Parameter estimation for complex thermal-fluid flows using approximate Bayesian computation
PHYSICAL REVIEW FLUIDS(2018)
摘要
Approximate Bayesian computation (ABC) is a data-driven technique that uses many low-cost numerical simulations to estimate unknown physical or model parameters (e.g., boundary conditions and material properties), as well as their uncertainties, given reference data from real-world experiments or higher-fidelity numerical simulations. In this study, ABC is used to estimate unknown parameters in complex thermal-fluid flows, and the technique is demonstrated on a periodically forced high-temperature jet and a steadily forced helium-air plume. In the first case, computational reference data are used to assess the accuracy of ABC when estimating the frequency, amplitude, and mean of the periodic velocity forcing at the jet inlet. These tests show that ABC provides accurate and reasonably certain estimates of inflow parameters even when the model simulations imperfectly represent the physics underlying the reference data. These tests also show that measurements far from the inlet can be used to perform the estimation, and that temperature measurements can be used to infer velocity inflow parameters. For the second case, ABC is used to estimate the inlet Richardson number and its uncertainty given experimental measurements of the Strouhal number within the plume. Once again, the approach is able to accurately estimate unknown parameters with relatively low uncertainty. As a result, ABC is shown to be a versatile technique for estimating unknown physical parameters when knowledge of a real-world system is limited or incomplete.
更多查看译文
AI 理解论文
溯源树
样例
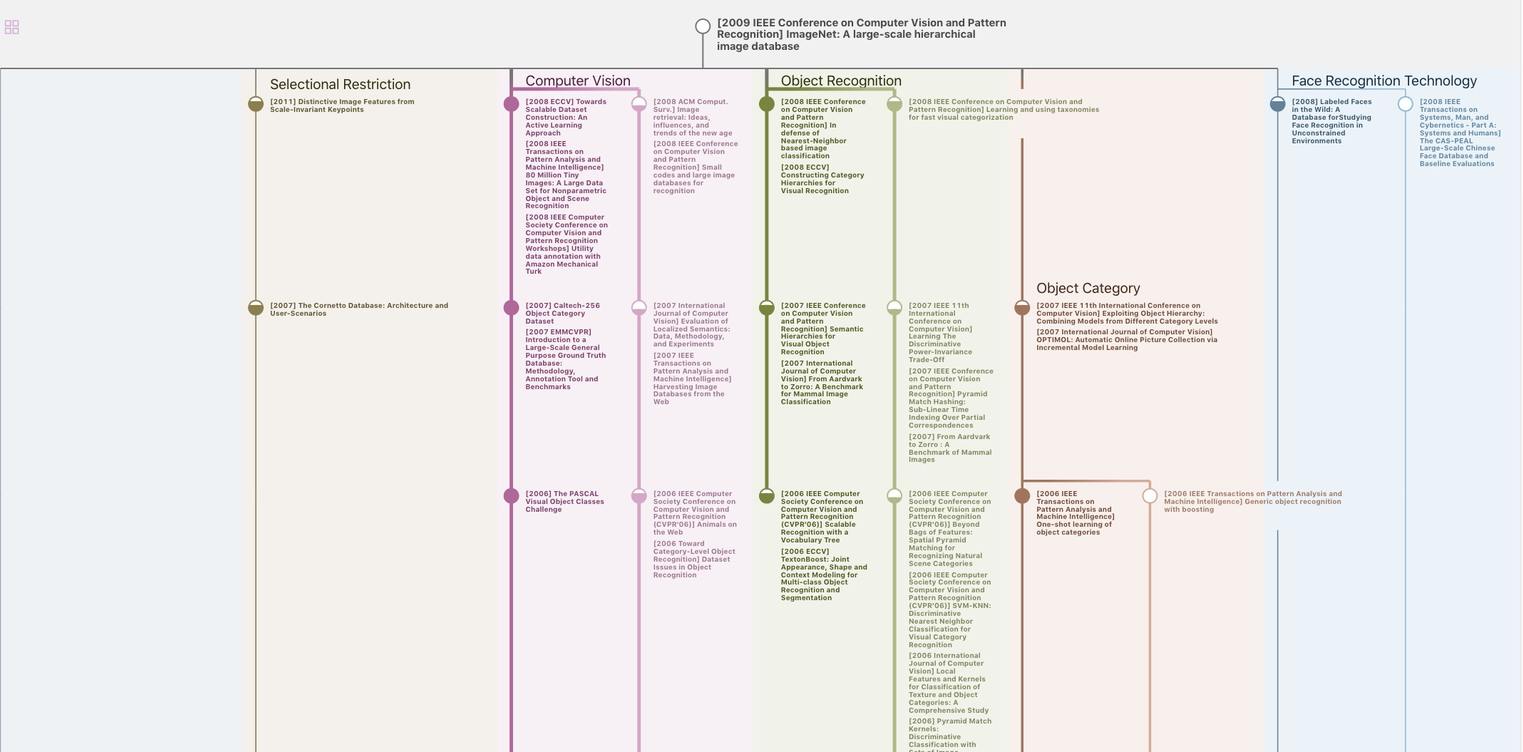
生成溯源树,研究论文发展脉络
Chat Paper
正在生成论文摘要