LEARN++: Recurrent Dual-Domain Reconstruction Network for Compressed Sensing CT
IEEE Transactions on Radiation and Plasma Medical Sciences(2023)
摘要
Compressed sensing (CS) computed tomography (CT) has been proven to be important for several clinical applications, such as sparse-view CT, digital tomosynthesis, and interior tomography. Traditional CS focuses on the design of handcrafted prior regularizers, which are usually image-dependent and time-consuming. Inspired by recently proposed deep learning-based CT reconstruction models, we extend the state-of-the-art LEARN model to a dual-domain version, dubbed LEARN++. Different from existing iteration unrolling methods, which only involve projection data in the data consistency layer, the proposed LEARN++ model integrates two parallel and interactive subnetworks to perform image restoration and sinogram inpainting operations on both the image and projection domains simultaneously, which can fully explore the latent relations between projection data and reconstructed images. The experimental results demonstrate that the proposed LEARN++ model achieves competitive qualitative and quantitative results compared to several state-of-the-art methods in terms of both artifact reduction and detail preservation.
更多查看译文
关键词
Compressed sensing (CS),computed tomography (CT),deep learning,sparse coding
AI 理解论文
溯源树
样例
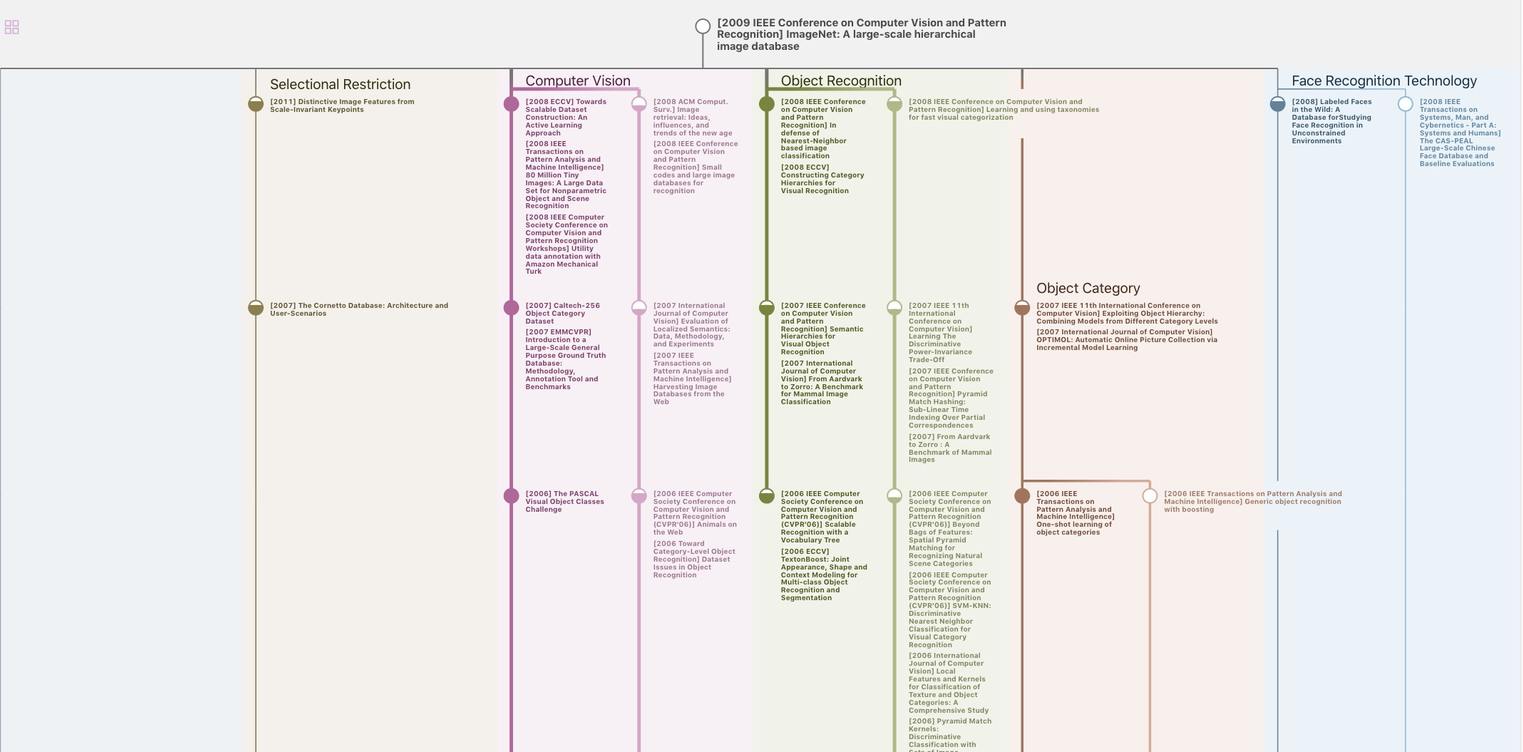
生成溯源树,研究论文发展脉络
Chat Paper
正在生成论文摘要