A New Feature Extraction And Recognition Method For Microexpression Based On Local Non-Negative Matrix Factorization
FRONTIERS IN NEUROROBOTICS(2020)
摘要
Microexpression is usually characterized by short duration and small action range, and the existing general expression recognition algorithms do not work well for microexpression. As a feature extraction method, non-negative matrix factorization can decompose the original data into different components, which has been successfully applied to facial recognition. In this paper, local non-negative matrix factorization is explored to decompose microexpression into some facial muscle actions, and extract features for recognition based on apex frame. However, the existing microexpression datasets fall short of samples to train a classifier with good generalization. The macro-to-micro algorithm based on singular value decomposition can augment the number of microexpressions, but it cannot meet non-negative properties of feature vectors. To address these problems, we propose an improved macro-to-micro algorithm to augment microexpression samples by manipulating the macroexpression data based on local non-negative matrix factorization. Finally, several experiments are conducted to verify the effectiveness of the proposed scheme, which results show that it has a higher recognition accuracy for microexpression compared with the related algorithms based on CK+/CASME2/SAMM datasets.
更多查看译文
关键词
macro-expression, micro-expression, macro-to-micro transformation, feature extraction, non-negative matrix factorization, CK plus, CASME2, SAMM datasets
AI 理解论文
溯源树
样例
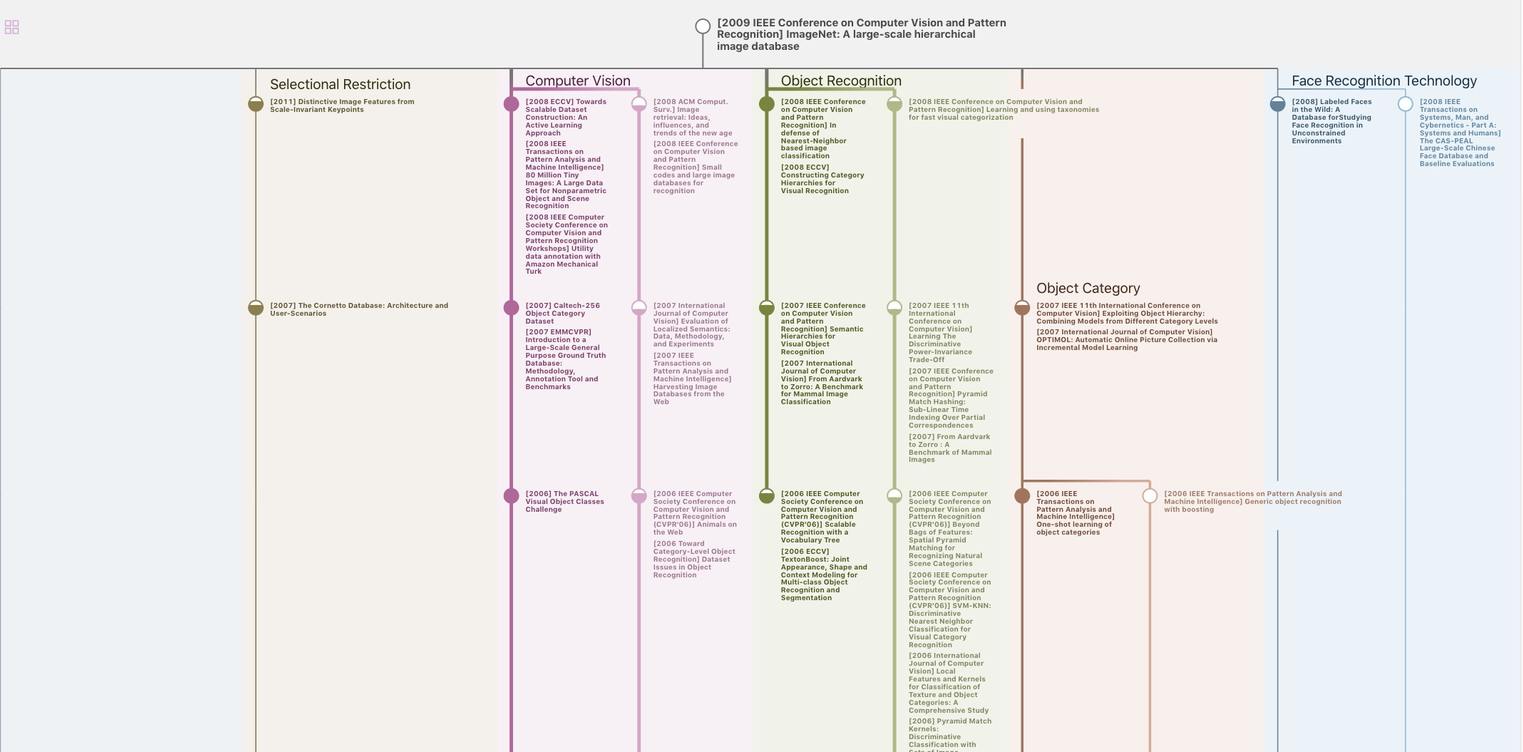
生成溯源树,研究论文发展脉络
Chat Paper
正在生成论文摘要