TADO: Time-varying Attention with Dual-Optimizer Model
20TH IEEE INTERNATIONAL CONFERENCE ON DATA MINING (ICDM 2020)(2020)
摘要
The review-based recommender systems are commonly utilized to measure users preferences towards different items. In this paper, we focus on addressing three main problems existing in the review-based methods. Firstly, these methods suffer from the class-imbalanced problem where rating levels with lower proportions will be ignored to some extent. Thus, their performance on relatively rare rating levels is unsatisfactory. As the first attempt in this field to address this problem, we propose a flexible dual-optimizer model to gain robustness from both regression loss and classification loss. Secondly, to address the problem caused by the insufficient contextual information extraction ability of word embedding, we first introduce BERT into the review-based method to improve the performance of the semantic analysis. Thirdly, the existing methods ignore the feature information of the time-varying user preferences. Therefore, we propose a time-varying feature extraction module with bidirectional long short-term memory and multi-scale convolutional neural network. Afterward, an interaction component is proposed to further summarize the contextual information of the user-item pairs. To verify the effectiveness of the proposed TADO, we conduct extensive experiments on 23 benchmark datasets selected from Amazon Product Reviews. Compared with several recently proposed state-of-the-art methods, our model obtains significant gain over ALFM, MPCN, and ANR averagely with 20.98\%, 9.84\%, and 15.46\%, respectively. Further analysis proves the necessity of jointly using the proposed components in TADO.
更多查看译文
关键词
natural language processing, deep learning, review-based recommender system, collaborative filtering
AI 理解论文
溯源树
样例
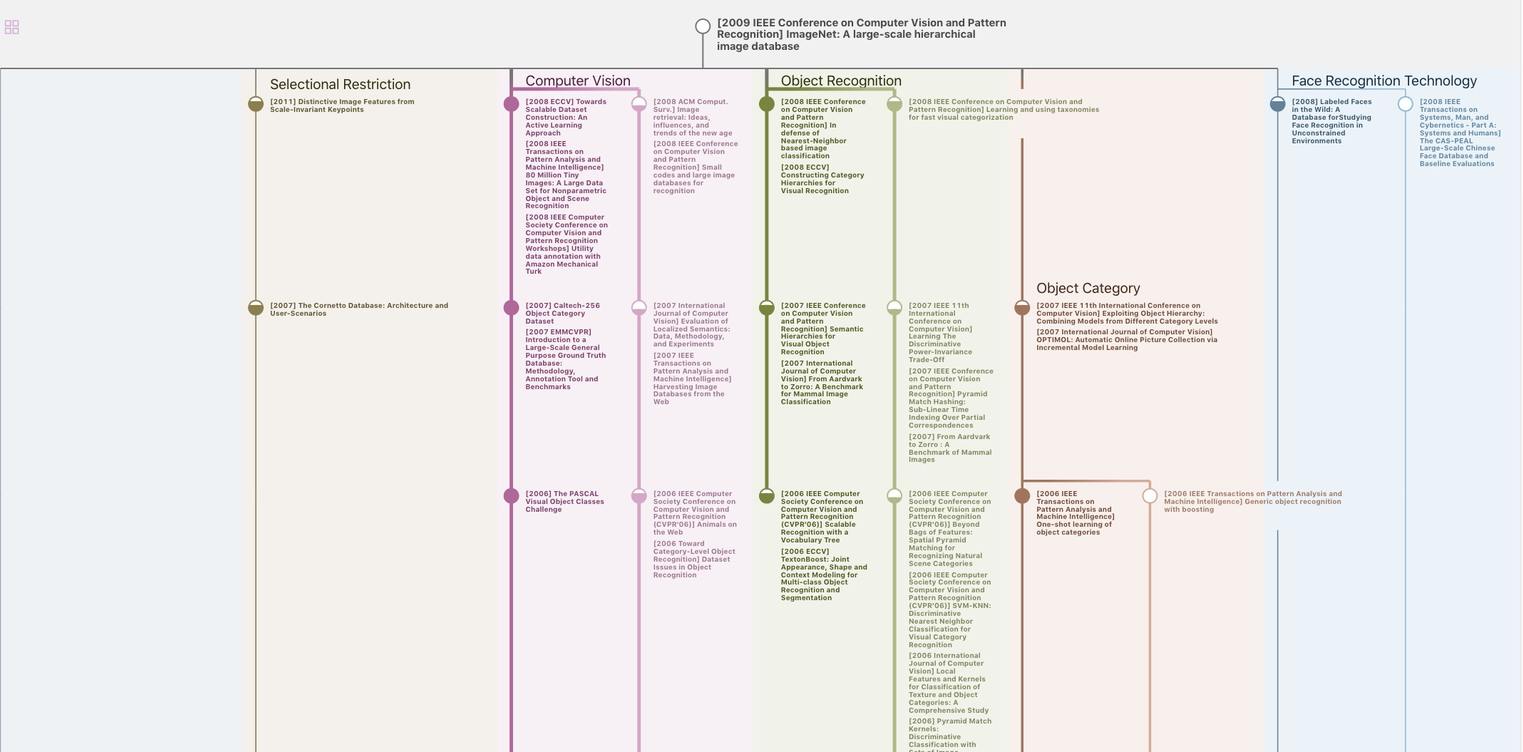
生成溯源树,研究论文发展脉络
Chat Paper
正在生成论文摘要