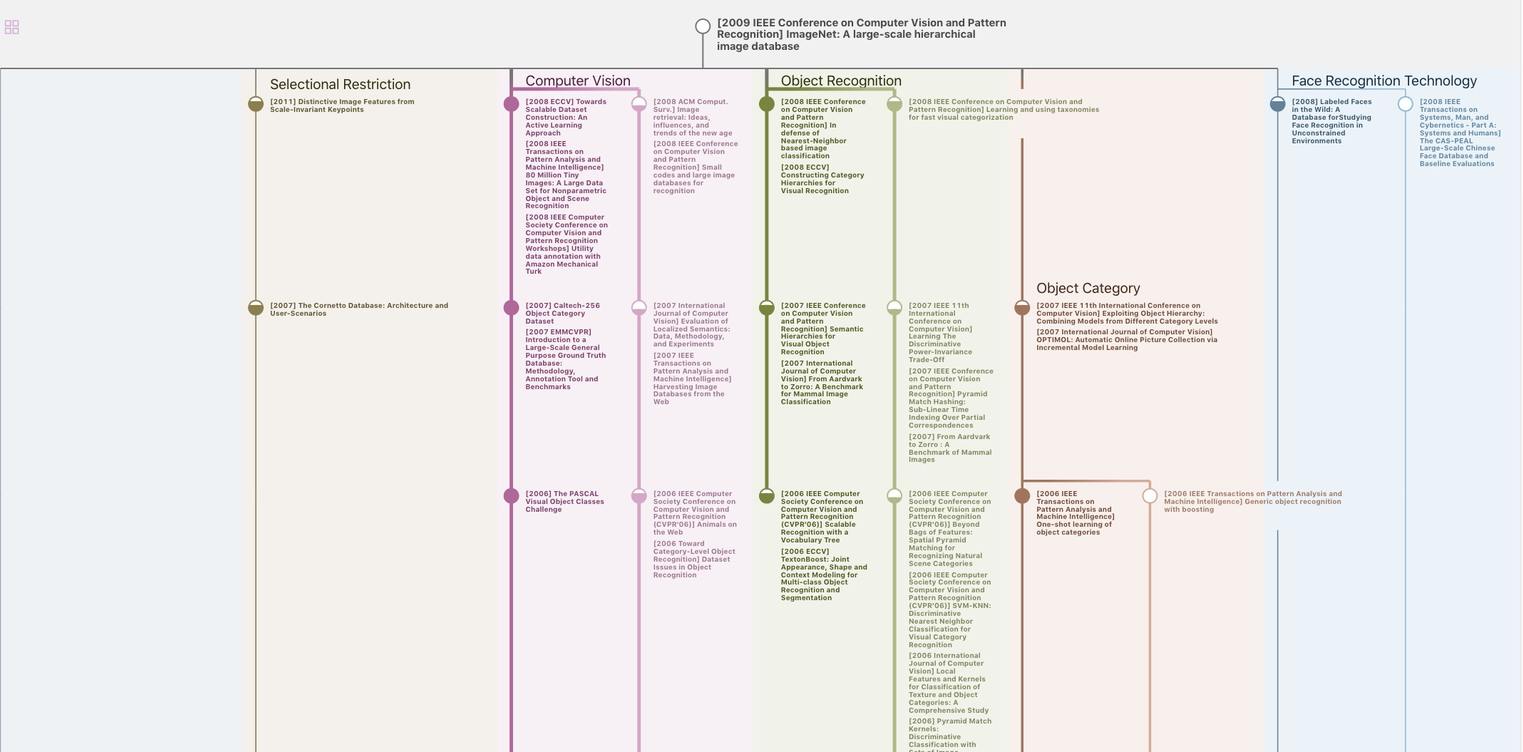
生成溯源树,研究论文发展脉络
Chat Paper
正在生成论文摘要