An Unsupervised Reconstruction Method for Low-Dose CT Using Deep Generative Regularization Prior
Biomedical signal processing and control(2022)
摘要
Low-dose CT imaging requires reconstruction from noisy indirect measurements which can be defined as an illposed linear inverse problem. In addition to conventional FBP method in CT imaging, recent compressed sensing based methods exploit handcrafted priors which are mostly simplistic and hard to determine. More recently, deep learning (DL) based methods have become popular in medical imaging field. In CT imaging, DL based methods try to learn a function that maps low-dose images to normal-dose images. Although the results of these methods are promising, their success mostly depends on the availability of high-quality massive datasets. In this study, we proposed a method that does not require any training data or a learning process. Our method exploits such an approach that deep convolutional neural networks (CNNs) generate patterns easier than the noise, therefore randomly initialized generative neural networks can be suitable priors to be used in regularizing the reconstruction. In the experiments, the proposed method is implemented with different loss function variants. Both analytical CT phantoms and real-world CT images are used with different views. Conventional FBP method, a popular iterative method (SART), and TV regularized SART are used in the comparisons. We demonstrated that our method with different loss function variants outperforms the other methods both qualitatively and quantitatively.
更多查看译文
关键词
Unsupervised reconstruction,Low-dose CT,Deep generative regularization,Deep image prior
AI 理解论文
溯源树
样例
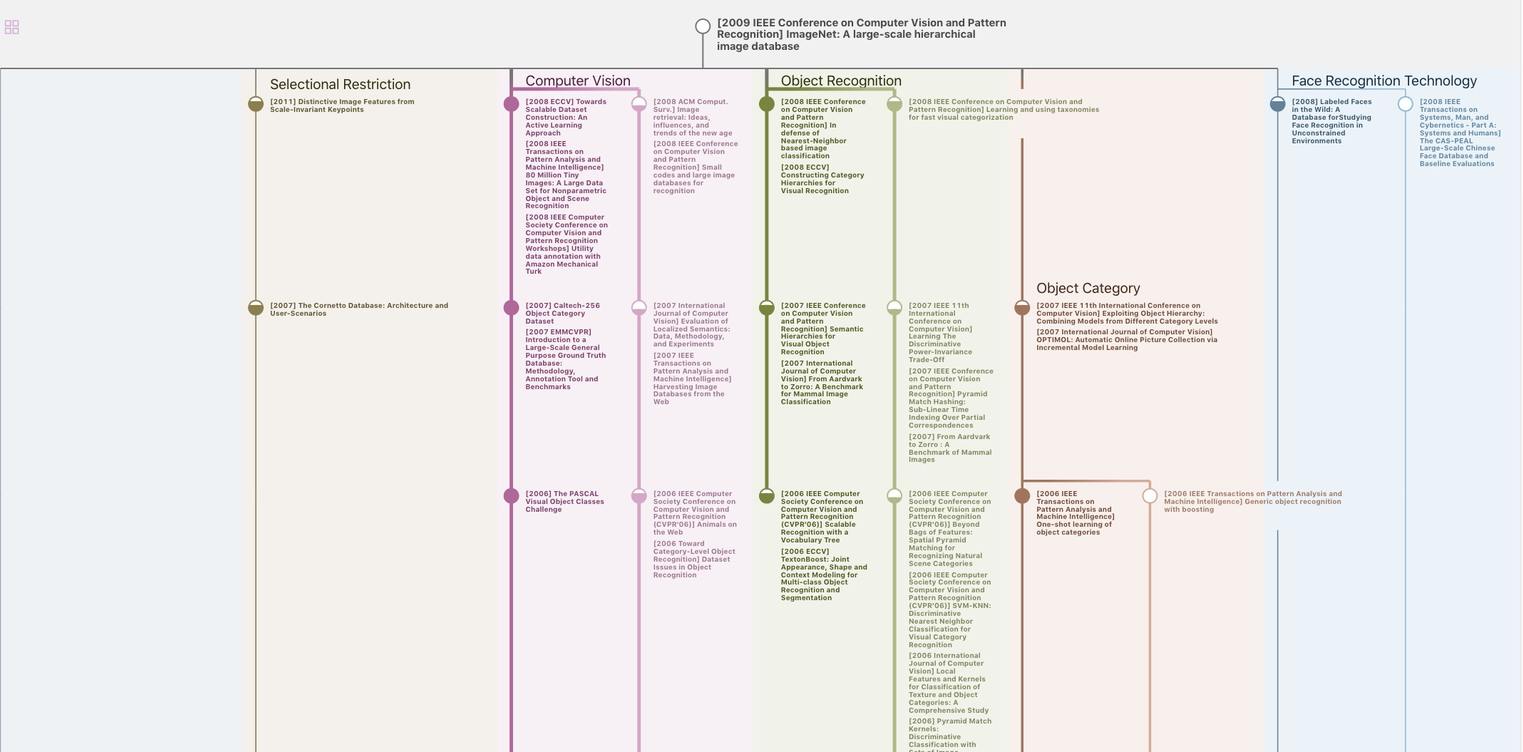
生成溯源树,研究论文发展脉络
Chat Paper
正在生成论文摘要