Unsupervised Domain Adaptation From Synthetic To Real Images For Anchorless Object Detection
VISAPP: PROCEEDINGS OF THE 16TH INTERNATIONAL JOINT CONFERENCE ON COMPUTER VISION, IMAGING AND COMPUTER GRAPHICS THEORY AND APPLICATIONS - VOL. 5: VISAPP(2021)
摘要
Synthetic images are one of the most promising solutions to avoid high costs associated with generating annotated datasets to train supervised convolutional neural networks (CNN). However, to allow networks to generalize knowledge from synthetic to real images, domain adaptation methods are necessary. This paper implements unsupervised domain adaptation (UDA) methods on an anchorless object detector. Given their good performance, anchorless detectors are increasingly attracting attention in the field of object detection. While their results are comparable to the well-established anchor-based methods, anchorless detectors are considerably faster. In our work, we use CenterNet, one of the most recent anchorless architectures, for a domain adaptation problem involving synthetic images. Taking advantage of the architecture of anchorless detectors, we propose to adjust two UDA methods, viz., entropy minimization and maximum squares loss, originally developed for segmentation, to object detection. Our results show that the proposed UDA methods can increase the mAP from 61% to 69% with respect to direct transfer on the considered anchorless detector. The code is available: https://github.com/scheckmedia/centernet-uda.
更多查看译文
关键词
Unsupervised Domain Adaptation, Synthetic Images, CenterNet, Anchorless/Keypoint-based Detectors, Object Detection
AI 理解论文
溯源树
样例
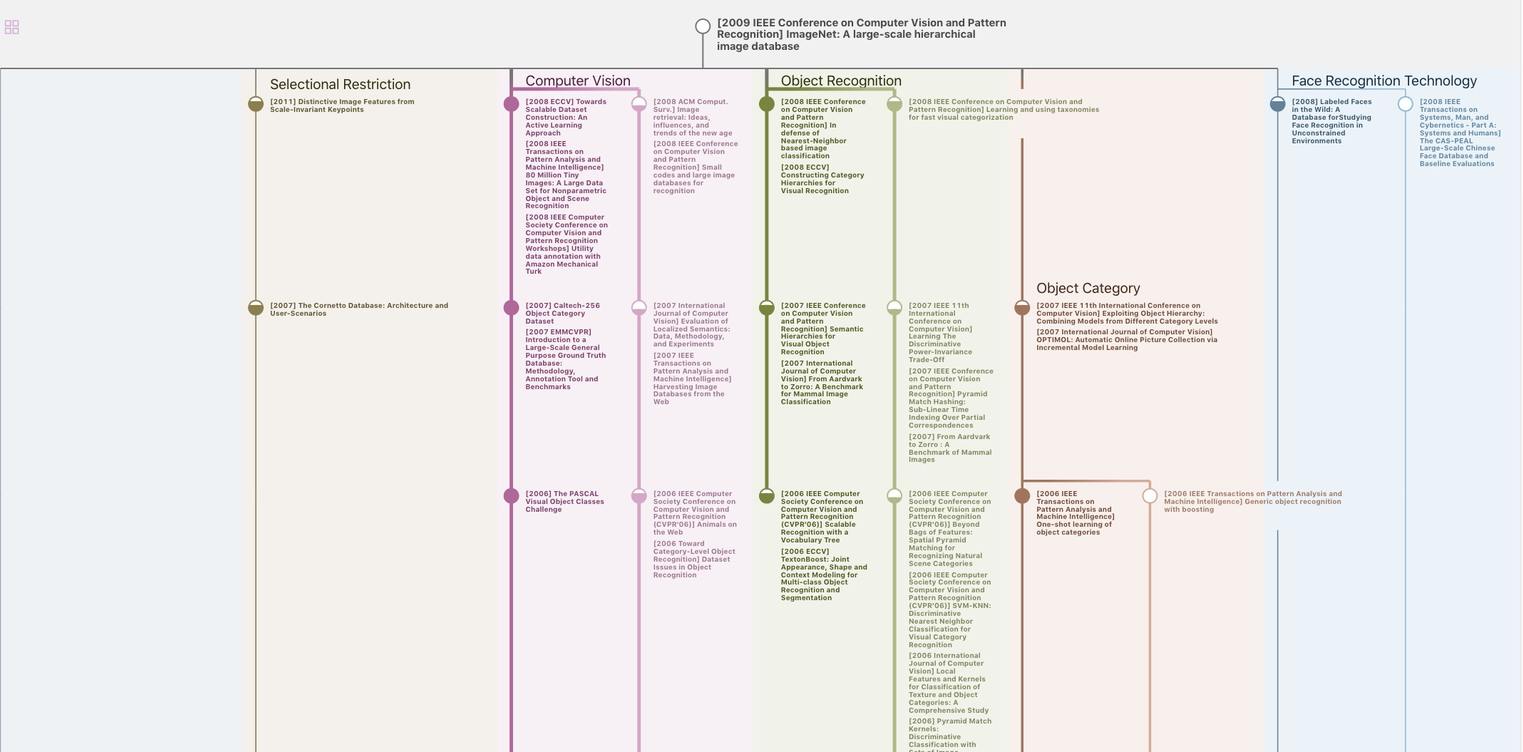
生成溯源树,研究论文发展脉络
Chat Paper
正在生成论文摘要