Algorithmically-consistent deep learning frameworks for structural topology optimization
ENGINEERING APPLICATIONS OF ARTIFICIAL INTELLIGENCE(2021)
摘要
Topology optimization has emerged as a popular approach to refine a component's design and increase its performance. However, current state-of-the-art topology optimization frameworks are compute-intensive, mainly due to multiple finite element analysis iterations required to evaluate the component's performance during the optimization process. Recently, machine learning (ML)-based topology optimization methods have been explored by researchers to alleviate this issue. However, previous ML approaches have mainly been demonstrated on simple two-dimensional applications with low-resolution geometry. Further, current methods are based on a single ML model for end-to-end prediction, which requires a large dataset for training. These challenges make it non-trivial to extend current approaches to higher resolutions. In this paper, we develop deep learning-based frameworks consistent with traditional topology optimization algorithms for 3D topology optimization with a reasonably fine (high) resolution. We achieve this by training multiple networks, each learning a different step of the overall topology optimization methodology, making the framework more consistent with the topology optimization algorithm. We demonstrate the application of our framework on both 2D and 3D geometries. The results show that our approach predicts the final optimized design better (5.76x reduction in total compliance MSE in 2D; 2.03x reduction in total compliance MSE in 3D) than current ML-based topology optimization methods.
更多查看译文
关键词
Topology optimization,Deep learning,Sequence models,Algorithmically-consistent learning
AI 理解论文
溯源树
样例
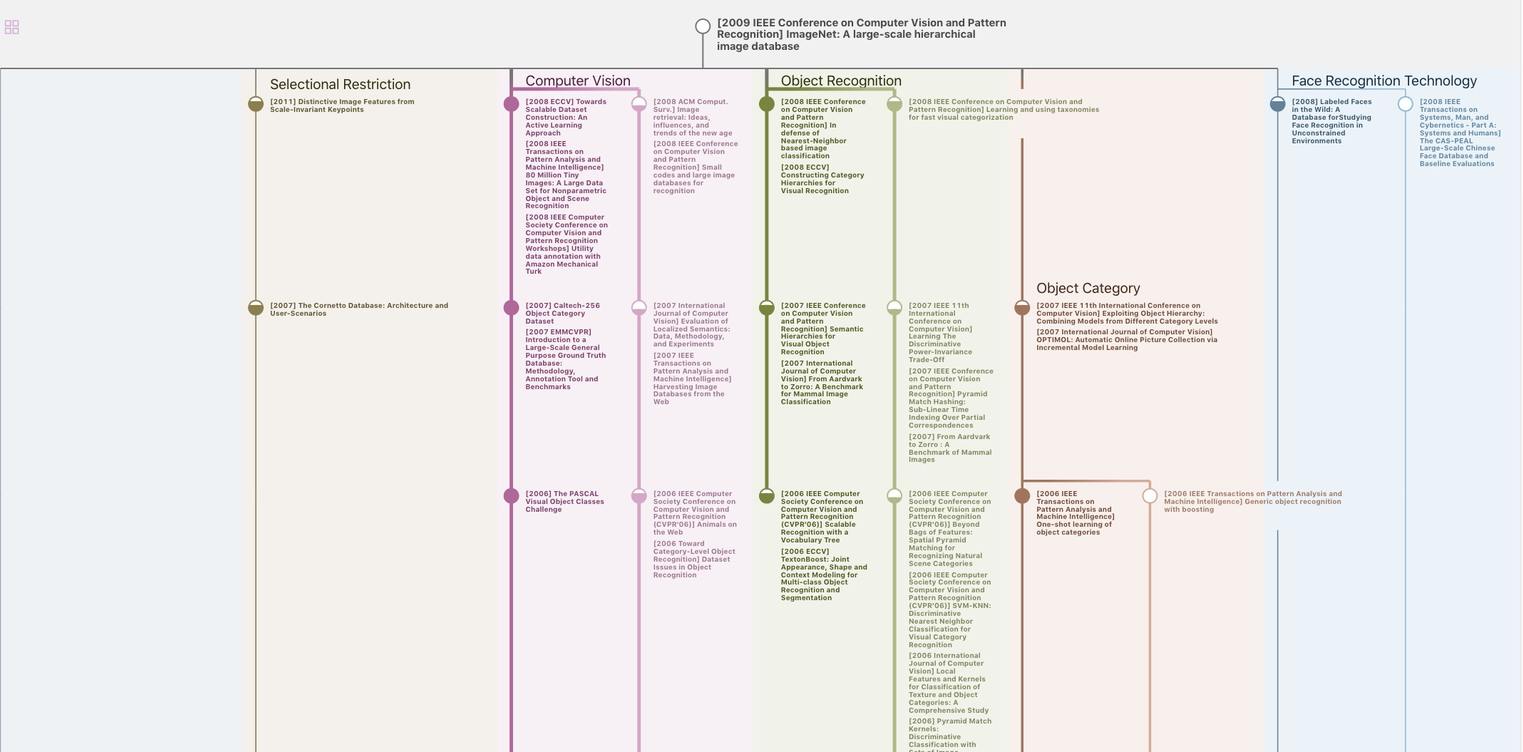
生成溯源树,研究论文发展脉络
Chat Paper
正在生成论文摘要