A Novel Hybrid Approach for Partial Discharge Signal Detection Based on Complete Ensemble Empirical Mode Decomposition with Adaptive Noise and Approximate Entropy
Entropy (Basel, Switzerland)(2020)
Abstract
To eliminate the influence of white noise in partial discharge (PD) detection, we propose a novel method based on complete ensemble empirical mode decomposition with adaptive noise (CEEMDAN) and approximate entropy (ApEn). By introducing adaptive noise into the decomposition process, CEEMDAN can effectively separate the original signal into different intrinsic mode functions (IMFs) with distinctive frequency scales. Afterward, the approximate entropy value of each IMF is calculated to eliminate noisy IMFs. Then, correlation coefficient analysis is employed to select useful IMFs that represent dominant PD features. Finally, real IMFs are extracted for PD signal reconstruction. On the basis of EEMD, CEEMDAN can further improve reconstruction accuracy and reduce iteration numbers to solve mode mixing problems. The results on both simulated and on-site PD signals show that the proposed method can be effectively employed for noise suppression and successfully extract PD pulses. The fusion algorithm combines the CEEMDAN algorithm and the ApEn algorithm with their respective advantages and has a better de-noising effect than EMD and EEMD.
MoreTranslated text
Key words
partial discharge,de-noising,complete ensemble empirical mode decomposition,approximate entropy,correlation coefficient analysis
AI Read Science
Must-Reading Tree
Example
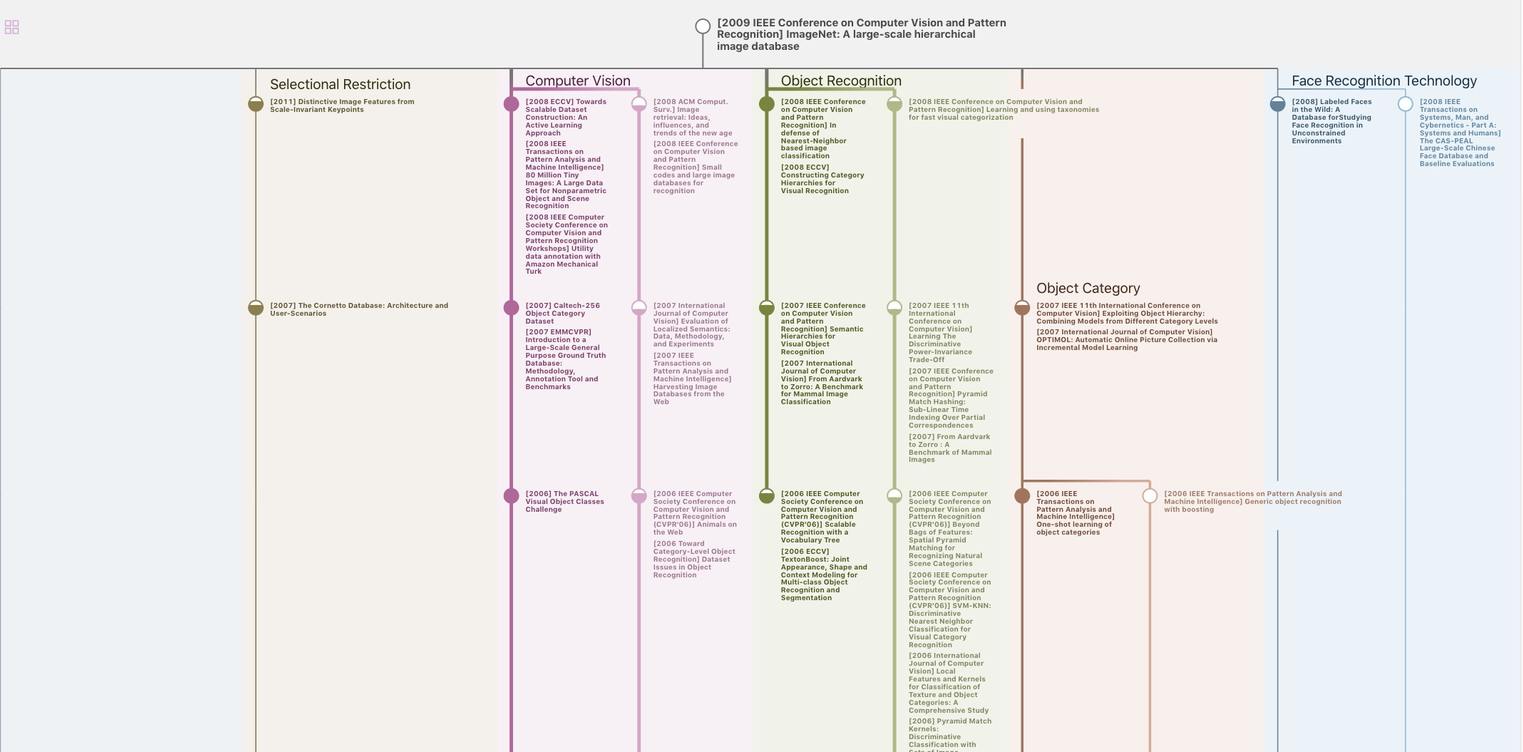
Generate MRT to find the research sequence of this paper
Chat Paper
Summary is being generated by the instructions you defined