Fully Automatic Atrial Fibrosis Assessment Using A Multilabel Convolutional Neural Network
CIRCULATION-CARDIOVASCULAR IMAGING(2020)
摘要
Background:Pathological atrial fibrosis is a major contributor to sustained atrial fibrillation. Currently, late gadolinium enhancement (LGE) scans provide the only noninvasive estimate of atrial fibrosis. However, widespread adoption of atrial LGE has been hindered partly by nonstandardized image processing techniques, which can be operator and algorithm dependent. Minimal validation and limited access to transparent software platforms have also exacerbated the problem. This study aims to estimate atrial fibrosis from cardiac magnetic resonance scans using a reproducible operator-independent fully automatic open-source end-to-end pipeline.Methods:A multilabel convolutional neural network was designed to accurately delineate atrial structures including the blood pool, pulmonary veins, and mitral valve. The output from the network removed the operator dependent steps in a reproducible pipeline and allowed for automated estimation of atrial fibrosis from LGE-cardiac magnetic resonance scans. The pipeline results were compared against manual fibrosis burdens, calculated using published thresholds: image intensity ratio 0.97, image intensity ratio 1.61, and mean blood pool signal +3.3 SD.Results:We validated our methods on a large 3-dimensional LGE-cardiac magnetic resonance data set from 207 labeled scans. Automatic atrial segmentation achieved a 91% Dice score, compared with the mutual agreement of 85% in Dice seen in the interobserver analysis of operators. Intraclass correlation coefficients of the automatic pipeline with manually generated results were excellent and better than or equal to interobserver correlations for all 3 thresholds: 0.94 versus 0.88, 0.99 versus 0.99, 0.99 versus 0.96 for image intensity ratio 0.97, image intensity ratio 1.61, and +3.3 SD thresholds, respectively. Automatic analysis required 3 minutes per case on a standard workstation. The network and the analysis software are publicly available.Conclusions:Our pipeline provides a fully automatic estimation of fibrosis burden from LGE-cardiac magnetic resonance scans that is comparable to manual analysis. This removes one key source of variability in the measurement of atrial fibrosis.
更多查看译文
关键词
atrial fibrillation, deep learning, fibrosis, magnetic resonance imaging
AI 理解论文
溯源树
样例
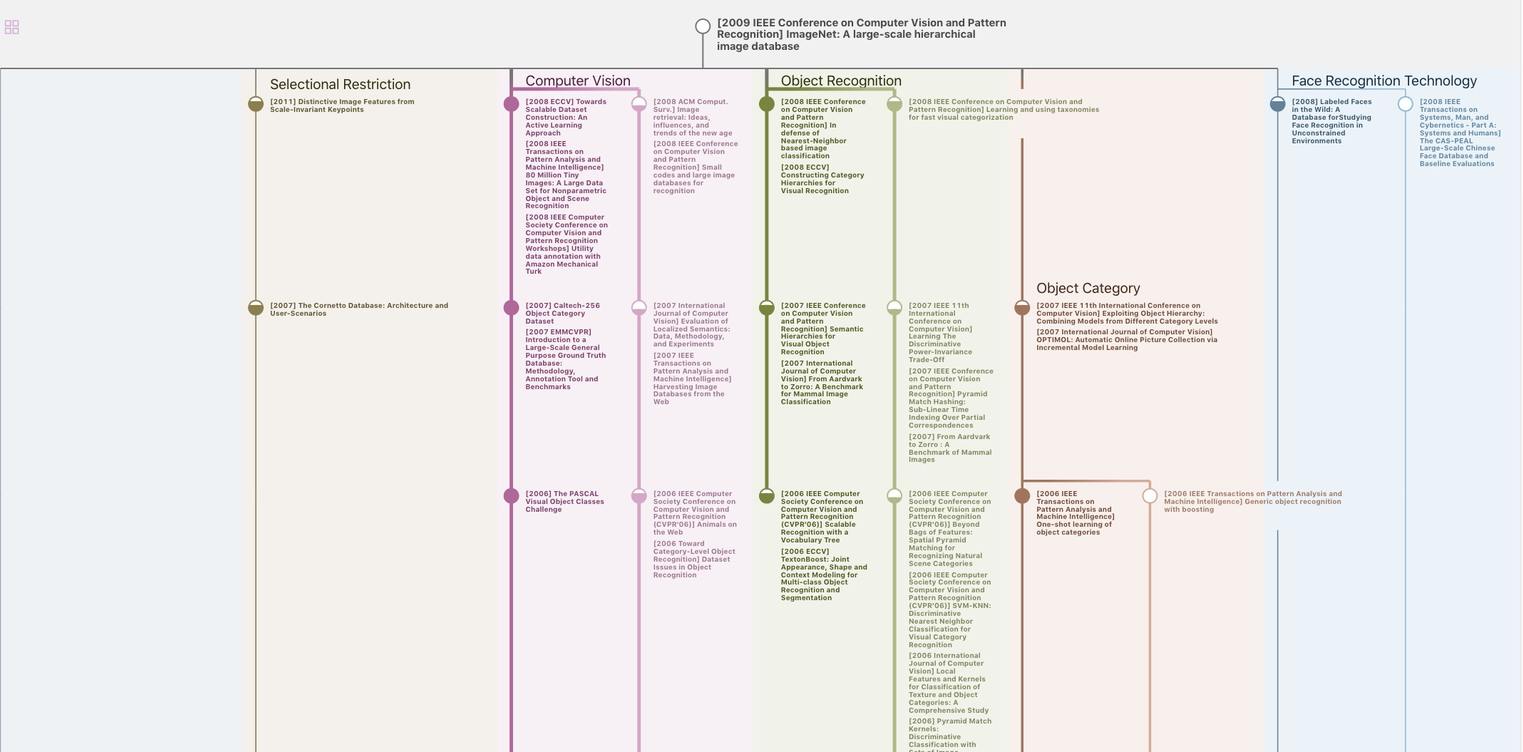
生成溯源树,研究论文发展脉络
Chat Paper
正在生成论文摘要