Systematic comparison of epidemic growth patterns using two different estimation approaches
Infectious Disease Modelling(2021)
摘要
Background: Different estimation approaches are frequently used to calibrate mathematical models to epidemiological data, particularly for analyzing infectious disease outbreaks. Here, we use two common methods to estimate parameters that characterize growth patterns using the generalized growth model (GGM) calibrated to real outbreak datasets.Materials and methods: Data from 31 outbreaks are used to fit the GGM to the ascending phase of each outbreak and estimate the parameters using both least squares (LSQ) and maximum likelihood estimation (MLE) methods. We utilize parametric bootstrapping to construct confidence intervals for parameter estimates. We compare the results including RMSE, Anscombe residual, and 95% prediction interval coverage. We also evaluate the correlation between the estimates from both methods.Results: Comparing LSQ and MLE estimates, most outbreaks have similar parameter estimates, RMSE, Anscombe, and 95% prediction interval coverage. Parameter estimates do not differ across methods when the model yields a good fit to the early growth phase. However, for two outbreaks, there are systematic deviations in model fit to the data that explain differences in parameter estimates (e.g., residuals represent random error rather than systematic deviation).Conclusion: Our findings indicate that utilizing LSQ and MLE methods produce similar results in the context of characterizing epidemic growth patterns with the GGM, provided that the model yields a good fit to the data. (c) 2020 The Authors. Production and hosting by Elsevier B.V. on behalf of KeAi Communications Co., Ltd. This is an open access article under the CC BY-NC-ND license (http://creativecommons.org/licenses/by-nc-nd/4.0/).
更多查看译文
关键词
Parameter estimation,Generalized growth model,Least squares estimation,Maximum likelihood estimation,Epidemiological models
AI 理解论文
溯源树
样例
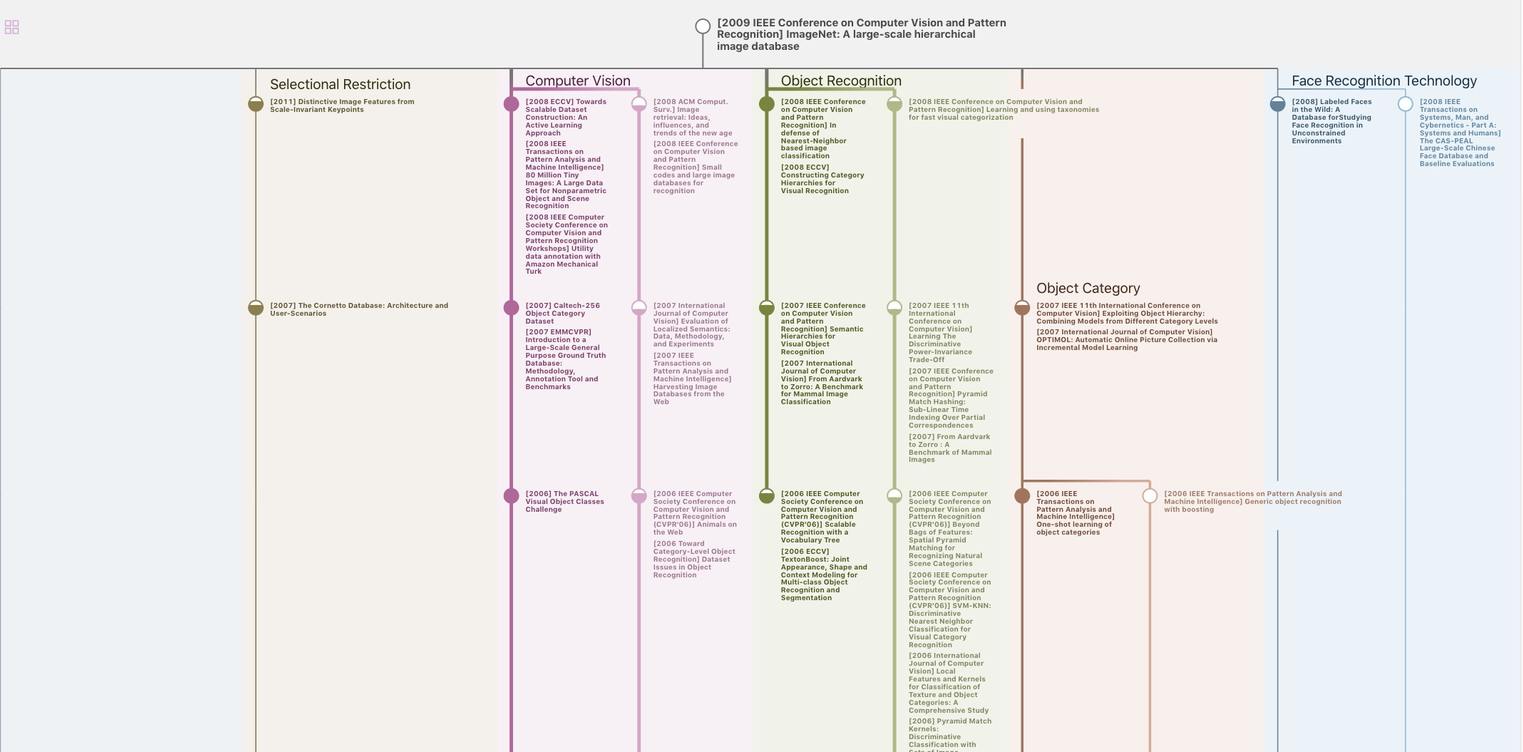
生成溯源树,研究论文发展脉络
Chat Paper
正在生成论文摘要